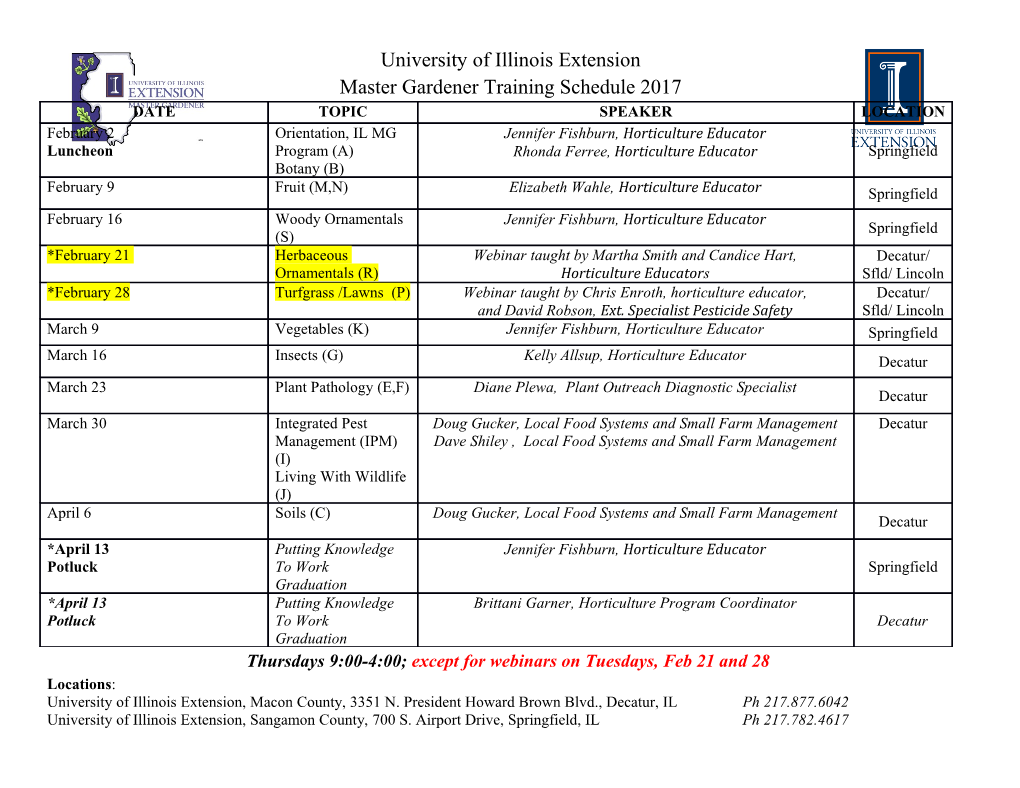
Random Process • A random variable is a function X(e) that maps the set of ex- periment outcomes to the set of numbers. • A random process is a rule that maps every outcome e of an experiment to a function X(t, e). Introduction to Random Processes • A random process is usually conceived of as a function of time, but there is no reason to not consider random processes that are Lecture 12 functions of other independent variables, such as spatial coordi- nates. Spring 2002 • The function X(u, v, e) would be a function whose value de- pended on the location (u, v) and the outcome e, and could be used in representing random variations in an image. Lecture 12 1 Random Process Random Process • e The domain of is the set of outcomes of the experiment. We • A RP is a family of functions, X(t, e). Imagine a giant strip chart assume that a probability distribution is known for this set. recording in which each pen is identified with a different e. This family of functions is traditionally called an ensemble. • The domain of t is a set, T , of real numbers. • A single function X(t, ek) is selected by the outcome ek. This is • If T is the real axis then X(t, e)isacontinuous-time random just a time function that we could call Xk(t). Different outcomes process give us different time functions. • If T is the set of integers then X(t, e)isadiscrete-time random • If t is fixed, say t = t , then X(t ,e) is a random variable. Its process 1 1 value depends on the outcome e. • We will often suppress the display of the variable e and write X(t) • t e X t, e for a continuous-time RP and X[n]orXn for a discrete-time RP. If both and are given then ( ) is just a number. Lecture 12 2 Lecture 12 3 Random Processes Moments and Averages X t ,e ( 1 ) is a random variable that represents the set of samples across t the ensemble at time 1 f x t If it has a probability density function X( ; 1) then the moments are ∞ n n mn(t )=E[X (t )] = x fX (x; t ) dx 1 1 −∞ 1 f x t The notation X( ; 1) may be necessary because the probability density may depend upon the time the samples are taken. µ m , The mean value is X = 1 which may be a function of time. The central moments are ∞ n n E[(X(t ) − µX(t )) ]= (x − µX(t )) fX (x; t ) dx 1 1 −∞ 1 1 Lecture 12 4 Lecture 12 5 Pairs of Samples Covariance and Correlation X t ,e X t ,e The numbers ( 1 ) and ( 2 ) are samples from the same time The covariance of the samples is function at different times. C t ,t E X − µ X − µ ∗ ( 1 2)= [( 1 1)( 2 2) ] X ,X . ∞ They are a pair of random variables ( 1 2) ∗ = (x − µ )(x − µ ) f(x ,x ; t ,t )dx dx −∞ 1 1 2 2 1 2 1 2 1 2 f x ,x t ,t . They have a joint probability density function ( 1 2; 1 2) The correlation function is ∞ From the joint density function one can compute the marginal den- R t ,t E X X∗ x x∗f x ,x t ,t dx dx ( 1 2)= [ 1 ]= 1 ( 1 2; 1 2) 1 2 sities, conditional probabilities and other quantities that may be of 2 −∞ 2 interest. C t ,t R t ,t − µ µ ( 1 2)= ( 1 2) 1 2 Note that both the covariance and correlation functions are conju- t t .Ct ,t C∗ t ,t R t ,t gate symmetric in 1 and 2 ( 1 2)= ( 2 1) and ( 1 2)= R∗ t ,t ( 2 1) Lecture 12 6 Lecture 12 7 Mean-Squared Value Example: Poisson Random Process t N t ,t The “average power” in the process at time is represented by Let ( 1 2) be the number of events produced by a Poisson process in the interval (t ,t ) when the average rate is λ events per second. R(t, t)=E[|X(t)|2] 1 2 The probability that N = n is and C(t, t) represents the power in the fluctuation about the mean n −λτ (λτ) e value. P [N = n]= n! τ t − t . E N t ,t λτ. where = 2 1 Then [ ( 1 2)] = A random process can be defined as the number of events in the interval (0,t). Thus, X(t)=N(0,t). The expected number of events in t is E[X(t)] = λt. Lecture 12 8 Lecture 12 9 