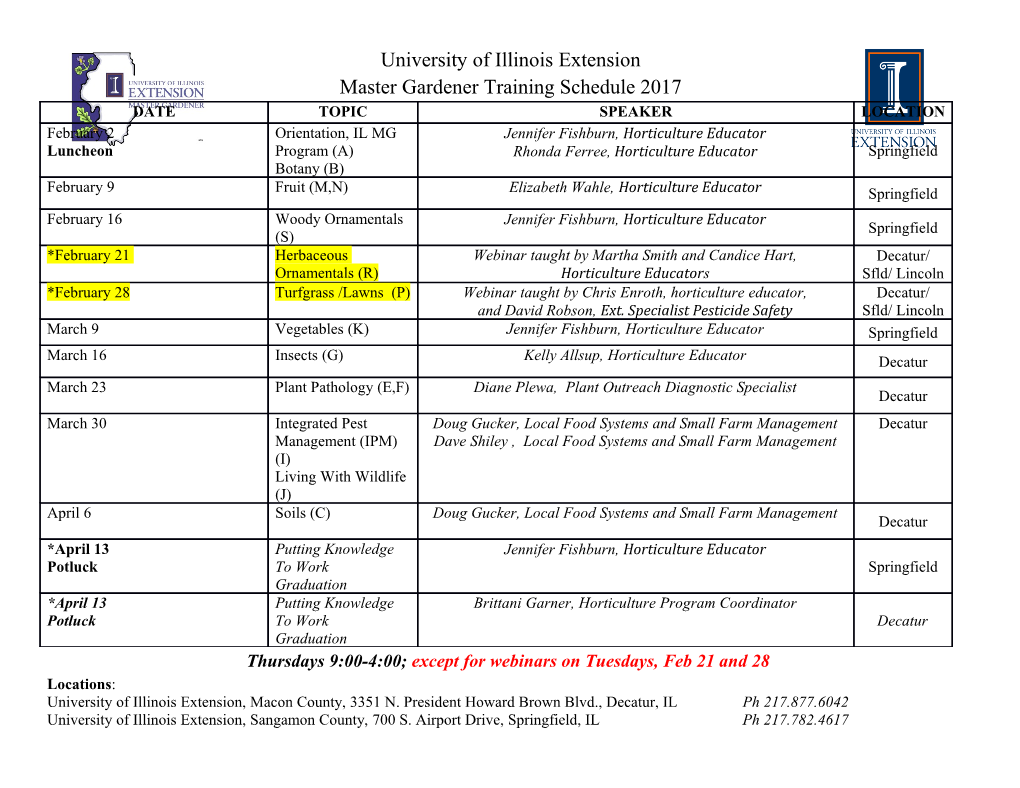
TheTheThe generalgeneralgeneral linearlinearlinear modelmodelmodel andandand StatisticalStatisticalStatistical ParametricParametricParametric MappingMappingMapping I:I:I: IntroductionIntroductionIntroduction tototo thethethe GLMGLMGLM Alexa Morcom and Stefan Kiebel, Rik Henson, Andrew Holmes & J-B Poline Overview • Introduction • Essential concepts – Modelling – Design matrix – Parameter estimates – Simple contrasts • Summary Some terminology • SPM is based on a mass univariate approach that fits a model at each voxel – Is there an effect at location X? Investigate localisation of function or functional specialisation – How does region X interact with Y and Z? Investigate behaviour of networks or functional integration • A General(ised) Linear Model – Effects are linear and additive – If errors are normal (Gaussian), General (SPM99) – If errors are not normal, Generalised (SPM2) Classical statistics... • Parametric – one sample t-test – two sample t-test – paired t-test all cases of the (univariate) – ANOVA General Linear Model – ANCOVA Or, with non-normal errors, the – correlation Generalised Linear Model – linear regression – multiple regression – F-tests – etc… Classical statistics... • Parametric – one sample t-test – two sample t-test – paired t-test all cases of the (univariate) – ANOVA General Linear Model – ANCOVA Or, with non-normal errors, the – correlation Generalised Linear Model – linear regression – multiple regression – F-tests – etc… Classical statistics... • Parametric – one sample t-test – two sample t-test – paired t-test all cases of the (univariate) – ANOVA General Linear Model – ANCOVA Or, with non-normal errors, the – correlation Generalised Linear Model – linear regression – multiple regression – F-tests – etc… • Multivariate? ® PCA/ SVD, MLM Classical statistics... • Parametric – one sample t-test – two sample t-test – paired t-test all cases of the (univariate) – ANOVA General Linear Model – ANCOVA Or, with non-normal errors, the – correlation Generalised Linear Model – linear regression – multiple regression – F-tests – etc… • Multivariate? ® PCA/ SVD, MLM • Non-parametric? ® SnPM Why modelling? Why? MakeMake inferences inferences about about effects effects of of interest interest 1. Decompose data into effects and error How? 1. Decompose data into effects and error 2.2. FormForm statistic statistic using using estimates estimates of of effects effects and and error error Model? UseUse any any available available knowledge knowledge effectseffects estimate estimate statisticstatistic datadata modelmodel errorerror estimate estimate Choose your model “All models are wrong, AA very very general general but some are useful” modelmodel George E.P.Box VarianceVariance BiasBias No normalisation No normalisation LotsLots of of normalisation normalisation defaultdefault No smoothing SPM No smoothing SPM LotsLots of of smoothing smoothing MassiveMassive model model SparseSparse model model Captures signal High sensitivity butbut ... ... not not much much butbut ...... maymay missmiss sensitivitysensitivity signalsignal Modelling with SPM Functional data Functional data DesignDesign matrix matrix ContrastsContrasts SmoothedSmoothed normalisednormalised