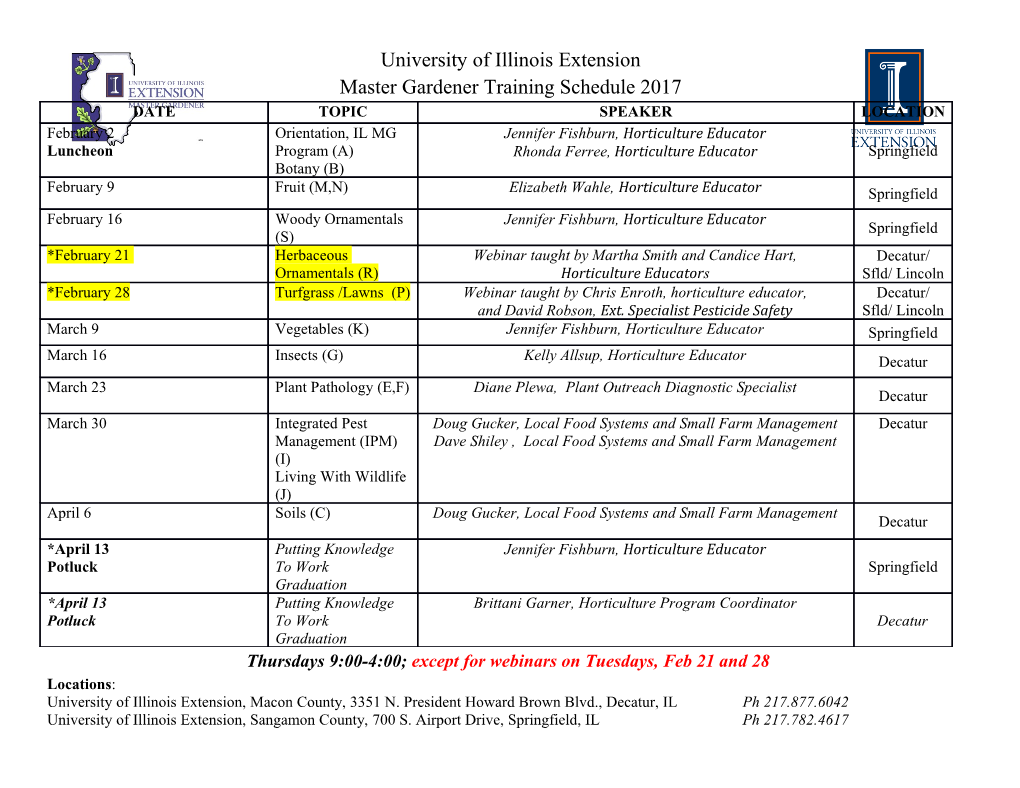
BUSEM at SemEval-2017 Task 4 Sentiment Analysis with Word Embedding and Long Short Term Memory RNN Approaches Deger Ayata1, Murat Saraclar 1, Arzucan Ozgur2 1Electrical & Electronical Engineering Department, Bogaziçi University 2Computer Engineering Department, Bogaziçi University Istanbul , Turkey {deger.ayata, murat.saraclar, arzucan.ozgur}@boun.edu.tr Abstract uses word embeddings for feature representation and Support Vector Machine This paper describes our approach for (SVM), Random Forest (RF) and Naive Bayes SemEval-2017 Task 4: Sentiment Analysis in (NB) algorithms for classification Twitter Twitter. We have participated in Subtask A: messages into negative, neutral and positive Message Polarity Classification subtask and polarity. The second system is based on Long developed two systems. The first system Short Term Memory Recurrent Neural uses word embeddings for feature representation and Support Vector Machine, Networks (LSTM) and uses word indexes as Random Forest and Naive Bayes algorithms sequence of inputs for feature representation. for the classification of Twitter messages into The remainder of this article is structured as negative, neutral and positive polarity. The follows: Section 2 contains information about second system is based on Long Short Term the system description and Section 3 explains Memory Recurrent Neural Networks and methods, models, tools and software packages uses word indexes as sequence of inputs for used in this work. Test cases and datasets are feature representation. explained in Section 4. Results are given in Section 5 with discussions. Finally, section 6 summarizes the conclusions and future work. 1 Introduction 2 System Description Sentiment analysis is extracting subjective information from source materials, via natural We have developed two independent language processing, computational linguistics, systems. The first system is word embedding text mining and machine learning. centric and described in subsection 2.1. The Classification of users’ reviews about a second is LSTM based and described in concept or political view may bring different subsection 2.2. Further details about both opportunities including customer satisfaction systems are given in Section 3. rating, making right recommendations to right target, categorization of users etc. Sentiment 2.1 Word Embedding based System Analysis is often referred to as subjectivity Description analysis, opinion mining and appraisal In word embedding centric system approach, extraction with some connections to affective each word in a tweet is represented with a computing. Sometimes whole documents are vector. Tweets consist of words and vectorial studied as a sentiment unit (Turney and values of words (word vectors) are used to Littman, 2003), but it’s generally agreed that represent tweets as vectorial values. Word sentiment resides in smaller linguistic units Embedding system framework is shown Figure (Pang and Lee, 2008). 