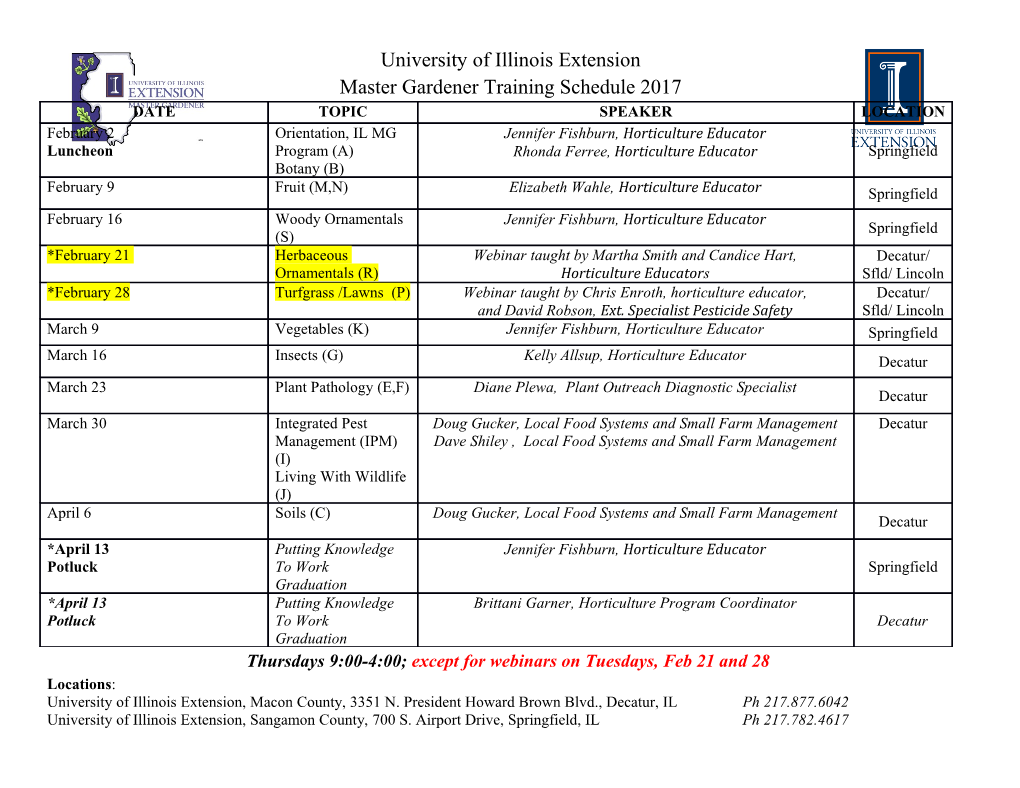
Capturing Domain Semantics with Representation Learning: Applications to Health and Function Dissertation Presented in Partial Fulfillment of the Requirements for the Degree Doctor of Philosophy in the Graduate School of The Ohio State University By Denis R. Newman-Griffis, B.A., M.S. Graduate Program in Computer Science and Engineering The Ohio State University 2020 Dissertation Committee: Prof. Eric Fosler-Lussier, Advisor Prof. Albert M. Lai Prof. Huan Sun Prof. Michael White c Copyright by Denis R. Newman-Griffis 2020 Abstract Natural language processing research is constantly expanding to new domains of text, new types of information, and new applications. A key factor for success in new settings is an ability to capture the characteristics of the language to be analyzed: i.e., the sublanguage of interest. One powerful tool for capturing information about lan- guage use is neural representation learning, a family of methods for mathematically representing words, phrases, and other units of language, based on usage patterns in large text corpora. Representation learning for language is predicated on the ob- servation that lexical usage patterns convey important information about meaning, and models this information in terms of geometric relationships between lexical repre- sentations. Thus, learned representations provide a lens for analyzing and capturing patterns of language use within restricted domains, as well as for general applications. This thesis presents two main contributions to the literature. First, we present a method for moving beyond word-level information to learn representations of do- main concepts from arbitrary text corpora. We demonstrate that these representa- tions capture domain-relevant information about similarity and relatedness, for both biomedical and encyclopedic concepts, and show that they reveal clinically-significant differences in how medical concepts are discussed among different types of health documentation. We further show how concept-level representations learned using a variety of techniques can be effectively combined for semantic grounding of text. ii Second, we present the functional status domain as a new area for NLP analy- sis and application, with far-reaching impact in both healthcare delivery and social benefits administration. We define how functional status information is realized in practical language, and identify rehabilitation medicine documentation as a distinct sublanguage rich in functional status information. Finally, we show that a combi- nation of neural representation learning from well-chosen data sources and modeling techniques informed by the characteristics of functional status information achieve high-quality extraction of mobility-related information from clinical data, helping to address issues of syntactic complexity and poor coverage in standardized vocabular- ies. We conclude by identifying future directions leading from our work, including broader application of representation-based analyses of differences in language use, combination of different representation strategies for NLP applications, and further analyses of the structure of functional status information to guide the development of new representation methods for this domain. iii For Eric Griffis and Robert Bauman, my first professors iv Acknowledgments It takes a village to raise a PhD student, and I've been