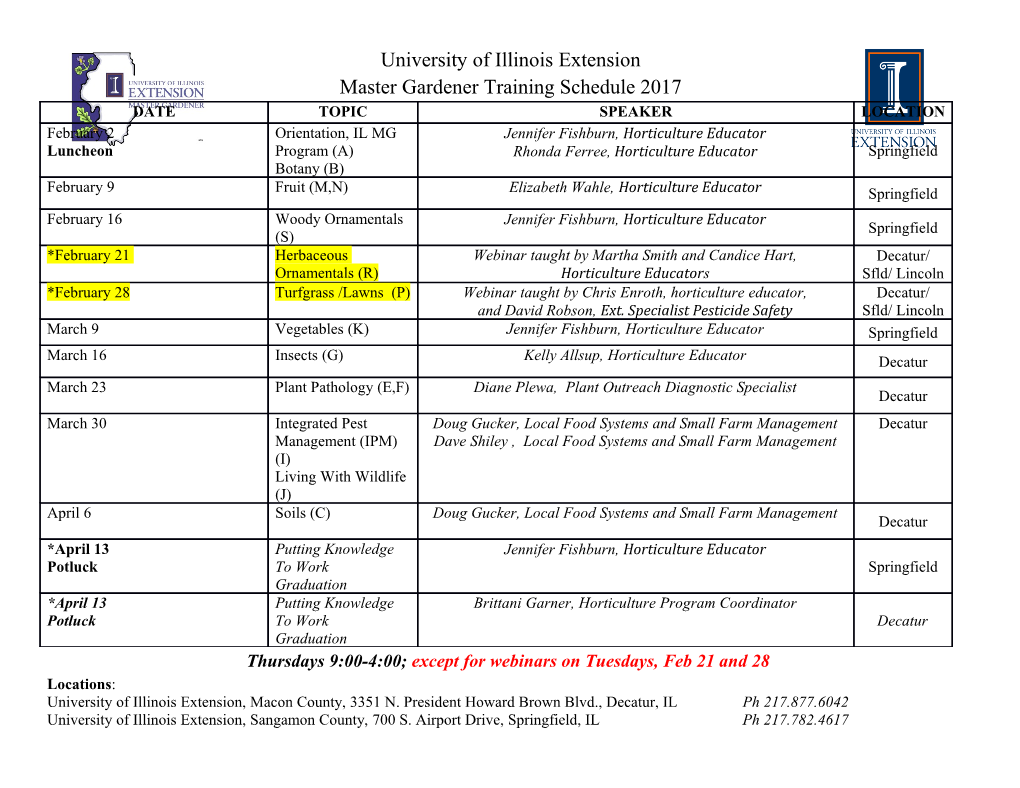
DOCUMENT RETRIEVAL USING A PROBABILISTIC KNOWLEDGE MODEL Shuguang Wang Intelligent Systems Program, University of Pittsburgh, Pittsburgh, PA, USA [email protected] Shyam Visweswaran Department of Biomedical Informatics, University of Pittsburgh, Pittsburgh, PA, USA [email protected] Milos Hauskrecht Department of Computer Science, University of Pittsburgh, Pittsburgh, PA, USA [email protected] Keywords: Information Retrieval, Link Analysis, Domain Knowledge, Biomedical Documents, Probabilistic Model. Abstract: We are interested in enhancing information retrieval methods by incorporating domain knowledge. In this pa- per, we present a new document retrieval framework that learns a probabilistic knowledge model and exploits this model to improve document retrieval. The knowledge model is represented by a network of associa- tions among concepts defining key domain entities and is extracted from a corpus of documents or from a curated domain knowledge base. This knowledge model is then used to perform concept-related probabilistic inferences using link analysis methods and applied to the task of document retrieval. We evaluate this new framework on two biomedical datasets and show that this novel knowledge-based approach outperforms the state-of-art Lemur/Indri document retrieval method. 1 INTRODUCTION Our hypothesis is that highly interconnected domain concepts define semantically relevant groups, and Due to the richness and complexity of scientific do- these patterns are useful in performing information- mains today, published research documents may fea- retrieval inferences, such as connecting hidden and sibly mention only a fraction of knowledge of the do- explicitly mentioned domain concepts in the docu- main. This is not a problem for human readers who ment. are armed with a general knowledge of the domain The analysis of network structures is typically and hence are able to overcome the missing link and done using link analysis methods. We adopt PHITS connect the information in the article to the overall (Cohn and Chang, 2000) to analyze the mutual con- body of domain knowledge. Many existing search nectivity of domain concepts in association networks and information-retrieval systems that operate by an- and derive a probabilistic model that reflects, if the alyzing and matching queries only to individual doc- above hypothesis is correct, the mutual relevance uments are very likely to miss these knowledge-based among domain concepts. Figure 1 illustrates the dis- connections. Hence, many documents that are ex- tinction between our model and existing related tech- tremely relevant to the query may not be returned by niques. The top layer in Figure 1 consists of a set the existing search systems. of documents, and the bottom layer corresponds to Our goal was to study ways of injecting knowl- knowledge and relations among domain concepts (or edge into the information retrieval process in order terms). The two layers are interconnected; individual to find better, more relevant documents that do not documents refer to multiple concepts and cite one an- exactly match the search queries. We present a new other. Latent semantic models, such as LSI (Landauer probabilistic knowledge model learned from the asso- et al., 1998) or PLSI (Hofmann, 1999), focus primar- ciation network relating pairs of domain concepts. In ily on document-term relations. Link analysis such general, associations may stand for and abstract a va- as PHITS is traditionally performed on the top (doc- riety of relations among domain concepts. We believe ument) layer and studies interconnections/citations that association networks and patterns therein give among documents to determine their dependencies. clues about mutual relevance of domain concepts. In this work we use link analysis to study intercon- nectedness of knowledge concepts (knowledge layer) is represented by a graph (network) structure, where and their mutual influences. We believe that the nodes represent domain concepts and arcs between structure and interconnectedness in the knowledge nodes represent the pairwise relations among domain (and also on the document) layer has the potential to concepts. In this paper, we focus on association rela- greatly enhance standard information retrieval infer- tions, that abstract a variety of relations that may exist ences. among domain concepts. Typically, a knowledge model is constructed by a document human expert. An example of such an expert-built layer domain knowledge model is the gene and protein in- teraction network that is available from the Molecu- doc1 doc2 doc3 ... docn lar Signatures Database (MSigDB) 2. Alternatively, a knowledge model can be extracted from existing doc- ument collections by mining and aggregating domain concepts and their relations across many documents. In the work described in this paper, we exper- iment with knowledge models from the biomedical domain with genes and proteins as domain concepts. knowledge We analyze the utility of knowledge models extracted layer from (1) the curated MSigDB database and (2) do- Figure 1: Illustration of the Knowledge Layer. Red dot- main document collections. Knowledge