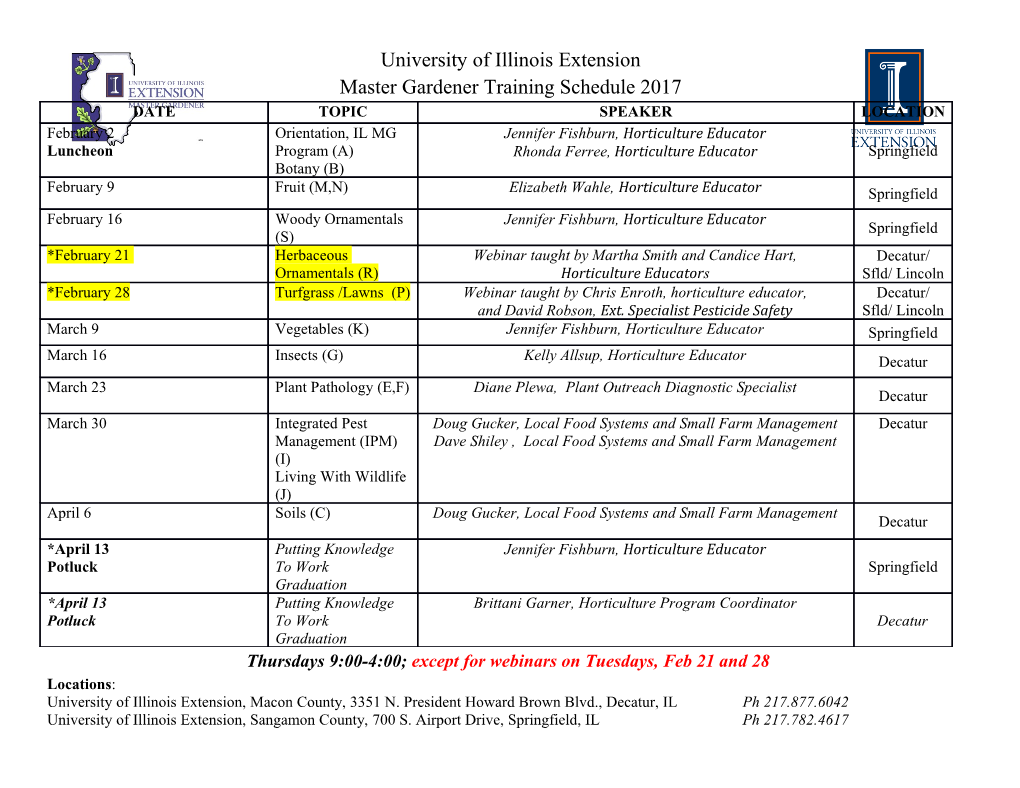
Polynomial Singular Value Decompositions of a Family of Source-Channel Models The MIT Faculty has made this article openly available. Please share how this access benefits you. Your story matters. Citation Makur, Anuran and Lizhong Zheng. "Polynomial Singular Value Decompositions of a Family of Source-Channel Models." IEEE Transactions on Information Theory 63, 12 (December 2017): 7716 - 7728. © 2017 IEEE As Published http://dx.doi.org/10.1109/tit.2017.2760626 Publisher Institute of Electrical and Electronics Engineers (IEEE) Version Author's final manuscript Citable link https://hdl.handle.net/1721.1/131019 Terms of Use Creative Commons Attribution-Noncommercial-Share Alike Detailed Terms http://creativecommons.org/licenses/by-nc-sa/4.0/ IEEE TRANSACTIONS ON INFORMATION THEORY 1 Polynomial Singular Value Decompositions of a Family of Source-Channel Models Anuran Makur, Student Member, IEEE, and Lizhong Zheng, Fellow, IEEE Abstract—In this paper, we show that the conditional expec- interested in a particular subclass of one-parameter exponential tation operators corresponding to a family of source-channel families known as natural exponential families with quadratic models, defined by natural exponential families with quadratic variance functions (NEFQVF). So, we define natural exponen- variance functions and their conjugate priors, have orthonor- mal polynomials as singular vectors. These models include the tial families next. Gaussian channel with Gaussian source, the Poisson channel Definition 1 (Natural Exponential Family). Given a measur- with gamma source, and the binomial channel with beta source. To derive the singular vectors of these models, we prove and able space (Y; B(Y)) with a σ-finite measure µ, where Y ⊆ R employ the equivalent condition that their conditional moments and B(Y) denotes the Borel σ-algebra on Y, the parametrized are strictly degree preserving polynomials. family of probability densities fPY (·; x): x 2 X g with re- Index Terms—Singular value decomposition, natural exponen- spect to µ that have support Y (independent of x) is called a tial family, conjugate prior, orthogonal polynomials. natural exponential family when each density has the form: 8x 2 X ; 8y 2 Y;PY (y; x) = exp (xy − α(x)) PY (y; 0) I. INTRODUCTION PECTRAL and singular value decompositions (SVDs) where PY (·; 0) is a base density function, and: S of conditional expectation operators have many uses in Z information theory and statistics [1]–[3]. As a result, it is 8x 2 X ; α(x) = log exp (xy) PY (y; 0) dµ(y) valuable to analytically determine the singular vectors corre- Y sponding to some widely studied toy models. In this paper, we is known as the log-partition function which satisfies α(0) = 0 illustrate that a certain simple family of source-channel models without loss of generality. The parameter x is called the always has corresponding conditional expectation operators natural parameter, and the natural parameter space X , with orthogonal polynomial singular vectors. We commence fx 2 R : jα(x)j < +1g ⊆ R is defined as the largest interval by presenting this family of models and formally defining where the log-partition function is finite. We usually