lucky enough to have several. First and foremost, I want to thank the mentors who have made this journey not just possible, but one of enormous learning and growth. Eric Fosler-Lussier, you have been the best advisor I could ask for. You have constantly pushed me: to think more deeply, communicate more clearly, and to pursue scientific inquiry with thoroughness and an insatiable curiosity. I am a much better scientist, communicator, and teacher for your training|and I remain forever grateful that you never did learn to close your office door to keep me from dropping in with the latest crazy idea. Beth Rasch, your unflagging support throughout this journey has meant the world to me. I have learned an incredible amount from you: about function and health, conducting interdisciplinary research, and keeping a large team running well. Getting the chance to be a part of this team's mission and apply my research to something that can make a real difference has been immensely fulfilling and has set a template I hope to pursue for the rest of my career. I will always be proud to have been a part of the change NIH makes every day. Albert Lai, working with you has been a real pleasure. You've taught me a great deal about medical informatics, about working across disciplinary boundaries, and v about the academic world. Even though most of our work together has been across several states, your perspective and ideas have always been invaluable. To my colleagues in the SLaTe lab: it's been real. Chaitanya Shivade and Joo- Kyung Kim, I learned a lot from our conversations and our work together|not to mention the chess games. Adam Stiff, Deblin Bagchi, Peter Plantinga, and Prashant Serai|complaining about ridiculous bugs, laughing at terrible jokes, and getting donuts won't be the same without you guys. Ryan He, Yi Ma, Manirupa Das, Andy Plummer, and all the other SLaTe lab folks|I've learned a lot about how to be a scientist from working with all of you. To everyone in the Clippers group, especially Michael White, Marie-Catherine de Marneffe, William Schuler, and Micha Elsner: your discussions and presentation feedback have been invaluable over the years, and I will dearly miss trekking over to Oxley every Tuesday. EpiBio, my second scientific family: I have been immensely proud to work as part of this amazing team, and it has been a delight to learn from all of you and to share lunches, SSA meetings, and fabulous baked goods with all of you. Ayah Zirikly, you have been a fantastic co-author, colleague, and friend. Bart Desmet and Guy Divita: it's been great getting to know both of you, and a pleasure to work with you; I look forward to more collaborations (and game nights) in future. Julia Porcino, you have saved my bacon more times than I can count, and talking over ideas with you never fails to bring new insight. Pei-Shu Ho, Jona Camacho Maldonado, and Maryanne Sacco: talking with you is always a joy, and you have taught me so much about annotation and conceptualizing information. Chunxiao Zhou, you have always kept a dozen ships running smoothly, and your curiosity and energy are infectious. To Dr. vi Chan and all the incredible folks in RMD and the Clinical Center, and to everyone else in EpiBio (past and present), an enormous thank you. To my family: I wouldn't be half the person I am today without you. To my father: your joy in and love of learning helped set my on this road, and I'm more proud than