extraction ted arrows represent citations; blue dotted arrows link doc- from MSigDB is done in a simple fashion. Concepts uments to the concepts mentioned in them; and green solid are already defined and the associations are based on lines connect associated concepts. the grouping of different domain concepts, i.e., con- cepts in the same group in the database are considered to be associated. Knowledge extraction from the doc- We experiment with and demonstrate the poten- uments is done in two steps. In the first step, key do- tial of our approach on biomedical research articles main concepts are identified using a dictionary look using search queries on protein and gene species ref- up approach that will be presented in next section. erenced in these articles. Our results show that the In the second step, relations (associations) are iden- addition of the knowledge layer inferences improves tified by analyzing the co-occurrence of pairs of do- the retrieval of relevant articles and outperforms the main concepts in the documents. state-of-the-art information retrieval systems, such as Domain concepts considered in our analysis con- the Lemur/Indri1. sist of genes and proteins. There are several freely This paper is organized as follows. Section 2 available resources that can be used to identify the describes the knowledge model derived from con- names of genes and proteins in text. However, these cept associations, and its construction from different resources are usually optimized with respect to F1 sources. Section 3 describe the learning of the proba- scores. To avoid introducing many false concepts and bilistic knowledge model using PHITS and proposes relations into the model, we developed a method that two inference methods to support document retrieval. is optimized to achieve high precision. Briefly, our An extensive set of the experimental evaluations of method performs the following steps: these methods is presented in Section 4. Several re- cent related projects are reviewed in Section 5 and in 1. Segments abstracts (with titles) into sentences. the final section we provide conclusions and some di- rections for future work. 2. Tags sentences with MedPost POS tagger from NCBI. 3. Parses tagged sentences with Collins’ full parser 2 THE KNOWLEDGE MODEL (Collins, 1999). 4. Matches the phrases with the concept names The knowledge in any scientific domain can be rep- based on the GPSDB (Pillet et al., 2005) vocab- resented as a rich network of relations among the do- ulary. main concepts. Our information retrieval framework adopts this kind of knowledge model to aid in the in- 5. Matches the synonyms of concepts and assigns formation retrieval process. The knowledge model unique id to distinct concepts. 1http://www.lemurproject.org/ 2http://www.broad.mit.edu/gsea/msigdb/ This method archived over 90% precision at approx- P(d) P(z) imately 65% recall when applied to a 100-document d test set to extract genes and proteins. P(z|d) z Once the concepts in the documents are identified, z P(d|z) P(c|z) we mine the associations among the concepts by ana- P(c|z) c lyzing co-occurrences of the concepts on the sentence c d level. Specifically, two concepts are associated and (a) Asymmetric parameterizations (b) Symmetric parameterizations linked in the knowledge model if they co-occur in the same sentence. Figure 2: Graphical representation of PHITS. 3 PROBABILISTIC KNOWLEDGE topics z is smaller than the number of documents d. Using the symmetric parametrization, the model de- MODEL fines P(d;c) as ∑z P(z)P(cjz)P(zjd). The parameters of the PHITS model are learned Our goal is to use the knowledge model represented from the link structure data using the Expectation- as an association network model to support inferences Maximization (EM) approach (Hofmann, 1999; Cohn on relevance among concepts. We propose to do so and Chang, 2000). In the expectation step, it com- by analyzing the interconnectedness of concepts in putes P(zjd;c) and in the maximization step it re- the association network. More specifically, our hy- estimates P(z), P(djz), and P(cjz). pothesis is that domain concepts are more likely to The PHITS model has two important features. be relevant to each other if they belong to the same, First, PHITS (much like the PLSI model) is not a well defined, and highly interconnected group of con- proper generative probabilistic model for documents. cepts. The intuition behind our approach is that con- Latent Dirichlet Allocation (LDA) (Blei et al., 2003) cepts that are semantically interconnected in terms of fixes the problem by using a Dirichlet prior to de- their roles or functions should be considered more rel- fine the latent factor distribution. Second, the PHITS evant to each other. And we expect these semanti- model does not represent individual citations with cally distinct roles and functions to be embedded in multiple random variables, instead citations are linked the documents and hence picked up and reflected in to topics using a multinomial distribution over cita- our association network.
Details
-
File Typepdf
-
Upload Time-
-
Content LanguagesEnglish
-
Upload UserAnonymous/Not logged-in
-
File Pages8 Page
-
File Size-