assume conditional expectation operators in the next two subsections. without loss of generality that 0 2 X . (Here, and throughout this paper, exp(·) and log(·) refer to the natural exponential A. Natural Exponential Families with Quadratic Variance and the natural logarithm with the base e, respectively.) Functions and their Conjugate Priors In [9] and [10], Morris specialized Definition1 further in Since we will study source-channel models that have ex- an effort to justify why certain natural exponential families ponential family and conjugate prior structure, we briefly in- like the Gaussian, Poisson, and binomial enjoy “many useful troduce these notions. Exponential families form an important mathematical properties” [9]. He asserted that the tractability class of distributions in statistics because they are analytically of these distributions stemmed from their quadratic variance tractable and intimately tied to several theoretical phenomena functions. To define this, observe that α(·) is infinitely differ- [4], [5]. For instance, they have sufficient statistics with entiable on X ◦ (the interior of X )[4], and satisfies: bounded dimension after i.i.d. sampling (Pitman-Koopman- 8x 2 X ; α(x) = log [exp (xY )] Darmois theorem) [6], they have conjugate priors [7], they EPY (·;0) (1) ◦ 0 admit efficient estimators that achieve the Cramer-Rao´ bound 8x 2 X ; α (x) = EPY (·;x) [Y ] (2) under a mean parametrization [5], they are maximum entropy ◦ 00 8x 2 X ; α (x) = VARPY (·;x) (Y ) (3) distributions under moment constraints [8], and they are used in tilting arguments in large deviations theory [5]. We are where Y denotes a random variable taking values in Y,(1) is the cumulant generating function of Y , and (3) is the Fisher Manuscript received on December 9, 2015; revised on July 16, 2017; information Y carries about x [5]. Following the exposition in accepted on September 21, 2017. This research was supported by the National 0 + Science Foundation Award 1216476 and a Hewlett-Packard Fellowship. This [9], we may define the variance function V : image(α ) ! R work was presented in part at the 54th Annual Allerton Conference on as the variance of Y written as a function of the mean of Y : Communication, Control, and Computing, 2016. −1 A. Makur and L. Zheng are with the Department of Electrical Engineering 8γ 2 image(α0);V (γ) α00(α0 (γ)) (4) and Computer Science, Massachusetts Institute of Technology, Cambridge, , MA 02139, USA (e-mail: a [email protected]; [email protected]). 0 00 Copyright (c) 2017 IEEE. Personal use of this material is permitted. where α (·) is injective because α (·) is strictly positive in However, permission to use this material for any other purposes must be non-degenerate scenarios. We can now define NEFQVFs. obtained from the IEEE by sending a request to [email protected]. 