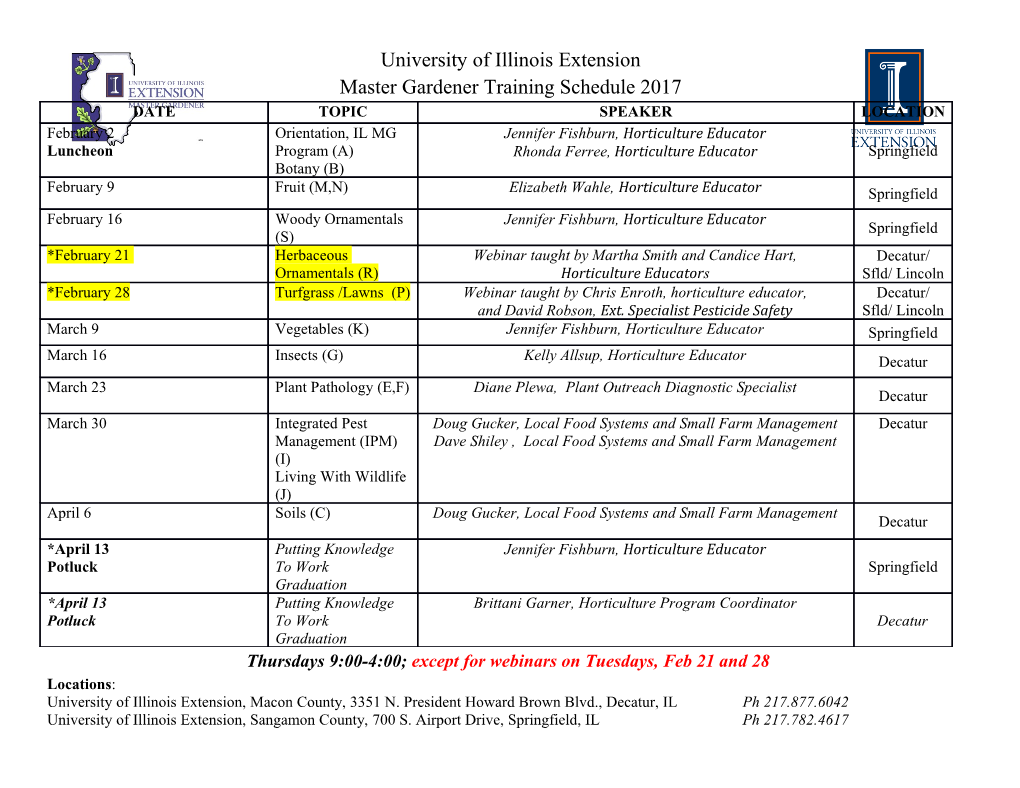
View metadata, citation and similar papers at core.ac.uk brought to you by CORE provided by Springer - Publisher Connector Solving Ordinary Differential Equations 4 Differential equations constitute one of the most powerful mathematical tools to understand and predict the behavior of dynamical systems in nature, engineering, and society. A dynamical system is some system with some state, usually expressed by a set of variables, that evolves in time. For example, an oscillating pendulum, the spreading of a disease, and the weather are examples of dynamical systems. We can use basic laws of physics, or plain intuition, to express mathematical rules that govern the evolution of the system in time. These rules take the form of differential equations. You are probably well experienced with equations, at least equations like axCb D 0 or ax2 CbxCc D 0. Such equations are known as algebraic equations, and the unknown is a number. The unknown in a differential equation is a function, and a differential equation will almost always involve this function and one or more derivatives of the function. For example, f 0.x/ D f.x/ is a simple differential equation (asking if there is any function f such that it equals its derivative – you might remember that ex is a candidate). The present chapter starts with explaining how easy it is to solve both single (scalar) first-order ordinary differential equations and systems of first-order differ- ential equations by the Forward Euler method. We demonstrate all the mathematical and programming details through two specific applications: population growth and spreading of diseases. Then we turn to a physical application: oscillating mechanical systems, which arise in a wide range of engineering situations. The differential equation is now of second order, and the Forward Euler method does not perform well. This observa- © The Author(s) 2016 95 S. Linge, H.P. Langtangen, Programming for Computations – Python, Texts in Computational Science and Engineering 15, DOI 10.1007/978-3-319-32428-9_4 96 4 Solving Ordinary Differential Equations tion motivates the need for other solution methods, and we derive the Euler-Cromer scheme1, the 2nd- and 4th-order Runge-Kutta schemes, as well as a finite difference scheme (the latter to handle the second-order differential equation directly without reformulating it as a first-order system). The presentation starts with undamped free oscillations and then treats general oscillatory systems with possibly nonlinear damping, nonlinear spring forces, and arbitrary external excitation. Besides de- veloping programs from scratch, we also demonstrate how to access ready-made implementations of more advanced differential equation solvers in Python. As we progress with more advanced methods, we develop more sophisticated and reusable programs, and in particular, we incorporate good testing strategies so that we bring solid evidence to correct computations. Consequently, the beginning with population growth and disease modeling examples has a very gentle learning curve, while that curve gets significantly steeper towards the end of the treatment of differential equations for oscillatory systems. 4.1 Population Growth Our first taste of differential equations regards modeling the growth of some pop- ulation, such as a cell culture, an animal population, or a human population. The ideas even extend trivially to growth of money in a bank. Let N.t/ be the number of individuals in the population at time t. How can we predict the evolution of N.t/ in time? Below we shall derive a differential equation whose solution is N.t/.The equation reads N 0.t/ D rN.t/; (4.1) where r is a number. Note that although N is an integer in real life, we model N as a real-valued function. We are forced to do this because the solution of differential equations are (normally continuous) real-valued functions. An integer-valued N.t/ in the model would lead to a lot of mathematical difficulties. With a bit of guessing, you may realize that N.t/ D Cert,whereC is any number. To make this solution unique, we need to fix C , done by prescribing the value of N at some time, usually t D 0.SayN.0/ is given as N0.ThenN.t/ D rt N0e . In general, a differential equation model consists of a differential equation,such as (4.1) and an initial condition,suchasN.0/ D N0. With a known initial con- dition, the differential equation can be solved for the unknown function and the solution is unique. It is, of course, very seldom that we can find the solution of a differential equa- tion as easy as in this example. Normally, one has to apply certain mathematical methods, but these can only handle some of the simplest differential equations. However, we can easily deal with almost any differential equation by applying nu- merical methods and a bit of programming. This is exactly the topic of the present chapter. 