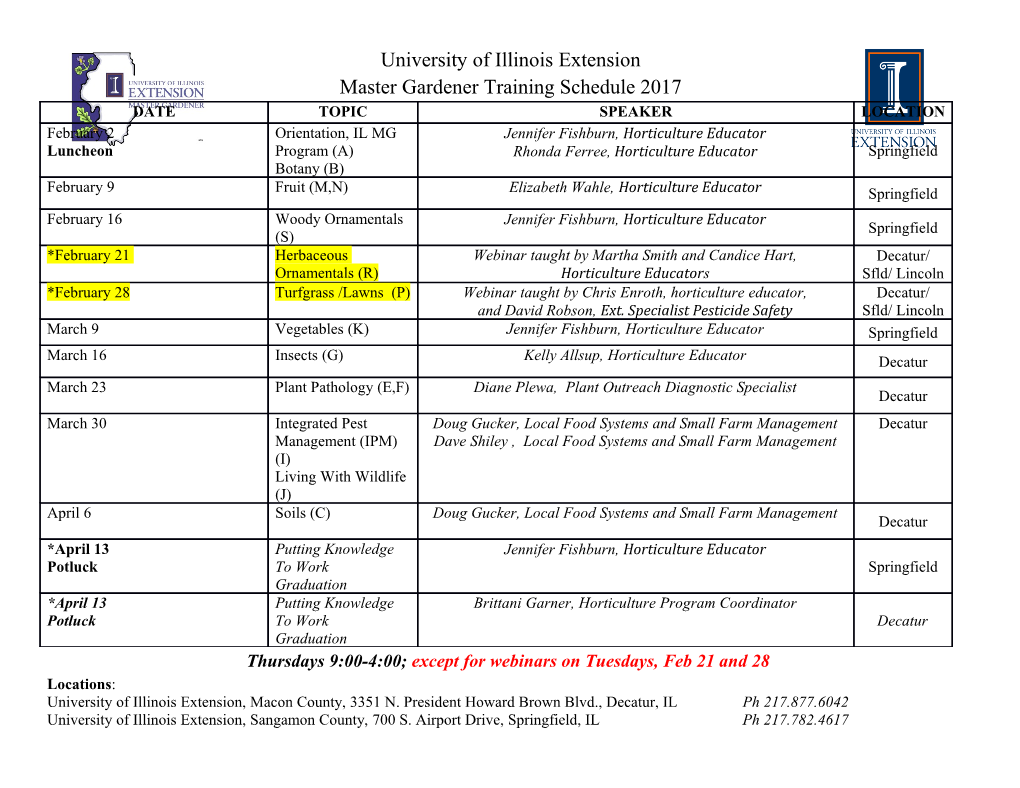
Proceedings of the Twenty-Fifth International Joint Conference on Artificial Intelligence (IJCAI-16) Personalizing EEG-Based Affective Models with Transfer Learning 1 1,2,3, Wei-Long Zheng and Bao-Liang Lu ⇤ 1Center for Brain-like Computing and Machine Intelligence Department of Computer Science and Engineering 2Key Laboratory of Shanghai Education Commission for Intelligent Interaction and Cognitive Engineering 3Brain Science and Technology Research Center Shanghai Jiao Tong University, Shanghai, China weilong, bllu @sjtu.edu.cn { } Abstract way to enhance current BCI systems with an increase of infor- mation flow, while at the same time without additional cost. Individual differences across subjects and non- Therefore, aBCIs have attracted increasing interests in both stationary characteristic of electroencephalography research and industry communities, and various studies have (EEG) limit the generalization of affective brain- presented their efficiency and feasibility [Muhl¨ et al., 2014a; computer interfaces in real-world applications. On 2014b; Jenke et al., 2014; Eaton et al., 2015]. Although the the other hand, it is very time consuming and large progress has been obtained about development of aB- expensive to acquire a large number of subject- CIs in recent years, there still exist some challenges such as specific labeled data for learning subject-specific the adaptation to changing environments and individual dif- models. In this paper, we propose to build per- ferences. sonalized EEG-based affective models without la- beled target data using transfer learning techniques. Until now, most of studies emphasized choices of fea- We mainly explore two types of subject-to-subject tures and classifiers [Singh et al., 2007; Jenke et al., 2014; transfer approaches. One is to exploit shared struc- Abraham et al., 2014] and neglected to consider individual ture underlying source domain (source subject) and differences in target persons. They focus on training subject- target domain (target subject). The other is to train specific affective models. However, these approaches are multiple individual classifiers on source subjects practically infeasible in real-world scenarios since they need and transfer knowledge about classifier parameters to collect a large number of labeled data. In addition, the to target subjects, and its aim is to learn a regression calibration phase is time-consuming and annoying. An in- function that maps the relationship between feature tuitive and straightforward way to dealing with this problem distribution and classifier parameters. We compare is to train a generic classifier on the collected data from a the performance of five different approaches on an group of subjects and then make inference on the unseen EEG dataset for constructing an affective model data from a new subject. However, the existing studies in- with three affective states: positive, neutral, and dicated that following this way, the performance of generic negative. The experimental results demonstrate that classifiers were dramatically degraded due to the structural our proposed subject transfer framework achieves and functional variability between subjects as well as the the mean accuracy of 76.31% in comparison with a non-stationary