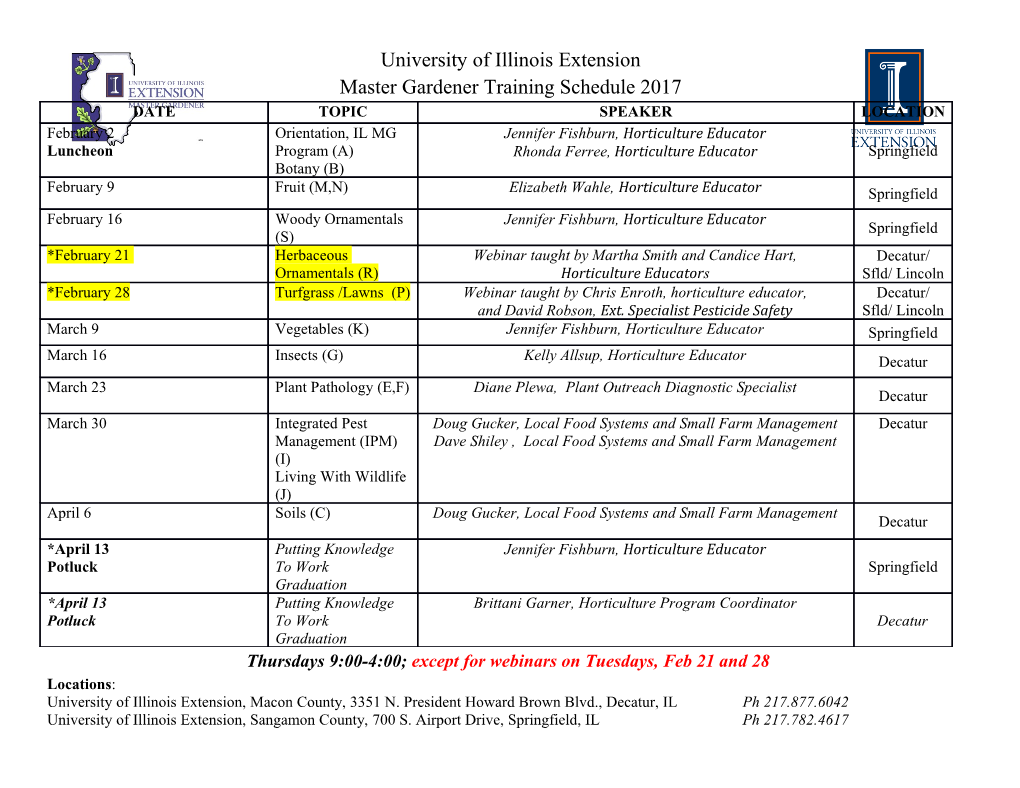
Theoretical Physics Preprint Parameter estimation for power-law distributions by maximum likelihood methods Heiko Bauke Rudolf Peierls Centre for Theoretical Physics, University of Oxford, 1 Keble Road, Oxford, OX1 3NP, United Kingdom∗ Distributions following a power-law are an ubiquitous phenomenon. Methods for determining the exponent of a power-law tail by graphical means are often used in practice but are intrinsically unreliable. Maximum likelihood estimators for the exponent are a mathematically sound alternative to graphical methods. arXiv:0704.1867v2 [cond-mat.other] 11 Aug 2007 . Parameter estimation for power-law distributions by maximum likelihood methods Heiko Bauke Rudolf Peierls Centre for Theoretical Physics, University of Oxford, 1 Keble Road, Oxford, OX1 3NP, United Kingdom∗ (Dated: August 11, 2007) Distributions following a power-law are an ubiquitous phenomenon. Methods for determining the exponent of a power-law tail by graphical means are often used in practice but are intrinsically unreliable. Maximum likelihood estimators for the exponent are a mathematically sound alternative to graphical methods. PACS numbers: 02.50.Tt, 89.75.-k 1. Introduction situations probabilities p(k) may differ from a power-law for k kmax as well, e. g. the distribution may have an exponential cut-off.≥ The distribution of a discrete random variable is referred to as Therefore, I will generalize the maximum likelihood ap- a distribution with a power-law tail if it falls as proach introduced in [3] to distributions that follow a power- γ law within a certain range kmin k < kmax but differ from a p(k) k− (1) ≤ ∼ power-law outside this range in an arbitrary way. Furthermore, I will give some statements about the large sample properties for k N and k kmin. Power-laws are ubiquitous distribu- tions that∈ can be≥ found in many systems from different disci- of the estimate of the power-law exponent and present a nu- plines, see [1, 2] and references therein for some examples. merical procedure to identify the power-law regime of the dis- Experimental data of quantities that follow a power-law are tribution p(k). But first, let us see what is wrong with popular usually very noisy; and therefore obtaining reliable estimates graphical methods. for the exponent γ is notoriously difficult. Estimates that are based on graphical methods are certainly used most often in practice. But simple graphical methods are intrinsically unre- 2. Trouble with graphical methods liable and not able to establish a reliable estimate of the expo- nent γ. For that reason, the authors of [3] introduced an alternative All graphical methods for estimating power-law exponents are approach based on a maximum likelihood estimator for the based on a linear least squaresfit of some empirical data points exponent γ. Unfortunately the authors concentrate on a rather (x1,y1), (x2,y2),..., (xM,yM) to the function idealized type of power-law distributions, namely y(x)= a0 + a1x. (4) γ k− p1(k;γ)= (2) The linear least squares fit minimizes the residual ζ(γ,1) N ζ γ M with k , where the normalization constant ( ,1) is given ∆ = ∑(y a a x )2 . (5) ∈ ζ γ i 0 1 i by the Hurwitz- -function which is defined for > 1 and a > i=1 − − 0 by Estimatesa ˆ anda ˆ of the parameters a and a are given by ∞ 0 1 0 1 1 [4] ζ(γ,a)= ∑ . (3) (i + a)γ i=0 ∑M ∑M 2 ∑M ∑M i=1 yi i=1 xi i=1 xi i=1 yixi aˆ0 = − (6) The distribution (2) is characterized by one parameter only, M 2 M 2 M ∑i 1 x ∑i 1 xi and therefore all properties of this distribution (e.g. its mean) = i − = are determined solely by the exponent γ. In many applications and the power-law (2) is too restrictive. If one states that a quantity follows a power-law, then this ∑M ∑M ∑M M i=1 yixi i=1 xi i=1 yi means usually that the tail (k kmin) of the distribution p(k) aˆ1 = − . (7) γ ≥ ∑M 2 ∑M 2 falls proportionally to k− . Probabilities p(k) for k < kmin may M i=1 xi i=1 xi differ from the power-law and admit the possibility to tune − the mean or other characteristics independently of γ. In some The ansatz for the residual (5) and derivation of (6) and (7) are based on several assumptions regarding the data points (xi,yi). It is assumed that there are no statistical uncertain- ties in xi, but yi may contain some statistical error. The errors ∗E-mail:[email protected] in different yi are independent identically distributed random 2 variables with mean zero. In particular the standard devia- Table I: Mean and standard deviation of the distribution of the