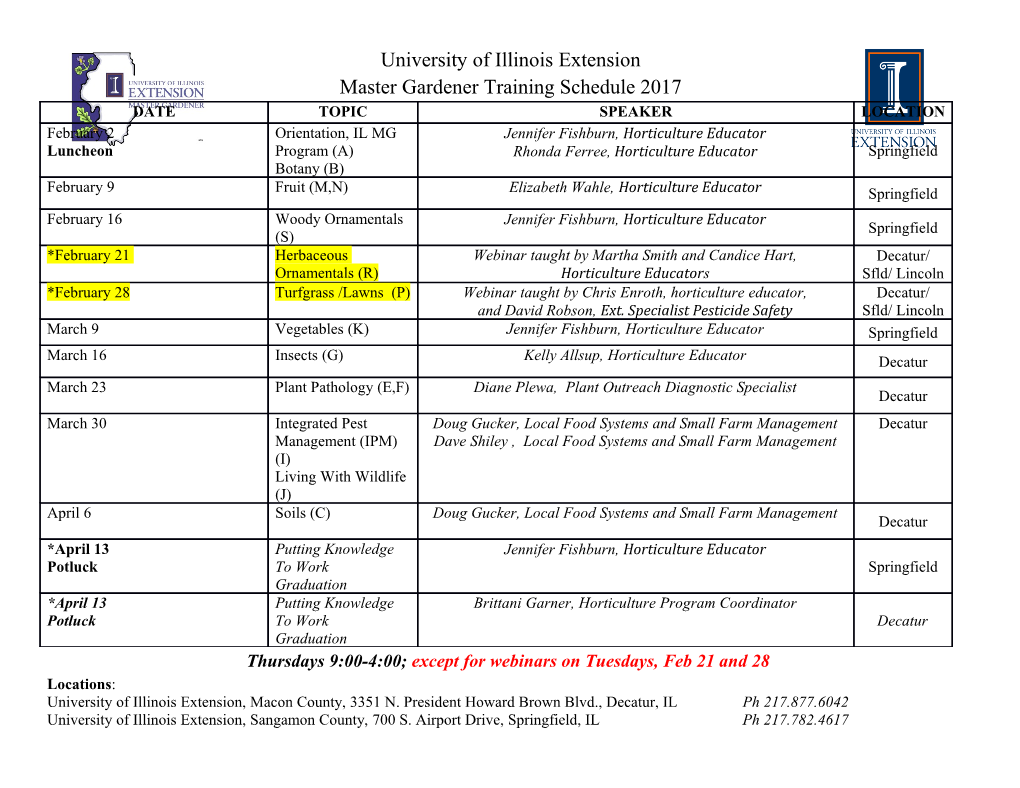
MONITORING THE IMPACTS OF A TAILINGS DAM FAILURE USING SATELLITE IMAGES Jaime Moraga, PhD1 Gurbet Gurkan, MSc2 Prof. Dr. H. Sebnem Duzgun3 ABSTRACT Monitoring dam failures using satellite images provides first responders with efficient management of early interventions. It is also equally important to monitor spatial and temporal changes in the inundation area to track the post-disaster recovery. On January 25th, 2019, the tailings dam of the Córrego do Feijão iron ore mine, located in Brumadinho, Brazil, collapsed. This disaster caused more than 230 fatalities and 30 missing people leading to damage on the order of multiple billions of dollars. This study uses Sentinel-2 satellite images to map the inundation area and assess and delineate the land use and land cover impacted by the dam failure. The images correspond to data captures from January 22nd (3 days before), and February 02 (7 days after the collapse). Satellite images of the region were classified for before and aftermath of the disaster implementing a machine learning algorithm. In order to have sufficient land cover types to validate the quality and accuracy of the algorithm, 7 classes were defined: mine (mining pit, stockpiles, tailings dam), forest, build up (buildings, houses, roads), river, agricultural (plots of land that are either being cultivated or agricultural bare land waiting to be cultivated), clear water (lakes and reservoirs), and grassland (non-forest natural vegetation and yards/parks). The developed classification algorithm yielded a high accuracy (99%) for the image before the collapse. This paper determines land cover impact using two different models, 1) by using the trained network in the “after” image (an approach that can be used as a low cost and first assessment of the impact), and 2) by creating a second network, trained in a subset of points of the “after” image, and then comparing the land cover results of the two trained networks (requires relabeling part of the new data and can delay results, but provides better outcomes). In the first model, applying the trained network to the "after" image, the accuracy is still high (86%), but lower than using the second model (98%). This strategy can be applied at a low cost for monitoring and assessment by using openly available satellite information and, in case of dam collapse or with a larger budget, higher resolution and faster data can be obtained by fly-overs on the area of concern. 1 Colorado School of Mines, Golden, Colorado, [email protected] 2 Colorado School of Mines, Golden, Colorado, [email protected] 3 Colorado School of Mines, Fred Banfield Distinguished Endowed Chair in Mining Engineering, Golden, Colorado, [email protected] USSD 2020 Annual Conference 1 INTRODUCTION During 2019, there have been three tailings dam collapses in Brazil: Córrego de Feijão mine, an iron mine in Brumadinho, Região Metropolitana de Belo Horizonte , in the state of Minas Gerais Jan 25, 2019); Machadinho d'Oeste, a tin mine in Oriente Novo, in the state of Rondônia (March 29, 2019), and; a gold mine in Nossa Senhora do Livramento , in the state of Mato Grosso (Oct 1, 2019). The most devastating one occurred in the Córrego do Feijão iron ore mine, located in Brumadinho on January 25th, 2019. This disaster caused more than 230 fatalities and 30 missing people, leading to damage in the order of multiple billions of dollars (BBC News, 2019). After such a disaster it is important to know the impacted area to make an effective assessment and take immediate measures for relief and recovery. For this purpose, remote sensing can be the most effective and the fastest practice in disaster management (Bello and Aina, 2014), which can assist in damage assessment and aftermath monitoring, providing a quantitative base for relief operations (Westen, 2000). This paper applies a remote sensing methodology based exclusively on openly available multispectral satellite imaging, but companies could use a mix of free-access and privately generated data to increase accuracy and speed of monitoring. Application of machine learning algorithms on remote sensing has been used to improve the results of classification. There are many studies focused on mapping the affected area after a disaster by using combination of satellite imagery with machine learning algorithms. For instance, Syifa et al. (2019) studied the Brumadinho dam collapse in Brazil to map and calculate the dimensions of the flood caused by the collapse using remote sensing. They made a pixel-based classification for the pre- and post-flood images from Landsat-8 and Sentinel-2 applying two different artificial intelligence techniques: artificial neural network (ANN) and support vector machines (SVM). These classifiers were able to determine and calculate the flood area successfully. Luo et al. (2019) analyzed the Bento Rodrigues dam disaster in Brazil by using Landsat ETM+ and OLI images of the disaster area. They tested the performance of SVM and decision tree (DT) classifiers for mapping the changes in land cover caused by the