nature of EEG signals [Samek et al., 2013; conventional generic classifier with 56.73% in av- Morioka et al., 2015]. Technically, this issue refers to the erage. covariate-shift challenges [Sugiyama et al., 2007]. The alter- native way to dealing with this problem is to personalize a generic classifier for target subjects in an unsupervised fash- 1 Introduction ion with knowledge transfer from the existing labeled data in hand. Affective brain-computer interfaces (aBCIs) [Muhl¨ et al., 2014a] introduce affective factors into conventional brain- The problem mentioned above has motivated many re- computer interfaces [Chung et al., 2011]. aBCIs provide rele- searchers from different fields in developing transfer learn- vant context information about users affective states in brain- ing and domain adaptation algorithms [Duan et al., 2009; computer interfaces (BCIs) [Zander and Jatzev, 2012], which Pan and Yang, 2010; Chu et al., 2013]. Transfer learning can help BCI systems react adaptively according to users’ af- methods try to transfer knowledge from source domain to tar- fective states, rather in a rule-based fashion. It is an efficient get domain with few or no labeled samples available from subjects of interest, which refer to inductive and transductive ⇤Corresponding author setups, respectively. Figure 1 illustrates the covariate-shift 2732 tive models by adopting two kinds of domain adaptation ap- proaches in an unsupervised manner. One is to find a shared 0.8 common feature space between source and target domains. Negative (Subject 1) 0.6 Neutral (Subject 1) We apply Transfer Component Analysis (TCA) and Kernel Positive (Subject 1) 0.4 Negative (Subject 2) Principle Analysis (KPCA) based methods proposed in [Pan Neutral (Subject 2) 0.2 Positive (Subject 2) et al., 2011]. These methods are adopted to learn a set of 0 common transfer components underlying both the source do- −0.2 Component 3 main and the target domain. When projected to this subspace, −0.4 the difference of feature distributions of both domains can −0.6 be reduced. The other is to construct individual classifiers −0.8 and learn a regression function that maps the relationship be- 0.5 tween data distribution and classifier parameters, which refers 0.6 0.4 0 0.2 to Transductive Parameter Transfer (TPT) [Sangineto et al., 0 −0.2 −0.5 −0.4 2014]. We evaluate the performance of these approaches on −0.6 1 Component 2 −0.8 Component 1 an EEG dataset, SEED , to personalize EEG-based affective models. Figure 1: Illustration of the covariate-shift challenges of con- structing EEG-based affective models. Here, two sample sub- 2 Methods jects (subjects 1 and 2) are with three classes of emotions and EEG features are different in conditional probability distribu- 2.1 TCA-based Subject Transfer tion across subjects. Transfer component analysis (TCA) proposed by Pan et al. learns a set of common transfer components between source domain and target domain [Pan et al., 2011] and finds a challenges of constructing EEG-based affective models. Tra- low-dimensional feature subspace across source domain and ditional machine learning methods have a prior assumption target domain, where the difference of feature distributions that the distributions of training data and test data are inde- between two domains can be reduced. The aim of this pendently and identically distributed (i.i.d.). However, due transfer learning algorithm is to find a transformation φ( ) to the variability from subject to subject, this assumption can · such that P (φ(XS)) P (φ(XT )) and P (YS φ(XS)) not be always satisfied in aBCIs. ⇡ | ⇡ P (YT φ(XT )) without any labeled data in target domain (tar- In this work, we adopt transfer learning algorithms in a get subjects).