1. Two methods are used to obtain word This paper describes our approach for vectors in this work. The first method is based SemEval-2017 Task 4: Sentiment Analysis in on generating word vectors via constructing a Twitter. We have participated in Subtask A: word2vec model from semeval corpus as Message Polarity Classification subtask. We depicted in Figure 1 steps 1 and 2. The second have developed two systems. The first system 777 Proceedings of the 11th International Workshop on Semantic Evaluations (SemEval-2017), pages 777–783, Vancouver, Canada, August 3 - 4, 2017. c 2017 Association for Computational Linguistics method is based on Google News pre-trained contains both training data and test data (steps word vectors model (step 3). 5 and 6). This stage includes also the preprocessing of the tweets, e.g. deleting http Corpus (1) links and twitter user names included in the (3) tweets, deleting the duplicate tweets which Use Google News (2) Generate word2vec pre-trained word occur multiple times on the dataset etc. Later, vectors model model preprocessed and formatted tweets are iterated to generate a tweet vector for each tweets by (4) Word vectors using the words they contained. Therefore, inputs of this stage are the dataset and the model which includes word vectors, while its Vectorize tweets in the (5) dataset (6) output is a set containing tweet vectors, both Vectorize each one of Dataset the tweets in the for the train and the test data. dataset using the word vectors Outputted tweet vectors are in a numerical Tweet (7) format which can be given as an input to vectors multiple machine learning algorithms with the purpose of classifying them into categories or (8) testing an existing model (step 8). At this Train and test a model stage, each tweet in the dataset is represented Train a classifier with train data and test the as a vector with multiple dimensions (step 7). classifier with test data It is possible to train a new classifier model or load a pre-trained and saved model. SVM, RF, and NB classifier models are trained in this (9) Results work. Tweets are categorized into three classes which are negative, neutral and positive (step Figure 1: General framework of the system 9). In the first method, a word2vec model is 2.2 LSTM Based System Description construted by using the entire semeval tweet corpus and a vector space (word2vec model) The pipeline of the second system consists of has been created. This model contains vector many steps : reading Tweets from Semeval values for all unique words in the corpus. datasets, preprocessing Tweets, representing Words which have similar contexts are each word with an index, then representing positioned closer on this space. The parameters each Tweet with a set of word index sequence used in training word2vec model effect the and training a LSTM classifier with sequence performance of the whole framework. index array. The Flowchart of this system is Therefore it is important to find optimal shown in Figure 2. parameter values. This work is focused on the parameter named feature vector dimension size and its impact on the general performance. Dataset Pre-processing Indexing LSTM This parameter determines the dimensionality of the word vectors, which are generated via the word2vec model. Figure 2: LSTM based system pipeline The second method is based on Google News pre-trained word vectors model. This method 3 Methods and Tools uses the Google News pre-trained word vectors model to obtain word vectors as shown in 3.1 Word Embedding Figure 1 step 3. The Google news pre-trained Word embedding stands for a set of natural model is a dictionary which contains word and language