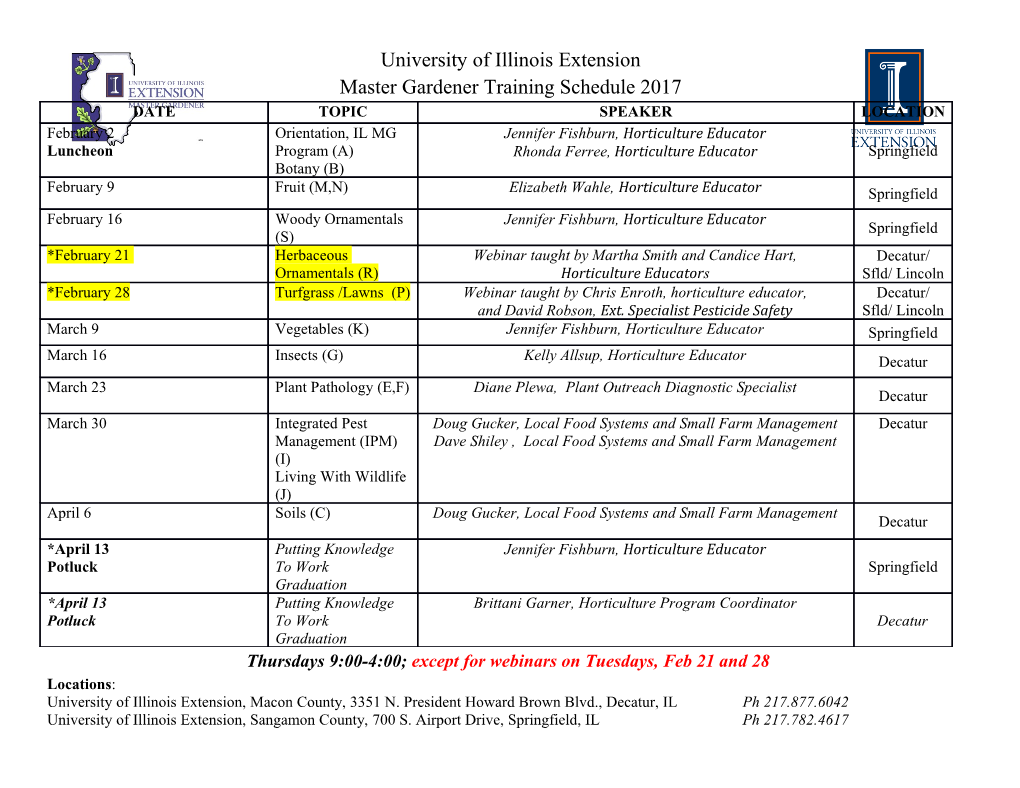
Multiple Linear Regression (MLR) Handouts Yibi Huang • Data and Models • Least Square Estimate, Fitted Values, Residuals • Sum of Squares • Do Regression in R • Interpretation of Regression Coefficients • t-Tests on Individual Regression Coefficients • F -Tests on Multiple Regression Coefficients/Goodness-of-Fit MLR - 1 Data for Multiple Linear Regression Multiple linear regression is a generalized form of simple linear regression, in which the data contains multiple explanatory variables. SLR MLR x y x1 x2 ::: xp y case 1: x1 y1 x11 x12 ::: x1p y1 case 2: x2 y2 x21 x22 ::: x2p y2 . .. case n: xn yn xn1 xn2 ::: xnp yn I For SLR, we observe pairs of variables. For MLR, we observe rows of variables. Each row (or pair) is called a case, a record, or a data point I yi is the response (or dependent variable) of the ith observation I There are p explanatory variables (or covariates, predictors, independent variables), and xik is the value of the explanatory variable xk of the ith case MLR - 2 Multiple Linear Regression Models 2 yi = β0 + β1xi1 + ::: + βpxip + "i where "i 's are i.i.d. N(0; σ ) In the model above, 2 I "i 's (errors, or noise) are i.i.d. N(0; σ ) I Parameters include: β0 = intercept; βk = regression coefficients (slope) for the kth explanatory variable; k = 1;:::; p 2 σ = Var("i ) is the variance of errors I Observed (known): yi ; xi1; xi2;:::; xip 2 Unknown: β0, β1; : : : ; βp, σ , "i 's I Random variables: "i 's, yi 's 2 Constants (nonrandom): βk 's, σ , xik 's MLR - 3 Questions I What are the mean, the variance, and the distribution of yi ? I We assume "i 's are independent. Are yi 's independent? MLR - 4 Fitting the Model | Least Squares Method 14 I Recall for SLR, the least ● squares estimates (βb0; βb1) regression line 10 ● for (β0; β1) is the intercept 8 an arbitrary ● ● ● y ● ● straight line and slope of the straight line 6 ● ● with the minimum sum of 4 2 squared vertical distance to ● the data points 0 Pn 2 0 1 2 3 4 5 6 7 8 i=1(yi − βb0 − βb1xi ) x I MLR is just like SLR. The least squares estimates (βb0; βb1;:::; βbp) for β0; : : : ; βp is the intercept and slopes of the (hyper)plane with the minimum sum of squared vertical distance to the data points n X 2 (yi − βb0 − βb1xi1 − ::: − βbpxip) i=1 MLR - 5 Solving the Least Squares Problem (1) From now on, we use the \hat" symbol to differentiate the estimated coefficient βbj from the actual unknown coefficient βj . To find the (βb0; βb1;:::; βbp) that minimize n X 2 L(βb0; βb1;:::; βbp) = (yi − βb0 − βb1xi1 − ::: − βbpxip) i=1 one can set the derivatives of L with respect to βbj to 0 n @L X = −2 (yi − βb0 − βb1xi1 − ::: − βbpxip) @βb0 i=1 n @L X = −2 xik (yi − βb0 − βb1xi1 − ::: − βbpxip); k = 1; 2;:::; p @βbk i=1 and then equate them to 0. This