disaster and, instead of just using machine learning algorithms directly on the images, evaluated the effect of adding normalized difference vegetation index (NDVI) as another layer of input data on top of the multi-spectral image from the satellite images. They concluded that NDVI can improve the vegetated land cover types image processing, as opposed to using NDVI or multi-spectral images independently. Besides, SVM gave higher accuracy than DT in classification of land use and land cover map. Junior et al. (2018) combined Unmixing Espectral Linear Model (UELM), artificial neural network, Enhanced Vegetation Index (EVI) and Normalized Difference Vegetation Index (NDVI) to determine the change in vegetation cover in the 2015 Fundão Dam failure, also in Brazil, by using Landsat-8 images. UELM was used to separate soil, shade, and vegetation classes. After that ANN was applied for classification USSD 2020 Annual Conference 2 followed by EVI and NDVI. With this classification, they found out a loss of vegetation of 13.02% occurred as a result of the disaster. In this study, convolutional neural networks (CNN), one of the most useful machine learning algorithms in classification of images, are used to create land cover maps in order to detect the affected area after the Brumadinho tailings dam disaster. The approach combines the use of a CNN tailored for analysis of multispectral images for Land Use Land Cover, LULC by Moraga, et al, (2019), a data gathering and data augmentation approach, and application-specific analysis that takes advantage of the characteristics of the problem to obtain highly accurate maps of the affected areas that include type of area affected. METHODOLOGY This paper uses a CNN tailored for analysis and classification of LULC, the Jigsaw CNN (Moraga, et al, 2019), to classify each point in a satellite image of the impacted area. Based on the classifications made for the before and after images, a change analysis is performed to identify affected area and changes in the LULC. Figure 1 describes the architecture of the Jigsaw CNN. It consists of more than 20 layers organized in 3 blocks. The source data is preprocessed and turned into 17x17 tiles that will become the input to be processed in two parallel structures (A&B), and the results merged and classified in block C. Block A applies convolutions to the image, looking for features and patterns in kernels of sizes 3x3, 5x5 and 7x7, also applying max pooling and a regular convolution to later turn all the results into a single vector for further processing. This results in a classification that identifies patterns that are area specific, but may lose definition or information that is present in each pixel. Block B, on the other hand, uses two layers of dense networks to extract information from each multi-spectral pixel in the image. The concatenated outputs of A & B are processed together in Block C, where an activation function turns them into a class that should match the LULC labels. USSD 2020 Annual Conference 3 Figure 1. Jigsaw architecture, based on Inception The approach in Figure 2 shows the process of acquisition, annotation, classification and evaluation of results, streamlining the effort required to evaluate the area affected and what type of impact the dam failure had in the region. Figure 2 illustrates the process used to train the network. Figure 2. Jigsaw Network Training Process For the input data, pre and post disaster multispectral images were acquired from Sentinel-2 (Figure 3). Sentinel-2 gives global coverage every five days and is equipped with multispectral imager (MSI) with 13 bands (Drusch et al., 2012). This limits the USSD 2020 Annual Conference 4 practical application of this approach in real life, because it may take days before an image is captured and published by ESO on a specific affected area. The spatial resolution of the MSI varies between 10m, 20m, and 60m for different bands (European Space Agency, 2019), so the image was resampled to 10m by 10m pixels by slicing the images with larger resolution into smaller pixels (no interpolation was applied). Figure 3. Visible spectra of the images used. Left is before the collapse, and right is after the collapse of the tailings dam To annotate the image, small representative were selected for seven relevant land use classes (Table 1). Table 1. Classes used for annotation, color used on maps, and class description Class Name Color Description 1 Mine & tailings Red Mining pit, stockpiles, tailings dam Large masses of trees either natural or 2 Forest Green man-made 3 Build up Yellow buildings, houses, roads 4 River Blue Rivers 5 Clear water Cyan Lakes and reservoirs Plots of land that are either being 6 Agricultural Purple cultivated or agricultural bare land waiting to be cultivated Non-forest natural vegetation and 7 Grassland White yards/parks The input for the network consists of small crops of the images – the pieces of the “jigsaw” (Moraga et al.
Details
-
File Typepdf
-
Upload Time-
-
Content LanguagesEnglish
-
Upload UserAnonymous/Not logged-in
-
File Pages12 Page
-
File Size-