Example-continued For a Poisson distribution we know that the variance is E[(X(t) − λt)2]=E[X2(t)] − (λt)2 = λt The “average power” in the function X(t)is E[X2(t)] = λt + λ2t2 A graph of X(t) would show a function fluctuating about an average trend line with a slope λ. Lecture 12 10 Program for Poisson Random Process Random Telegraph Signal FUNCTION PoissonProcess,t,lambda,p ; S=PoissonProcess(t,lambda,p) Consider a random process that has the following properties: ; divides the interval [0,t] into intervals of size ; deltaT=p/lambda where p is sufficiently small so that ; the Poisson assumptions are satisfied. ; ; The interval (0,t) is divided into n=t*lambda/p intervals 1. X(t)=±1, ; and the number of events in the interval (0,k*deltaT) is ; returned in the array S. The maximum length of S is 10000. ; ; USAGE 2. The number of zero crossings in the interval 0,t is described ; S=PoissonProcess(10,1,0.1) ( ) ; Plot,S by a Poisson process ; FOR m=1,10 DO OPLOT,PoissonProcess(10,1,0.1) NP=N_PARAMS() IF NP LT 3 THEN p=0.1 X . n=lambda*t/p 3. (0) = 1 (to be removed later) u=RANDOMN(SEED,n,POISSON=p) s=INTARR(n+1) FOR k=1,n DO s[k]=s[k-1]+u[k-1] RETURN,s Find the expected value at time t. END Lecture 12 12 Lecture 12 14 Random Telegraph Signal Let N(t) equal the number of zero crossings in the interval (0,t). with t ≥ 0. n −λt (λt) e P (N = n)= n! P X t P N [ ( ) = 1] = [ = even number] 2 4 −λt (λt) (λt) = e 1+ + + ··· 2! 4! −λt = e cosh λt P X t − P N [ ( )= 1] = [ = odd number] 3 5 −λt (λt) (λt) = e λt + + + ··· 3! 5! −λt = e sinh λt Lecture 12 15 Random Telegraph Signal Random Telegraph Signal R t ,t The expected value is The autocorrelation function is computed by finding ( 1 2)= E X t X t . x − x [ ( 1) ( 2)] Let 0 = 1 and 1 = 1 denote the two values −λt −λt − λt X t ≥ t . E[X(t)|X(0) = 1] = e cosh λt − e sinh λt = e 2 that can attain. For the moment assume that 2 1 Then 1 1 Note that the expected value decays toward x = 0 for large t. That R(t ,t )= xjxkP [X(t )=xk]P [X(t )=xj|X(t )=xk] t 1 2 1 2 1 happens because the influence of knowing the value at = 0 decays j=0 k=0 exponentially. The first term in each product is given above. To find the conditional probabilities we take note of the fact that the number of sign changes t − t in 2 1 is a Poisson process. Hence, in a manner that is similar to the analysis above, P X t |X t P X t − |X t − [ ( 2)=1 ( 1) = 1] = [ ( 2)= 1 ( 1)= 1] −λ t −t e ( 2 1) λ t − t = cosh ( 2 1) Lecture 12 16 Lecture 12 17 Random Telegraph Signal Random Telegraph Signal The autocorrelation for the telegraph signal depends only upon the P X t − |X t P X t |X t − [ ( 2)= 1 ( 1) = 1] = [ ( 2)=1 ( 1)= 1] time difference, not the location of the time interval. We will see −λ t −t e ( 2 1) λ t − t soon that this is a very important characteristic of stationary random = sinh ( 2 1) processes. Hence −λt −λ t −t −λ t −t We can now remove condition (3) on the telegraph process. Let R(t ,t )= e 1 cosh λt e ( 2 1) cosh λ(t − t ) − e ( 2 1) sinh λ(t − t ) 1 2 1 2 1 2 1 Y t AX t A X −λt −λ t −t −λ t −t ( )= ( )where is a random variable independent of that −e 1 λt e ( 2 1) λ t − t − e ( 2 1) λ t − t sinh 1 cosh ( 2 1) sinh ( 2 1) takes on the values ±1 with equal probability. Then Y (0) will equal ±1 with equal probability, and the telegraph process will no longer After some algebra this reduces to have the restriction of being positive at t =0. −λ t −t R t ,t e ( 2 1) t ≥ t ( 1 2)= for 2 1 Since A and X are independent, the autocorrelation for Y (t) is given by t ≤ t , A parallel analysis applies to the case 2 1 so that −λ|t −t | E [Y (t )Y (t )] = E[A2]E [X(t )X (t )] = e 2 1 −λ|t −t | 1 2 1 2 R(t ,t )=e 2 1 1 2 since E[A2]=1.
Details
-
File Typepdf
-
Upload Time-
-
Content LanguagesEnglish
-
Upload UserAnonymous/Not logged-in
-
File Pages7 Page
-
File Size-