| An intuitive approach to find the mapping transductive setup (without any labeled samples from target φ( ) is to minimize the Maximum Mean Discrepancy (MMD) subjects) to tackle the subject-to-subject variability for build- [Gretton· et al., 2006] between the empirical means of the two ing EEG-based affective models. Let X be the EEG domains, recording of a sample (X, y), here y represents2X the cor- 2Y C d n1 n2 responding emotion labels. In this case, = R ⇥ , C is 1 s 1 t 2 X MMD(XS0 ,XT0 )= φ(xi ) φ(xi) , (1) the number of channels, and d is the number of time series ||n − n ||H 1 i=1 2 i=1 samples. Let P (X) be the marginal probability distribution X X of X. According to [Pan and Yang, 2010], = ,P(X) D {X } where n1 and n2 represent the sample numbers of source do- is a domain, which in our case is a given subject from which main and target domain, respectively. However, φ( ) is usu- we record the EEG signals. The source and target domains in ally highly nonlinear and a direct optimization with· respect this paper share the same feature space, S = T , but the re- [ X X to φ( ) can easily get stuck in poor local minima Pan et al., spective marginal probability distributions are different, that 2011]·. is, P (XS) = P (XT ). The key assumption in most domain TCA is a dimensionality reduction based domain adapta- adaptation methods6 is that P (Y X )=P (Y X ). S| S T | T tion method. It embeds both the source and target domain Recently, Jayaram and colleges made a timely survey on data into a shared low-dimensional latent space using a map- current transfer learning techniques for BCIs [Jayaram et al., ping φ. Specially, let the Gram matrices defined on the source 2016]. Morioka et al. proposed to learn a common dictio- domain, target domain and cross-domain data in the embed- nary shared by multiple subjects and used the resting-state ded space be KS,S , KT,T , and KS,T , respectively. The kernel activity of a previously unseen target subject as calibration matrix K is defined on all the data as data for compensating for individual differences, rather than task sessions [Morioka et al., 2015]. Krauledat and colleges KS,S KS,T (n1+n2) (n1+n2) K = R ⇥ . (2) KT,S KT,T 2 proposed a zero-training framework for extracting prototyp- ical spatial filters that have better generalization properties By virtue of kernel trick, the MMD distance can be rewritten [Krauledat et al., 2008]. Although most of these methods are 2 based on the variants of common spacial patterns (CSP) for as tr(KL), where K =[φ(xi)>φ(xj)], and Lij =1/n1 if x ,x X , else L =1/n2 if x ,x X , otherwise, motor-imagery paradigms, some studies focused on passive i j 2 S ij 2 i j 2 T [ ] ⇠ (n1+n2) m BCIs Wu et al., 2013 and EEG-based emotion recognition Lij = (1/n1n2).
Details
-
File Typepdf
-
Upload Time-
-
Content LanguagesEnglish
-
Upload UserAnonymous/Not logged-in
-
File Pages7 Page
-
File Size-