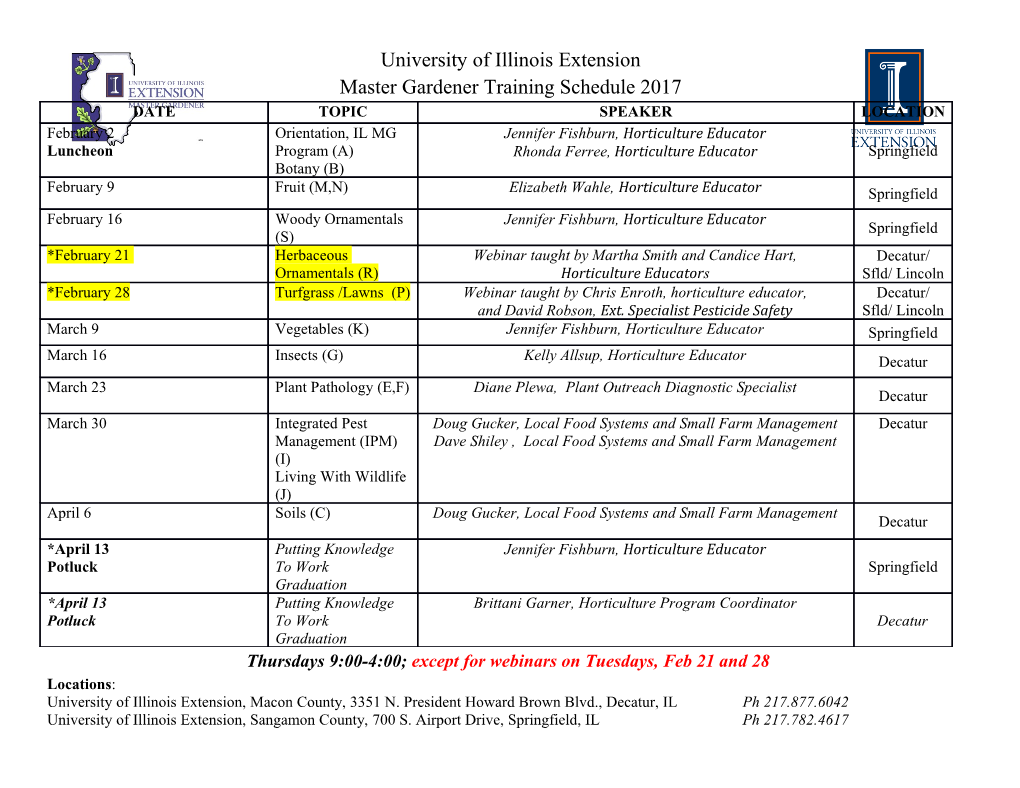
University of Kentucky UKnowledge Theses and Dissertations--Computer Science Computer Science 2018 RETAIL DATA ANALYTICS USING GRAPH DATABASE Rashmi Priya University of Kentucky, [email protected] Digital Object Identifier: https://doi.org/10.13023/ETD.2018.221 Right click to open a feedback form in a new tab to let us know how this document benefits ou.y Recommended Citation Priya, Rashmi, "RETAIL DATA ANALYTICS USING GRAPH DATABASE" (2018). Theses and Dissertations-- Computer Science. 67. https://uknowledge.uky.edu/cs_etds/67 This Master's Thesis is brought to you for free and open access by the Computer Science at UKnowledge. It has been accepted for inclusion in Theses and Dissertations--Computer Science by an authorized administrator of UKnowledge. For more information, please contact [email protected]. STUDENT AGREEMENT: I represent that my thesis or dissertation and abstract are my original work. Proper attribution has been given to all outside sources. I understand that I am solely responsible for obtaining any needed copyright permissions. I have obtained needed written permission statement(s) from the owner(s) of each third-party copyrighted matter to be included in my work, allowing electronic distribution (if such use is not permitted by the fair use doctrine) which will be submitted to UKnowledge as Additional File. I hereby grant to The University of Kentucky and its agents the irrevocable, non-exclusive, and royalty-free license to archive and make accessible my work in whole or in part in all forms of media, now or hereafter known. I agree that the document mentioned above may be made available immediately for worldwide access unless an embargo applies. I retain all other ownership rights to the copyright of my work. I also retain the right to use in future works (such as articles or books) all or part of my work. I understand that I am free to register the copyright to my work. REVIEW, APPROVAL AND ACCEPTANCE The document mentioned above has been reviewed and accepted by the student’s advisor, on behalf of the advisory committee, and by the Director of Graduate Studies (DGS), on behalf of the program; we verify that this is the final, approved version of the student’s thesis including all changes required by the advisory committee. The undersigned agree to abide by the statements above. Rashmi Priya, Student Dr. Victor Marek, Major Professor Dr. Miroslaw Truszczynski, Director of Graduate Studies RETAIL DATA ANALYTICS USING GRAPH DATABASE THESIS A thesis submitted in partial fulfillment of the requirements for the degree of Master of Science in the College of Engineering at the University of Kentucky By Rashmi Priya Lexington, Kentucky Director : Dr. Victor Marek, Professor of Department of Computer Science Lexington, Kentucky 2018 Copyright c Rashmi Priya 2018 ABSTRACT OF THESIS RETAIL DATA ANALYTICS USING GRAPH DATABASE Big data is an area focused on storing, processing and visualizing huge amount of data. Today data is growing faster than ever before. We need to find the right tools and applications and build an environment that can help us to obtain valuable insights from the data. Retail is one of the domains that collects huge amount of transaction data everyday. Retailers need to understand their customer’s purchasing pattern and behavior in order to take better business decisions. Market basket analysis is a field in data mining, that is focused on discovering pat- terns in retail’s transaction data. Our goal is to find tools and applications that can be used by retailers to quickly understand their data and take better business decisions. Due to the amount and complexity of data, it is not possible to do such activities manually. We witness that trends change very quickly and retailers want to be quick in adapting the change and taking actions. This needs automation of processes and using algorithms that are efficient and fast. In our work, we mine transaction data by modeling the data as graphs. We use clustering algorithms to discover communities (clusters) in the data and then use the clusters for building a recommendation system that can recommend products to customers based on their buying behavior. KEYWORDS: Retail Data Analytics, Clustering, Graph Database, Neo4j, Louvain Al- gorithm, Recommendation System Author’s signature: Rashmi Priya Date: May 30, 2018 RETAIL DATA ANALYTICS USING GRAPH DATABASE By Rashmi Priya Director of Thesis: Dr. Victor Marek Director of Graduate Studies: Dr. Miroslaw Truszczynski Date: May 30, 2018 Dedicated to my father who is not anymore with us but has taught his numerous students, my sister and me to always be thirsty for knowledge and to work hard. Acknowledgements My sincerest gratitude to my advisor, Dr. Victor Marek , for his insights and guidance throughout the thesis. He was always happy to meet me and discuss our work. He encouraged and motivated me throughout the process. I would also like to thank all the professors and especially Dr. Mirek Truszczyn- ski, Director of Graduate Studies, for guiding me throughout my master’s study. Finally, I am thankful to my family and friends who have always supported me, in particular my mother, my sister and my life partner. iii Contents Acknowledgements iii Contents iv List of Figures vi List of Tables vii 1 Introduction 1 2 Background 3 2.1 Big Data and Data Analytics......................... 