2 IEEE TRANSACTIONS ON INFORMATION THEORY Definition 2 (NEFQVF). An NEFQVF is a natural exponential probability densities PY jX=x : x 2 X with respect to a σ- family whose variance function V (γ) is a polynomial in γ with finite measure µ on the standard measurable space (Y; B(Y)). degree at most 2. We will use the notation PX and PY to denote the marginal probability laws of X and Y , and we will assume that and We will only analyze channel conditional distribution mod- PX have (measure theoretic) supports X and Y (which are the els P (yjx) = P (y; x) that are NEFQVFs (although we PY Y jX Y closures of X and Y), respectively. Finally, we note that this will not explicitly use the NEFQVF parametrization in our source-channel model defines a joint probability density P calculations). There are six possible NEFQVFs [9]: X;Y on the product measure space (X × Y; B(X ) ⊗ B(Y); λ × µ) 1) Gaussian pdfs with mean parameter and fixed variance such that PX;Y (x; y) = PY jX (yjx)PX (x) for every x 2 X 2) Poisson pmfs with rate parameter and y 2 Y. In sectionII, our channels will be NEFQVFs and 3) binomial pmfs with success probability parameter and our sources will be the corresponding conjugate priors. fixed number of Bernoulli trials We next define the Hilbert spaces and linear operators 4) gamma pdfs with rate parameter and fixed “shape” pertinent to our discussion. Corresponding to the measure 5) negative binomial pmfs with success probability param- space (X ; B(X ); PX ), we define the separable Hilbert space eter and fixed “number of failures” 2 L (X ; PX ) over the field R: 6) generalized hyperbolic secant pdfs (see [9] for details 2 2 regarding this family) L (X ; PX ) , f : X! R j E f (X) < +1 (5) and only the first three will lead to non-degenerate situations. which is the space of all Borel measurable and PX -square Given a channel PY jX (yjx) = PY (y; x) defined by an NE- integrable functions, with correlation as the inner product: FQVF, we will only analyze source distributions that belong 2 8f; g 2 L (X ; X ) ; hf; gi [f(X)g(X)] (6) to the corresponding conjugate prior family. For any natural P PX , E exponential family, we may define a conjugate prior family as and induced norm: shown next [4], [5]. 1 1 2 2 2 2 8f 2 L (X ; X ) ; kfk hf; fi = f (X) : (7) Definition 3 (Conjugate Prior). Suppose we are given a natural P PX , PX E 2 exponential family from Definition1 such that X is a non- Likewise, we define the separable Hilbert space L (Y; PY ) empty open interval defining the measurable space (X ; B(X )) corresponding to the measure space (Y; B(Y); PY ). The condi- with σ-finite measure λ. The corresponding conjugate prior tional expectation operators are maps that are defined between family is the parametrized family of probability densities these Hilbert spaces. The “forward” conditional expectation 2 2 fPX (·; z; n):(z; n) 2 Ξg with respect to λ that have support operator C : L (X ; PX ) !L (Y; PY ) is defined as: X (independent of (z; n)), and are of the form: 2 8f 2 L (X ; PX ) ; (C(f))(y) , E [f(X)jY = y] ; (8) 8x 2 X ;PX (x; z; n) = exp (zx − nα(x) − τ(z; n)) and the “reverse” conditional expectation operator C∗ : 2 2 for any (z; n) 2 Ξ, where the log-partition function τ :Ξ ! R L (Y; PY ) !L (X ; PX ) is defined as: is given by: 2 ∗ 8g 2 L (Y; PY ) ; (C (g))(x) , E [g(Y )jX = x] : (9) Z 8(z; n) 2 Ξ; τ(z; n) = log exp (zx − nα(x)) dλ(x) It is straightforward to verify from (8) and (9) that the X codomains of C and C∗ are indeed Hilbert spaces.
Details
-
File Typepdf
-
Upload Time-
-
Content LanguagesEnglish
-
Upload UserAnonymous/Not logged-in
-
File Pages14 Page
-
File Size-