1 The term scheme is used as synonym for method or computational recipe, especially in the con- text of numerical methods for differential equations. 4.1 Population Growth 97 4.1.1 Derivation of the Model It can be instructive to show how an equation like (4.1) arises. Consider some population of (say) an animal species and let N.t/ be the number of individuals in a certain spatial region, e.g. an island. We are not concerned with the spatial distribution of the animals, just the number of them in some spatial area where there is no exchange of individuals with other spatial areas. During a time interval t, some animals will die and some new will be born. The number of deaths and births are expected to be proportional to N . For example, if there are twice as many individuals, we expect them to get twice as many newborns. In a time interval t, the net growth of the population will be N.t C t/ N.t/ D bN.t/N dN.t/;N where bN.t/N is the number of newborns and dN.t/N is the number of deaths. If we double t, we expect the proportionality constants bN and dN to double too, so it makes sense to think of bN and dN as proportional to t and “factor out” t.That is, we introduce b D b=tN and d D d=tN to be proportionality constants for newborns and deaths independent of t. Also, we introduce r D b d,whichis the net rate of growth of the population per time unit. Our model then becomes N.t C t/ N.t/ D t rN.t/ : (4.2) Equation (4.2) is actually a computational model. Given N.t/, we can advance the population size by N.t C t/ D N.t/ C t rN.t/ : This is called a difference equation. If we know N.t/ for some t, e.g., N.0/ D N0, we can compute N.t/ D N0 C t rN0; N.2t/ D N.t/ C t rN.t/; N.3t/ D N.2t/ C t rN.2t/; : : N..k C 1/t/ D N.kt/ C t rN.kt/; where k is some arbitrary integer. A computer program can easily compute N..k C 1/t/ for us with the aid of a little loop. Warning Observe that the computational formula cannot be started unless we have an initial condition! The solution of N 0 D rN is N D Cert for any constant C , and the initial condition is needed to fix C so the solution becomes unique. However, from a mathematical point of view, knowing N.t/ at any point t is sufficient as initial 98 4 Solving Ordinary Differential Equations condition. Numerically, we more literally need an initial condition: we need to know a starting value at the left end of the interval in order to get the computa- tional formula going. In fact, we do not need a computer since we see a repetitive pattern when doing hand calculations, which leads us to a mathematical formula for N..k C 1/t/,: N..k C 1/t/ D N.kt/ C t rN.kt/ D N.kt/.1 C t r/ D N..k 1/t/.1 C t r/2 : : kC1 D N0.1 C t r/ : Rather than using (4.2) as a computational model directly, there is a strong tra- dition for deriving a differential equation from this difference equation. The idea is to consider a very small time interval t and look at the instantaneous growth as this time interval is shrunk to an infinitesimally small size. In mathematical terms, it means that we let t ! 0.As(4.2) stands, letting t ! 0 will just produce an equation 0 D 0,sowehavetodividebyt and then take the limit: N.t C t/ N.t/ lim D rN.t/ : t!0 t The term on the left-hand side is actually the definition of the derivative N 0.t/,so we have N 0.t/ D rN.t/; which is the corresponding differential equation. There is nothing in our derivation that forces the parameter r to be constant – it can change with time due to, e.g., seasonal changes or more permanent environ- mental changes. Detour: Exact mathematical solution If you have taken a course on mathematical solution methods for differential equations, you may want to recap how an equation like N 0 D rN or N 0 D r.t/N is solved. The method of separation of variables is the most convenient solution strategy in this case: N 0 D rN dN D rN dt dN D rdt N ZN Zt dN D rdt N N0 0 4.1 Population Growth 99 Zt ln N ln N0 D r.t/dt 0 0 1 Zt @ A N D N0 exp r.t/dt ; 0 rt t which for constant r results in N D N0e .
Details
-
File Typepdf
-
Upload Time-
-
Content LanguagesEnglish
-
Upload UserAnonymous/Not logged-in
-
File Pages66 Page
-
File Size-