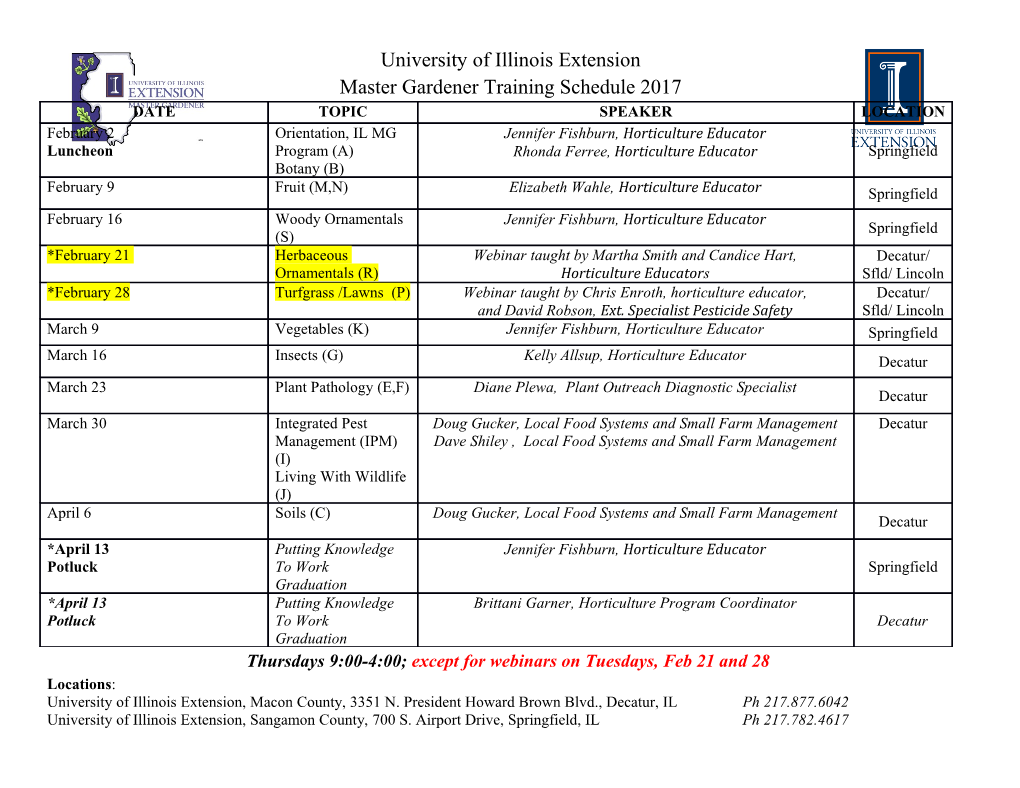
STATISTICS IN MEDICINE Statist. Med. 2004; 23:2729–2744 (DOI: 10.1002/sim.1840) Modelling the distribution of ischaemic stroke-specic survival time using an EM-based mixture approach with random eects adjustment S. K. Ng1;∗;†, G. J. McLachlan1, Kelvin K. W. Yau2 and Andy H. Lee3 1Department of Mathematics; University of Queensland; Brisbane; QLD 4072, Australia 2Department of Management Sciences; City University of Hong Kong; Tat Chee Avenue; Kowloon; Hong Kong 3Department of Epidemiology and Biostatistics; Curtin University of Technology; GPO Box U 1987; Perth; WA 6845; Australia SUMMARY A two-component survival mixture model is proposed to analyse a set of ischaemic stroke-specic mortality data. The survival experience of stroke patients after index stroke may be described by a subpopulation of patients in the acute condition and another subpopulation of patients in the chronic phase. To adjust for the inherent correlation of observations due to random hospital eects, a mixture model of two survival functions with random eects is formulated. Assuming a Weibull hazard in both components, an EM algorithm is developed for the estimation of xed eect parameters and variance components. A simulation study is conducted to assess the performance of the two-component survival mixture model estimators. Simulation results conrm the applicability of the proposed model in a small sample setting. Copyright ? 2004 John Wiley & Sons, Ltd. KEY WORDS: EM algorithm; GLMM; stroke-specic death; survival mixture; Weibull distribution 1. INTRODUCTION Mixture models have been widely used to model failure-time data in a variety of situations [1–5]. As a exible way of modelling data, the mixture approach is directly applicable in situations where the adoption of a single parametric family for the distribution of failure time is inadequate. For example, following open-heart surgery for heart valve replacement, the risk of death can be characterized by three merging phases [6]: an early phase immediately ∗Correspondence to: Angus S. K. Ng, Department of Mathematics, University of Queensland, Brisbane, QLD 4072, Australia. †E-mail: [email protected] Contract=grant sponsor: Australian Research Council Contract=grant sponsor: Research Grants Council of Hong Kong Contract=grant sponsor: National Health and Medical Research Council of Australia Received June 2003 Copyright ? 2004 John Wiley & Sons, Ltd. Accepted December 2003 2730 S. K. NG ET AL. following surgery in which the risk of dying is relatively high, a middle phase of constant risk, and nally a late phase in which the risk of death starts to increase as the patient ages. These phases overlap each other in time and thus cannot be modelled satisfactorily by attempting to t a separate parametric model to each discrete time period; see for example References [4, 7] who specied mixture models for the survival function with three components corresponding to the three phases of death. In this paper, we focus on the modelling of survival experience of ischaemic stroke patients after index stroke. In Australia, there are about 50 000 stroke and transient ischaemic attacks every year, with ischaemic stroke accounting for about 60 per cent of all stroke events [8]. Of all stroke events each year, 70 per cent are rst-ever strokes. Ischaemic stroke is diagnosed by the ICD9-CM [9] and the ICD10 [10] codes and is usually accompanied by acute and chronic phases [11]. In Western Australia, the annual incidence of stroke was estimated at 178 per 100 000. Approximately, around 70 per cent of acute stroke events result in hospitalization and 15 per cent of hospitalized ischaemic stroke patients die within one month [12]. By adopting a mixture model with two components corresponding to the acute and chronic phases, the survival function of time to death T is modelled as S(t; x)=pS1(t; x)+(1− p)S2(t; x) (1) where p denotes the proportion of patients belonging to the acute phase and S1(t; x) and S2(t; x) are the conditional survival functions given that the patient is within the acute and chronic phases, respectively. Here x is a vector of covariates associated with each patient. With this concomitant information, eects of demographic characteristics and co-morbidities on the survival of patients in the acute and chronic phases can then be determined. One issue concerns the heterogeneity due to random hospital eects arising from the clus- tering of patients within the same hospital. Although clinical practice should conform to a designated standard if guidelines on treatment and care have strictly been followed, it is in- evitable that some inherent dierences will still exist among hospitals. Thus, it is expected that observations from patients admitted to the same hospital at index stroke will be cor- related. In this paper, we assume that such inherent hospital eects are random and shared among patients admitted to the same hospital. We extend the survival model (1) to adjust for random hospital eects based on the generalized linear mixed