results in a system of (p + 1) equations in (p + 1) unknowns. MLR - 6 Solving the Least Squares Problem (2) The least square estimate (βb0; βb1;:::; βbp) is the solution to the following system of equations, called normal equations. Pn Pn Pn nβb0 + βb1 i=1 xi1 + ··· + βbp i=1 xip = i=1 yi Pn Pn 2 Pn Pn βb0 i=1 xi1 + βb1 i=1 xi1 + ··· + βbp i=1 xi1xip = i=1 xi1yi . Pn Pn Pn Pn βb0 i=1 xik + βb1 i=1 xik xi1 + ··· + βbp i=1 xik xip = i=1 xik yi . Pn Pn Pn 2 Pn βb0 i=1 xip + βb1 i=1 xipxi1 + ··· + βbp i=1 xip = i=1 xipyi I Don't worry about solving the equations. R and many other softwares can do the computation for us. I In general, βbj 6= βj , but they will be close under some conditions MLR - 7 Fitted Values The fitted value or predicted value: ybi = βb0 + βb1xi1 + ::: + βbpxip I Again, the \hat" symbol is used to differentiate the fitted value ybi from the actual observed value yi . MLR - 8 Residuals I One cannot directly compute the errors "i = yi − β0 − β1xi1 − ::: − βpxip since the coefficients β0; β1; : : : ; βp are unknown. I The errors "i can be estimated by the residuals ei defined as: residual ei = observed yi − predicted yi = yi − ybi = yi − (βb0 + βb1xi1 + ::: + βbpxip) = β0 + β1xi1 + ::: + βpxip + "i − βb0 − βb1xi1 − ::: − βbpxip I ei 6= "i in general since βbj 6= βj I Graphical explanation MLR - 9 Properties of Residuals Recall the least square estimate (βb0; βb1;:::; βbp) satisfies the equations n X (yi − βb0 − βb1xi1 − ::: − βbpxip) = 0 and i=1 | {z } = yi −ybi = ei = residual n X z }| { xik (yi − βb0 − βb1xi1 − ::: − βbpxip) = 0; k = 1; 2;:::; p: i=1 Thus the residuals ei have the properties Xn Xn ei = 0 ; xik ei = 0; k = 1; 2;:::; p: i=1 i=1 | {z } | {z } Residuals add up to 0. Residuals are orthogonal to covariates. The two properties also imply that the residuals are uncorrelated with each of the p covariates. (proof: HW1 bonus). MLR - 10 Sum of Squares Observe that yi − y = (ybi − y) + (yi − ybi ) Squaring up both sides we get 2 2 2 (yi − y) = (ybi − y) + (yi − ybi ) + 2(ybi − y)(yi − ybi ) Summing up over all the cases i = 1; 2;:::; n, we get SST SSR SSE zn }| { zn }| { zn }| { X 2 X 2 X 2 (yi − y) = (ybi − y) + (yi − ybi ) i=1 i=1 i=1 | {z } =ei n X + 2 (ybi − y)(yi − ybi ) i=1 | {z } We'll shortly show this is 0. MLR - 11 Pn Why i=1(ybi − y)(yi − ybi ) = 0? n X (ybi − y)(yi − ybi ) i=1 | {z } =ei n n X X = ybi ei − yei i=1 i=1 n n X X = (βb0 + βb1xi1 + ::: + βbpxip)ei − yei i=1 i=1 n n n n X X X X = βb0 ei +βb1 xi1ei + ::: + βbp xipei −y ei i=1 i=1 i=1 i=1 | {z } | {z } | {z } | {z } =0 =0 =0 =0 = 0 Pn in which we used the properties of residuals that i=1 ei = 0 and Pn i=1 xik ei = 0 for all k = 1;:::; p: MLR - 12 Interpretation of Sum of Squares =e n n n i X 2 X 2 X z }| { 2 (yi − y) = (ybi − y) + (yi − ybi ) i=1 i=1 i=1 | {z } | {z } | {z } SST SSR SSE I SST = total sum of squares I total variability of y I depends on the response y only, not on the form of the model I SSR = regression sum of squares I variability of y explained by x1;:::; xp I SSE = error (residual) sum of squares Pn 2 I = minβ0,β1,...,βp i=1(yi − β0 − β1xi1 − · · · − βpxip) I variability of y not explained by x's MLR - 13 Nested Models We say Model 1 is nested in Model 2 if Model 1 is a special case of Model 2 (and hence Model 2 is an extension of Model 1). E.g., for the 4 models below, Model A : Y = β0 + β1X1 + β2X2 + β3X3 + " Model B : Y = β0 + β1X1 + β2X2 + " Model C : Y = β0 + β1X1 + β3X3 + " Model D : Y = β0 + β1(X1 + X2) + " I B is nested in A . since A reduces to B when β3 = 0 I C is also nested in A. .since A reduces to C when β2 = 0 I D is nested in B . since B reduces to D when β1 = β2 I B and C are NOT nested in either way I D is NOT nested in C MLR - 14 Nested Relationship is Transitive If Model 1 is nested in Model 2, and Model 2 is nested in Model 3, then Model 1 is also nested in Model 3. For example, for models in the previous slide, D is nested in B, and B is nested in A, implies D is also nested in A, which is clearly true because Model A reduces to Model D when β1 = β2; and β3 = 0: When two models are nested (Model 1 is nested in Model 2), I the smaller model (Model 1) is called the reduced model, and I the more general model (Model 2) is called the full model. MLR - 15 SST of Nested Models Question: Compare the SST for Model A and the SST for Model B. Which one is larger? Or are they equal? What about the SST for Model C? For Model D? MLR - 16 SSE of Nested Models When a reduced model is nested in a full model, then (i) SSEreduced ≥ SSEfull ; and (ii) SSRreduced ≤ SSRfull : Proof. We will prove (i) for I the full model yi = β0 + β1xi1 + β2xi2 + β3xi3 + "i and I the reduced model yi = β0 + β1xi1 + β3xi3 + "i : The proofs for other nested models are similar. n X 2 SSEfull = min (y1 − β0 − β1xi1 − β2xi2 − β3xi3) β0,β1,β2,β3 i=1 n X 2 ≤ min (y1 − β0 − β1xi1 − β3xi3) β0,β1,β3 i=1 = SSEreduced Part (ii) follows directly from (i), the identity SST = SSR + SSE, and the fact that all MLR models of the same data set have a common SST MLR - 17 Degrees of Freedom It can be shown that if the MLR model 2 yi = β0 + β1xi1 + ::: + βpxip + "i , "i 's i.i.d. ∼ N(0; σ ) is true then SSE ∼ χ2 ; σ2 n−p−1 If we further assume that β1 = β2 = ··· = βp = 0, then SST SSR ∼ χ2 ; ∼ χ2 σ2 n−1 σ2 p and SSR is independent of SSE. Note the degrees of freedom of the 3 chi-square distributions dfT = n − 1; dfR = p; dfE = n − p − 1 break down similarly dfT = dfR + dfE just like SST = SSR + SSE.
Details
-
File Typepdf
-
Upload Time-
-
Content LanguagesEnglish
-
Upload UserAnonymous/Not logged-in
-
File Pages50 Page
-
File Size-