3 2.2 Relational and Non-Relational Databases.................. 5 2.2.1 Relational database........................... 5 2.2.2 Non-Relational Database....................... 7 2.3 Relevant concepts of Graph Theory..................... 10 2.3.1 Centrality................................ 10 2.3.2 Graph Clustering............................ 12 2.3.3 Basic Clustering Methods....................... 13 2.3.4 Evaluation of Clustering........................ 15 2.3.5 Clustering Algorithms......................... 16 2.3.6 Modularity:............................... 17 2.3.7 Girvan-Newman Algorithm...................... 18 2.3.8 Louvain Algorithm........................... 19 2.3.9 Limitations of a Modularity Maximization Technique....... 20 3 Methodology 22 3.1 Data Preparation in Neo4j........................... 24 3.2 Algorithm for Clustering............................ 26 3.3 Evaluating Clustering Algorithm....................... 27 3.4 Recommendation systems........................... 29 4 Processing the Instacart Retail Data 31 4.1 Data Set..................................... 31 4.2 Preprocessing Data............................... 32 4.3 Data Representation.............................. 32 4.4 Analyzing the Data............................... 34 iv 4.5 Product-Product Network........................... 38 4.6 Community Detection............................. 39 4.7 Web Application For Recommendation System............... 47 5 Performance of Neo4j 58 6 Conclusions and Future Work 61 6.1 Conclusions................................... 61 6.2 Future Work................................... 62 References 63 Vita 68 v List of Figures 2.1 Example of data implemented by means of Relational Model...... 6 2.2 Examples of NoSQL databases........................ 8 2.3 Graph Data Model in Neo4j.......................... 9 3.1 Example of data represented in Neo4j.................... 25 3.2 Connected Caveman Graph of 6 cliques................... 28 4.1 Procedure for creating relationship between Orders to Products..... 33 4.2 Procedure for Creation of Nodes in Neo4j.................. 33 4.3 Data Model Loaded in Neo4j graph database................ 34 4.4 Number of orders placed on each day of the week............. 35 4.5 Number of orders placed at different hours of the day.......... 36 4.6 Number of orders placed at different hours of the day on various days of the week.................................... 36 4.7 Top 10 Most Ordered Products........................ 37 4.8 Top 10 Departments.............................. 37 4.9 Top 10 Aisles................................... 37 4.10 Procedure for creating the product-product network........... 38 4.11 Relationships among Customers, Orders and Products.......... 39 4.12 Some of the communities obtained from first model............ 41 4.13 List of Products in cluster 5 formed on the subgraph of the Product Network..................................... 44 4.14 List of Products in cluster 7 formed on the subgraph of the Product Network..................................... 44 4.15 Communities in "Babies" department using Gephi............. 45 4.16 View Data Page from the application.................... 48 4.17 Departments Page from the application................... 49 4.18 Community Detection Home Page from the application......... 50 4.19 Screen shot of Recommendation Based On Sub-Graph of the Product Network..................................... 52 4.20 Products in a Community Page from the application........... 53 4.21 Example of Product Recommendation For Customer "Id10" in Model 2 54 4.22 Recommending Products within Departments Page from the application 56 4.23 Recommendation based on Departments.................. 57 vi List of Tables 2.1 Girvan-Newman Algorithm [17]....................... 19 2.2 Louvain community detection Algorithm.................. 21 3.1 Communities detected in unweighted Caveman Graphs of different sizes 28 3.2 Communities detected in weighted Caveman Graphs of different sizes. 29 4.1 Dataset used in the Project........................... 35 4.2 Number of Nodes and Edges in Product Network............. 39 4.3 Weight Distribution in the Product Network...............
Details
-
File Typepdf
-
Upload Time-
-
Content LanguagesEnglish
-
Upload UserAnonymous/Not logged-in
-
File Pages79 Page
-
File Size-