processing methods, where words or vectorial value pairs, and it is generated via a phrases from the vocabulary are mapped to word2vec model trained on the Google News vectorial values of real numbers (Bengio et text corpus. al.,2003). Embeddings have been shown to Next stage includes using the obtained word boost the performance of natural language vectors to vectorize tweets in the dataset which processing tasks such as sentiment analysis 778 (Socher et al., 2013). Vector representations of is given in equation (E.4). We have used words can be used in vector operations like Random Forest with the following parameters addition and subtraction. Vectors generated by ={bagSizePercent =100, batchSize=100} word embedding can be used to represent sentences, tweets or whole documents as 푛 {(푥푖, 푦푖)}푖=1 : 푡푟푎푖푛푖푛푔 푠푒푡, vectorial values. There are multiple methods to generate sentence vectors using the word 푦 ∗ : 푝푟푒푑푖푐푡푖표푛푠, vectors, a modified version of the sum 푥′ ∶ 푛푒푤 푝표푖푛푡푠 푡표 푐푙푎푠푠푖푓푦, ′ ′ representation method which is proposed by 푊(푥푖, 푥 )푦푖 ∶ 푤푒푖푔ℎ푡 표푓 푡ℎ푒 푖 푡ℎ 푓푢푛푐푡푖표푛, Blacoe is used in this work (Blacoe et al., 푊 ∶ 푤푒푖푔ℎ푡 푓푢푛푐푡푖표푛, 2012). The sum representation model, in its original 푚 푛 1 state, is generated via summing the vectorial 푦∗ = ∑ ∑ 푊 (푥 , 푥′)푦 푚 푗 푖 푖 embeddings of words which a sentence 푗=1 푖=1 푛 푚 (E.4) contains. The related equations are given 1 = ∑ ( ∑ 푊 (푥 , 푥′)) 푦 below with E.1, E.2 and E.3: 푚 푗 푖 푖 푖=1 푗=1 푇푤푡: 푡푤푒푒푡, 푡푤푡푉푒푐: 푡푤푒푒푡 푣푒푐푡표푟, 푤: 푤표푟푑, 푤푑푉푒푐: 푤표푟푑 푣푒푐푡표푟, 푛: 푛푢푚푏푒푟 표푓 푤표푟푑푠 푖푛 푡푤푒푒푡, 3.2.3 Naïve Bayes (푖) (푖) 푇푤푡푖 = (푤1 , … , 푤푛 ) ∶ words in tweet Naïve-Bayes is a probabilistic classifier based on Bayes’ theorem, based on 푡푤푡푉푒푐[푗] = ∑ 푤푑푉푒푐 [푗] independence of features (John et al, 1995). 푤푘 (E.1) 푘=1,…,푛푖 Mathematical expression is given in equation (E.5). A modified version is used in this work. Derived version considers the number of words. The related 푐 ∗∶ 푝푟푒푑푖푐푡푒푑 푐푙푎푠푠 푥 ∶ 푠푎푚푝푙푒 equations are given below: ℎ푁퐵: 푛푎ï푣푒 푏푎푦푒푠 푓푢푛푐 ( ) ( ) 푇푤푡 = (푤 푖 , … , 푤 푖 ) (E.2) ∗ 푖 1 푛 푐 = ℎ푁퐵(푥) ∑푘=1,…,푛 푤푑푉푒푐푤 [푗] 푡푤푡푉푒푐[푗] = 푖 푘 (E.3) 푛 = 푎푟푔푚푎푥푗=1…푚푃(푐푗) ∏ 푃(푋푖 = 푥푖|푐푗) (E.5) 3.2 Classification Models 3.2.4 Long Short Term Memory Recurrent Neural Nets 3.2.1 Support Vector Machine LSTM networks have similiar architecture to SVM finds a hyper plane seperating tweet Recurrent Neural Nets (RNNs), except that vectors according to their classes while making they use different functions and architecture to the margin as large as possible. After training, compute the hidden state. They were it classifies test records according to which introduced by Hochreiter & Schmidhuber side of the hyperplane their positions are (1997) to avoid the long-term dependency (Fradkin et al, 2000). We have used SVM with problem and were refined and popularized by the following parameters = {Kernel = many people in next studies. PolyKernel, batchSize=100} LSTMs have the form of a chain of 3.2.2 Random Forest repeating modules of a special kind of architecture. The memory in LSTMs are Random forests, first proposed by Ho (Ho, called cells. Internally, these cells decide what 1995) and later improved by Breiman to keep in and what to erase from memory.
Details
-
File Typepdf
-
Upload Time-
-
Content LanguagesEnglish
-
Upload UserAnonymous/Not logged-in
-
File Pages7 Page
-
File Size-