ParameterParameter datadata GeneralisedGeneralised estimatesestimates PreprocessingPreprocessing linearlinear SPMsSPMs modelmodel Thresholding TemplatesTemplates VarianceVariance components components Thresholding Modelling with SPM DesignDesign matrix matrix ContrastsContrasts PreprocessedPreprocessed data:data: single single voxelvoxel ParameterParameter GeneralisedGeneralised estimatesestimates linearlinear SPMsSPMs modelmodel VarianceVariance components components fMRI example OneOne session session TimeTime series series of of BOLD BOLD responsesresponses in in one one voxel voxel PassivePassive word word listening listening versusversus rest rest 77 cycles cycles of of restrest and and listening listening EachEach epoch epoch 6 6 scans scans withwith 7 7 sec sec TR TR StimulusStimulus function function Question:Question: Is Is there there a a change change in in the the BOLD BOLD responseresponse between between listening listening and and rest? rest? GLM essentials • The model – Design matrix: Effects of interest – Design matrix: Confounds (aka effects of no interest) – Residuals (error measures of the whole model) • Estimate effects and error for data – Parameter estimates (aka betas) – Quantify specific effects using contrasts of parameter estimates • Statistic – Compare estimated effects – the contrasts – with appropriate error measures – Are the effects surprisingly large? GLM essentials • The model – Design matrix: Effects of interest – Design matrix: Confounds (aka effects of no interest) – Residuals (error measures of the whole model) • Estimate effects and error for data – Parameter estimates (aka betas) – Quantify specific effects using contrasts of parameter estimates • Statistic – Compare estimated effects – the contrasts – with appropriate error measures – Are the effects surprisingly large? General Regression model case 2 Time e~N(0, s I) (error is normal and = b1 + b2 + independently and error identically distributed) Intensity x1 x2 e Question:Question: Is Is there there a a change change in in the the BOLD BOLD Hypothesis test: b1 = 0? responseresponse between between listening listening and and rest? rest? (using t-statistic) General Regression model case ˆ ˆ = b1 + b2 + ˆ ˆ Y = b1x1 + b2 x2 + eˆ ModelModel is is specified specified by by 1.1. DesignDesign matrix matrix X X 2.2. AssumptionsAssumptions about about e e Matrix formulation Yi = b1 xi + b2 + ei i = 1,2,3 Y1 = b1 x1 + b2 × 1 + e1 Y2 = b1 x2 + b2 × 1 + e2 Y3 = b1 x3 + b2 × 1 + e3 dummy variables Y1 x1 1 b1 e1 Y2= x2 1 + e2 Y3 x3 1 b1 e3 Y = X b + e ^ Matrix formulation HatsHats = = estimatesestimates Linear regression y Yi Yi = b1 xi + b2 + ei i = 1,2,3 ^b ´ 1 1 ^ Y1 = b1 x1 + b2 × 1 + e1 ´ Y ^ i Y2 = b1 x2 + b2 × 1 + e2 b2 ´ Y3 = b1 x3 + b2 × 1 + e3 x dummy variables ^ ^ Y x 1 b e Parameter estimates b1 & b2 1 1 1 1 ^ ^ Y2= x2 1 + e2 Fitted values Y1 & Y2 Y3 x3 1 b1 e3 ^ ^ Residuals e1 & e2 Y = X b + e Geometrical perspective Y x 1 b e 1 1 1 1 DATA Y2 = x2 1 + e2 (Y1, Y2, Y3) Y3 x3 1 b2 e3 Y Y = b1 ´ X1 +b2 ´ X2 + e (x1, x2, x3) X1 (1,1,1) O X2 design space GLM essentials • The model – Design matrix: Effects of interest – Design matrix: Confounds (aka effects of no interest) – Residuals (error measures of