esti- tion of the error is independent of x . For various graphical i mate for the power-law exponent γ for various methods. All methods methods for the estimation of the exponent of a power-law have been applied to the same data sets of random numbers from distribution these conditions are not met, leading to the poor distribution (2) with γ = 2.5. See text for details. performance of these methods. To illustrate the failure of graphical methods by a computer mean standard deviation method estimate of estimate experiment N = 10000 random numbers mi had been drawn from distribution (2) with γ = 2.5 and an estimate γˆ for the ex- fit on empirical distribution 1.597 0.167 ponent γ was determined by various graphical methods. The fit on cumulative empirical distri- 2.395 0.304 estimator is a random variable and its distribution depends on bution the method that has been used to obtain the estimate. Impor- fit on empirical distribution with 2.397 0.080 tant measures of the quality of an estimator are its mean and logarithmic binninga its standard deviation. If the mean of the estimator equals the fit on cumulative empirical distri- 2.544 0.127 γ true exponent then the estimator is unbiased and estimators bution with logarithmic binning with a distribution that is concentrated around γ are desirable. maximum likelihood 2.500 0.016 For each graphical method a histogram of the distribution of the estimator was calculated to rate the quality of the estimator aIn [3] a similar experiment is reported. For a fit of the logarithmically by repeating the numerical experiment 500 times. binned empirical probability distribution the authors find a systematical bias of 29 %. I cannot reconstruct such a strong bias, instead I get a bias of 5 % The most straight forward (and most unreliable) graphical only. Probably the quality of this method depends on the details of the binning approach is based on a plot of the empirical probability dis- procedure. tributionp ˆ(k) on a double-logarithmic scale. Introducing the indicator function I[ ], which is one if the statement in the brackets is true and else· zero, the empirical probability distri- to a straight line gives much better estimates for the exponent, bution is given by see Figure 1 b. But there is still a small bias to too small values and the distribution of this estimate is rather broad, see Table I. N 1 I Logarithmic binning reduces the noise in the tail of the em- pˆ(k)= ∑ [mi = k] . (8) pirical distributionsp ˆ(k) and Pˆ(k) by merging data points into N i 1 = groups. By introducing the logarithmically scaled boundaries γ γ An estimate ˆ for the power-law exponent is established by i a least squares fit to bi = roundc with some c > 1 (14) (The function roundx rounds x to the nearest integer.) a linear (xi,yi) = (lnk,lnp ˆ(k)) for all k N withp ˆ(k) > 0, (9) ∈ least squares fit is performed to γ ˆ equals the estimate (7) for the slope, see Figure 1a. Because bi+1 1 bi + bi+1 1 − pˆ(k) the lack of data points in the tail of the empirical distribution (xi,yi)= ln − ,ln ∑ (15) γ 2 bi+1 bi this procedure underestimates systematically the exponent , k=bi − ! see Table I. or There are two ways to deal with the sparseness in the tail of the empirical distribution, logarithmic binning and consider- bi+1 1 bi + bi+1 1 − Pˆ(k) ing the empirical cumulative distribution Pˆ(k) instead ofp ˆ(k). (xi,yi)= ln − ,ln ∑ , (16) 2 bi+1 bi The cumulative probability distribution of (2) is defined by k=bi − ! ∞ i γ respectively. As a consequence of the binning the width of P(k)= ∑ − . (10) the distribution of the estimate γˆ of the power-law exponent ζ(γ,1) i=k γ is reduced, see Figure 1c, 1d and Table I. According to If p(k) has a power-law tail with exponent γ then P(k) follows the numerical experiments a fit of the logarithmically binned approximately a power-law with exponent γ 1 because for cumulative distribution gives the best results among graphical k 1 the distribution P(k) can be approximated− by methods. It shows the smallest systematic bias. ≫ All the methods that have been considered so far have a ∞ i γ k1 γ common weakness. In the deviation of (6) and (7) it was as- P(k) − di = − . (11) ≈ k ζ(γ,1) (γ 1)ζ(γ,1) sumed that the standard deviation of the distribution of the Z − error in yi is the same for all data points (xi,yi). But this is The empirical cumulative probability distribution is given by obviously not the case.
Details
-
File Typepdf
-
Upload Time-
-
Content LanguagesEnglish
-
Upload UserAnonymous/Not logged-in
-
File Pages9 Page
-
File Size-