I can say to have had you with me on this journey. To my mother: your scholasticism, wit, and endless love are my guiding lights. Matthew: you've always given me something to strive for, and someone to share highs, lows, and games with. To my friends, spread afar from Carleton and here in Columbus: you bring light to my life and a laugh to brighten any cloudy day. I have grown so much in this city, sharing in the Symphony Chorus, the art, the food, the parks, and everything Ohio State has had to offer, and I will take many wonderful memories from my life here. My PhD studies would not have been possible without research funding from multiple sources. My initial studies were supported by a Graduate Administrative Assistantship from the Engineering Career Services office at OSU, and most of my degree was supported by a Pre-Doctoral Fellowship from the NIH Clinical Center, funded in part by the NIH Intramural Research Program and the U.S. Social Security Administration. Finally, to Anna: there are no words. This would not be without you. vii Vita July 23, 1991 . Born - Hobart, IN, USA 2012 . .B.A. Computer Science / Russian, Carleton College, Northfield, MN, USA 2017 . .M.S. Computer Science & Engineering, The Ohio State University, Columbus, OH, USA 2015-present . .Pre-Doctoral Fellow, National Institutes of Health Clinical Center, Bethesda, MD, USA Publications Journal Articles Denis Newman-Griffis, Julia Porcino, Ayah Zirikly, Thanh Thieu, Jonathan Ca- macho Maldonado, Pei-Shu Ho, Min Ding, Leighton Chan, and Elizabeth Rasch. \Broadening horizons: the case for capturing function and the role of health informat- ics in its use." BMC Public Health, (2019) 19:1288. DOI: 10.1186/s12889-019-7630-3 Conference Proceedings Gordon E. Moon, Denis Newman-Griffis, Jinsung Kim, Aravind Sukumaran- Rajam, Eric Fosler-Lussier, and P. Sadayappan. \Parallel Data-Local Training for Optimizing Word2Vec Embeddings for Word and Graph Embeddings." 2019 IEEE/ ACM Workshop on Machine Learning in High Performance Computing Environments (MLHPC), (2019) 1:44-55. DOI: 10.1109/MLHPC49564.2019.00010 Denis Newman-Griffis and Eric Fosler-Lussier. \Writing habits and telltale neigh- bors: analyzing clinical concept usage patterns with sublanguage embeddings." Pro- ceedings of the Tenth International Workshop on Health Text Mining and Information Analysis (LOUHI 2019), (2019) 146-156. DOI: 10.18653/v1/D19-6218 viii Denis Newman-Griffis and Eric Fosler-Lussier. \HARE: a Flexible Highlighting Annotator for Ranking and Exploration." Proceedings of the 2019 Conference on Empirical Methods in Natural Language Processing and the 9th International Joint Conference on Natural Language Processing (EMNLP-IJCNLP): System Demonstra- tions, (2019) 3:85-90. DOI: 10.18653/v1/D19-3015 Denis Newman-Griffis, Ayah Zirikly, Guy Divita, and Bart Desmet. \Classifying the reported ability in clinical mobility descriptions." Proceedings of the 18th BioNLP Workshop and Shared Task, (2019) 1-10. DOI: 10.18653/v1/W19-5001 Brendan Whitaker, Denis Newman-Griffis, Aparajita Haldar, Hakan Ferhatos- manoglu, and Eric Fosler-Lussier. \Characterizing the impact of geometric properties of word embeddings on task performance." Proceedings of the 3rd Workshop on Eval- uating Vector Space Representations for NLP, (2019) 8-17.
Details
-
File Typepdf
-
Upload Time-
-
Content LanguagesEnglish
-
Upload UserAnonymous/Not logged-in
-
File Pages356 Page
-
File Size-