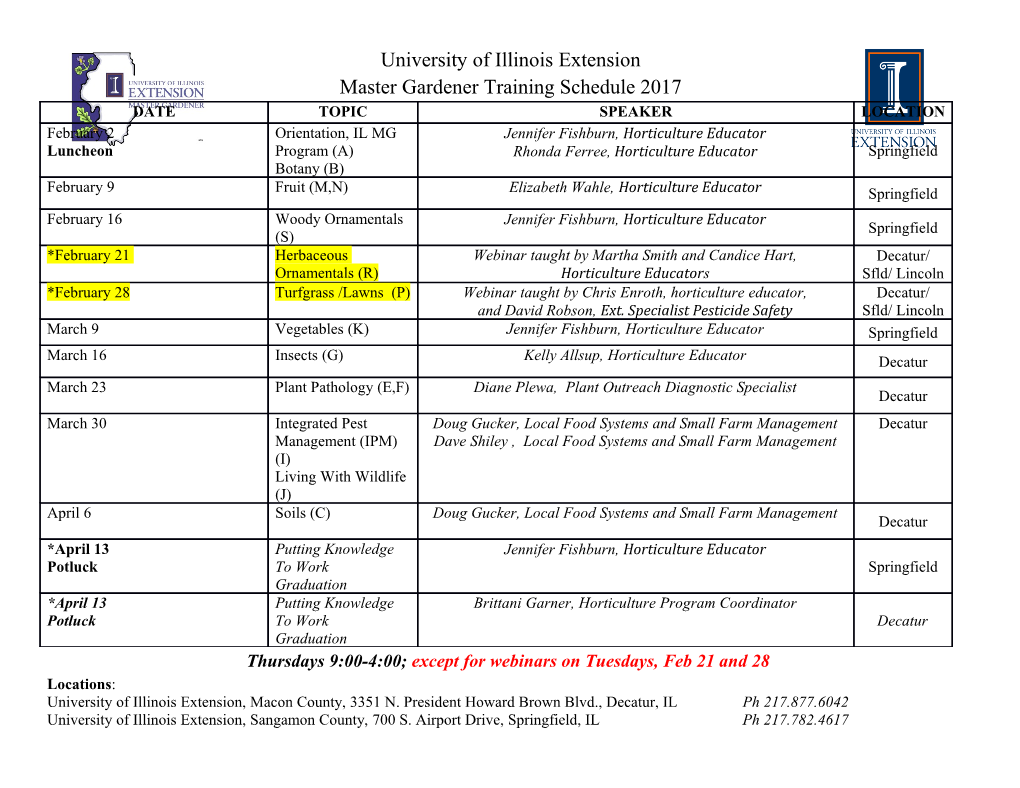
Factors influencing estimates of the climate sensitivity parameter from a zero-dimensional climate model Angus J. Ferraro University of Reading, UK Submitted in partial fulfillment of the requirements of the degree of Bachelor of Science, Meteorology at the University of Reading. 12/03/2010 ABSTRACT Climate sensitivity is defined and its role as a key parameter governing the climate system is explained. Estimates of climate sensitivity from the literature are compared and their methods are examined. The 5-95% range used by the Intergovernmental Panel on Climate Change is 0.3 to 2.4 K(Wm-2)-1. Using a simple zero-dimensional climate model it is shown that the constraint on climate sensitivity varies dramatically between two configurations of the model, with one indicating that very high sensitivities (> 2 K(Wm-2)-1) are unlikely. The change in constraint is found to be due to the change in radiative forcing data between the two configurations of the model, specifically the biomass burning aerosol forcing and the forcing from the indirect effect of aerosol on cloud albedo. The methods by which forcing data sets are obtained are critically examined. The uncertainty on biomass burning aerosol forcing, and consequently whether this change in constraint is significant, is discussed. The change in the biomass burning aerosol forcing is shown to be due to observational evidence showing that the forcing is in fact positive when the aerosol overlies cloud. The time evolution of the forcing is generally scaled to gross tropical deforestation and this assumption is discussed. Suggestions are made for improving the estimate of the climate sensitivity parameter by obtaining more accurate forcing data. 1 INTRODUCTION 1. Introduction Changing climate has the potential to be a great challenge to human development over the coming decades. Responses will depend on climate predictions produced by complex models. However these models contain uncertainties both in initial conditions and in parameterising processes on a spatial scale smaller than their grid size. One of the main uncertainties in climate prediction is the climate system's response to radiative forcing, termed `climate sensitivity'. Radiative forcing is defined as a net change in the Earth's radiation balance per square metre, usually at the tropopause. If climate sensitivity is high, a relatively small change in Earth's radiation balance could produce a very significant warming effect with severe consequences for both human society and planetary ecology. There are two main parameters used to quantify the climate sensitivity. The `climate sensitivity parameter' is the most basic measure, defined as the equilibrium annual global- mean temperature response to a unit change in radiative forcing. It is usually denoted λ and has units of K(Wm-2)-1. The equilibrium global-mean temperature response ∆T to a forcing ∆F is therefore: ∆T = λ∆F (1) Some, including the Intergovernmental Panel on Climate Change (IPCC), prefer to use `equilibrium climate sensitivity' (ECS), which is the equilibrium annual global mean temper- ature response to a forcing equivalent to a doubling of atmospheric CO2 from pre-industrial levels (the atmospheric concentration in 1750). The ECS therefore has units of K and is sometimes written as ∆T 2x. The ECS and the climate sensitivity parameter are essentially the same parameter, but expressed in different units. Since the radiative forcing associated -2 with a doubling of CO2 concentration is approximately 3.7 Wm , the conversion is simply: ∆T 2x = 3:7λ. It is also sometimes useful to define a `transient climate response' (TCR), which is the annual global mean temperature change at the time of CO2 doubling in a climate simulation with a 1% per year compounded increase in CO2 concentration. With units of K, it is a measure of the magnitude and also the speed of the response to a forcing. This depends greatly on the rate at which the ocean takes up heat. The TCR is outside the scope of this investigation, and here the climate sensitivity parameter λ will be used as the sole measure of climate sensitivity. It is simple to calculate the climate sensitivity parameter for a black body Earth with no atmosphere by differentiating the Stefan-Boltzmann law and assuming a mean Earth surface 1 1 INTRODUCTION temperature, giving λ=0.3 K(Wm-2)-1 (using a surface temperature of 288 K). However, the situation is complicated, not only because the Earth is not a black body (if this were the only problem it could be solved easily by including albedo and emissivity in our calculations) but because the atmosphere, biosphere, cryosphere, hydrosphere and lithosphere all contribute to the climate system internally via feedbacks. For example, warming is associated with melt- ing ice which decreases the Earth's albedo and consequently reinforces the warming. The main climate feedback mechanisms are described in Soden and Held (2006). See Wallace and Hobbs (2006) Ch. 10.3 for a mathematical description of climate