the whole model) • Estimate effects and error for data – Parameter estimates (aka betas) – Quantify specific effects using contrasts of parameter estimates • Statistic – Compare estimated effects – the contrasts – with appropriate error measures – Are the effects surprisingly large? Ordinary least Parameter estimation squares Y = Xb + e bˆ = (X T X )-1 X TY eˆ = Y - Xbˆ residualsresiduals ParameterParameter estimatesestimates EstimateEstimate parameters parameters N 2 LeastLeast squares squares suchsuch that that eˆ minimalminimal å t parameterparameter estimate estimate t=1 Ordinary least Parameter estimation squares Y = Xb + e bˆ = (X T X )-1 X TY eˆ = Y - Xbˆ residualsresiduals = = r r ParameterParameter estimatesestimates ErrorError variance variance s s22== (sum (sum of) of) squared squared residuals residuals standardisedstandardised for for df df == r rTTrr// df df (sum(sum of of squares) squares) …where…where d degreesegreesofof freedom freedom df df(assuming(assuming iid iid):): == N N - -rank(Xrank(X)) (=N(=N--PP if if X X full full rank) rank) Estimation (geometrical) Y x 1 b e 1 1 1 1 DATA Y2 = x2 1 + e2 (Y1, Y2, Y3) Y3 x3 1 b2 e3 Y RESIDUALS Y = b1 ´ X1 +b2 ´ X2 + e ^ ^ ^ ^ T e = (e1,e2,e3) ^ (Y^ ,Y^ ,Y^ ) (x1, x2, x3) Y 1 2 3 X1 (1,1,1) O X2 design space GLM essentials • The model – Design matrix: Effects of interest – Design matrix: Confounds (aka effects of no interest) – Residuals (error measures of the whole model) • Estimate effects and error for data – Parameter estimates (aka betas) – Quantify specific effects using contrasts of parameter estimates • Statistic – Compare estimated effects – the contrasts – with appropriate error measures – Are the effects surprisingly large? Inference - contrasts A contrast = a linear combination of Y = Xb + e parameters: c´ x b à spm_con*img t-test: one-dimensional F-test: tests multiple linear contrast, difference in hypotheses – does subset of means/ difference from model account for zero significant variance DoesDoes boxcar boxcar parameter parameter boxcarboxcar parameter parameter > > 0 0 ? ? modelmodel anything? anything? Null hypothesis: variance Null hypothesis: Null hypothesis: variance Null hypothesis: b1 = 0 ofof tested tested effects effects = = error error variancevariance à spmT_000*.img à ess_000*.img SPM{t} map SPM{F} map t-statistic - example Y = Xb + e Contrast of parameter cT bˆ Contrast of parameter t = estimatesestimates ˆ T ˆ Std(c b) Variance estimate cc = = 1 1 0 0 0 0 0 0 0 0 0 0 0 0 0 0 0 0 0 0 0 0 Variance estimate X StandardStandard error error of of contrast contrast depends depends on on the the design design, , andand is is larger larger with with greater greater residual residual error error and and ‚greater‘‚greater‘ covariance/ covariance/ autocorrelation autocorrelation DegreesDegrees of of freedom freedom d.f. d.f.thenthen = = n n--pp, ,where where n n observations,observations, p pparametersparameters TestsTests for for a adirectionaldirectional differencedifference in in means means F-statistic - example DoDo movement movement parameters parameters (or (or other other confounds)
Details
-
File Typepdf
-
Upload Time-
-
Content LanguagesEnglish
-
Upload UserAnonymous/Not logged-in
-
File Pages35 Page
-
File Size-