model (GLMM) method of McGilchrist [13]. The method commences from the best linear unbiased predictor (BLUP) and extends to obtain approximate residual maximum likelihood (REML) estimators for the variance component [14]. In addition, we propose the use of the EM algorithm [15] to obtain the BLUP estimate. This EM-based mixture approach has a number of desirable properties, including its simplicity of implementation and reliable global convergence [16, Section 1.7]. Moreover, it does not require the calculation of second derivatives of a conditional likelihood as required with some Newton–Raphson approaches [17] and it does not require Monte Carlo approximation as required with some computationally intensive methods [18, 19]. This paper is organized as follows. In Section 2, we describe the ischaemic stroke-specic mortality data. The two-component survival mixture model with random eects is presented in Section 3. In Section 4, we describe how the EM algorithm is adopted to obtain the BLUP estimate. The analysis of the ischaemic stroke data is presented in Section 5, and in Section 6, we present a simulation study to assess the performance of the proposed EM-based mixture approach in a small sample setting. Finally, some concluding remarks are provided in Section 7. Copyright ? 2004 John Wiley & Sons, Ltd. Statist. Med. 2004; 23:2729–2744 MODELLING THE DISTRIBUTION OF STROKE-SPECIFIC SURVIVAL TIME 2731 2. ISCHAEMIC STROKE-SPECIFIC MORTALITY DATA In this study, hospital separation records corresponding to all ischaemic stroke events from January 1996 to December 1998 were retrieved from the Western Australia Hospital Morbidity Data System. Only those individuals who had an initial hospitalization for ischaemic stroke during the rst six months of 1996 constituted our study cohort and this initial hospitalization was dened as the index stroke. Death data were obtained from the Australian Bureau of Statistics mortality database. Record linkage was used to extract the medical history for each patient from the diagnostic information recorded on hospital separation summaries. A unique patient identier attached by record linkage to all hospital separation records for the same individual facilitated the retrieval of a patient’s medical history. A data set was then compiled containing one record for each person in the cohort discharged from hospital after suering an index stroke. Each record contained information describing the patient’s demographics at the time of the index stroke. Demographic information included age at admission (AGE), gender (SEX), and indigenous status (ABORIGINAL). Clinical information such as a history of diabetes (DIABETES) and atrial brillation (AF) was also included. The data set consists of 557 patients from 56 hospitals. When death occurred, the date and cause of death was included on the patient’s record. Since stroke is generally an old-age disease, death occurrence may be due to stroke or other causes. In this study, the eect of risk factors on the stroke-specic death hazard is investigated with death due to other causes treated as censored observations. The proportion of censored observations is 72.9 per cent. As described in Section 1, the main aim of the study is to identify and assess risk factors aecting the survival of ischaemic stroke patients in the acute and chronic phases, respectively, with an adjustment for random hospital variation. The outcomes of the analysis will thus assist clinicians to rationalize their medical practice, as well as hospital management in terms of budgetary allocation and rehabilitation planning. By comparing the signicant factors between the two phases, appropriate strategy and policies can be prescribed to improve the eciency of service delivery and manage the cost of acute care. In addition, the analysis provides information on inter-hospital variation. As a result, the relative eciency of hospitals may be evaluated based on the predicted random eects. From another clinical perspective, it may also be important to assess the eect of risk factors on the proportion of ischaemic stroke patients in the acute and chronic phases, say, via a logistic function [20] with possibly an adjustment for random hospital variation. Adjusted odds ratio can then be calculated to estimate the relative risk of belonging to the acute phase or the chronic phase. 3. TWO-COMPONENT SURVIVAL MIXTURE MODEL WITH RANDOM EFFECTS Let Tij denote the observable failure=censoring time of the jth individual within the ith hos- pital; let M denote the number of hospitals and ni the number of observations in the ith M hospital, the total number of observations is therefore N = i=1 ni. Let xij be a vector of covariates associated with Tij. The survival function of T is modelled by a two-component mixture model as S(tij; xij)=pS1(tij; xij)+(1− p)S2(tij; xij)(i =1;:::;M; j=1;:::;ni) (2) Copyright ? 2004 John Wiley & Sons, Ltd. Statist.
Details
-
File Typepdf
-
Upload Time-
-
Content LanguagesEnglish
-
Upload UserAnonymous/Not logged-in
-
File Pages16 Page
-
File Size-