sensitivity involving feedbacks. Another well-understood feedback is the water vapour content of the atmosphere. Warming increases the atmosphere's vapour capacity, and water vapour is radiatively warm- ing. However, many feedbacks are not well understood. These include changes in the ability of the oceans and other carbon sinks to absorb CO2, and how clouds respond to changing climate. Our incomplete understanding of these feedbacks makes climate sensitivity hard to quantify, so estimates still have a large degree of uncertainty. Roe and Baker (2007) provide more detail on why climate sensitivity is so unpredictable. They note that the probability density function (PDF) of the climate sensitivity parameter is skewed such that high values are unlikely but not impossible. They show that this is a mathematical result which emerges due to the definition of the climate sensitivity as λ = ∆T0=(1 − f) where ∆T0 is the initial blackbody temperature response to a forcing and f is the feedback factor (which takes a value between 0 and 1, with f = 0representing no feedbacks). The relationship between f and λ is therefore nonlinear,and a Gaussian distribution in f must lead to a skewed distribution in λ. In addition, different forcing mechanisms might have a different sensitivity. This is termed their ‘efficacy’. These effects are poorly understood and it is generally assumed (as it will be here) that the sensitivity is constant for all forcing mechanisms. Hansen et al. (2005) find that efficacy varies between approximately 50-150% of the climate sensitivity parameter for various forcing mechanisms. Another important parameter in climate models is the rate of diffusion of heat into the deep ocean from the mixed layer. This parameterises the heat transport from the mixed to the deep layer of the ocean in the real climate system. The ocean diffusivity, κ, has units of m2s-1. This quantity is similarly uncertain and is equally worthy of investigation, being especially relevant to the TCR, but is outside the scope of this investigation. 2 a Methods for estimating climate sensitivity 1 INTRODUCTION a. Methods for estimating climate sensitivity Ideally the sensitivity of the climate system could be derived directly from observations of radiative forcing and global-mean temperature. There are several reasons why this is not possible. There is uncertainty over the temperature change which can be attributed to a single forcing due to uncertainty over the precise nature of feedbacks, uncertainty over temperature observations themselves and much greater uncertainty in forcing. The feedbacks are dependent on the climate state, which means the sensitivity is also a function of the state of the system (global and regional temperatures). This makes calculations very complex. Knutti and Hegerl (2008) summarise the methods of estimating climate sensitivity and their limitations as well as detailing the essentials of climate sensitivity theory, and giving an overview of current best estimates. They conclude that a climate sensitivity parameter of 0.4 - 1.2 K(Wm-2)-1 produces simulations which perform well in replicating instrumental data. One approach to estimating the climate sensitivity is to use climate models to vary the parameters influencing λ, and then attach probabilities to the different λ values based on how closely the resulting model simulation matches up to observations. In simpler models the value of λ can be varied explicitly, while in complex GCMs it is an emergent property derived from a number of other parameters. Estimates for λ from IPCC (2007) take the form of PDFs with a 5 to 95% range of 0.3 to 2.4 K(Wm-2)-1. The estimates from studies contributing to the IPCC Fourth Assessment Report (AR4) are shown in Figure 1. Recall that the IPCC define sensitivity as ∆T 2x = 3:7λ, the ECS. The lower bound is relatively well constrained but there is significant uncertainty on the upper bound. There are a number of reasons for this asymmetrical nature of the climate sensitivity PDF. It is largely caused by uncertainties and nonlinearities in the response to forcings. In addition, a high sensitivity is difficult to rule out because of the uncertainty over aerosol forcings (see Figure 2). It is possible that a large aerosol forcing could all but cancel out the greenhouse gas forcing over the 20th Century. This would result in a very small net forcing which must be responsible for the recent increase in global-mean temperatures as detailed in Kennedy et al. (2009). Varying parameters and comparing model simulations to observations is the most com- mon method for estimating climate sensitivity. The advantage of the method is its theoretical simplicity - it simply finds the combination of model parameters which produces the best model fit to observations. One obvious limitation is that of the imperfect models - no model 3 a Methods for estimating climate sensitivity 1 INTRODUCTION Fig.
Details
-
File Typepdf
-
Upload Time-
-
Content LanguagesEnglish
-
Upload UserAnonymous/Not logged-in
-
File Pages39 Page
-
File Size-