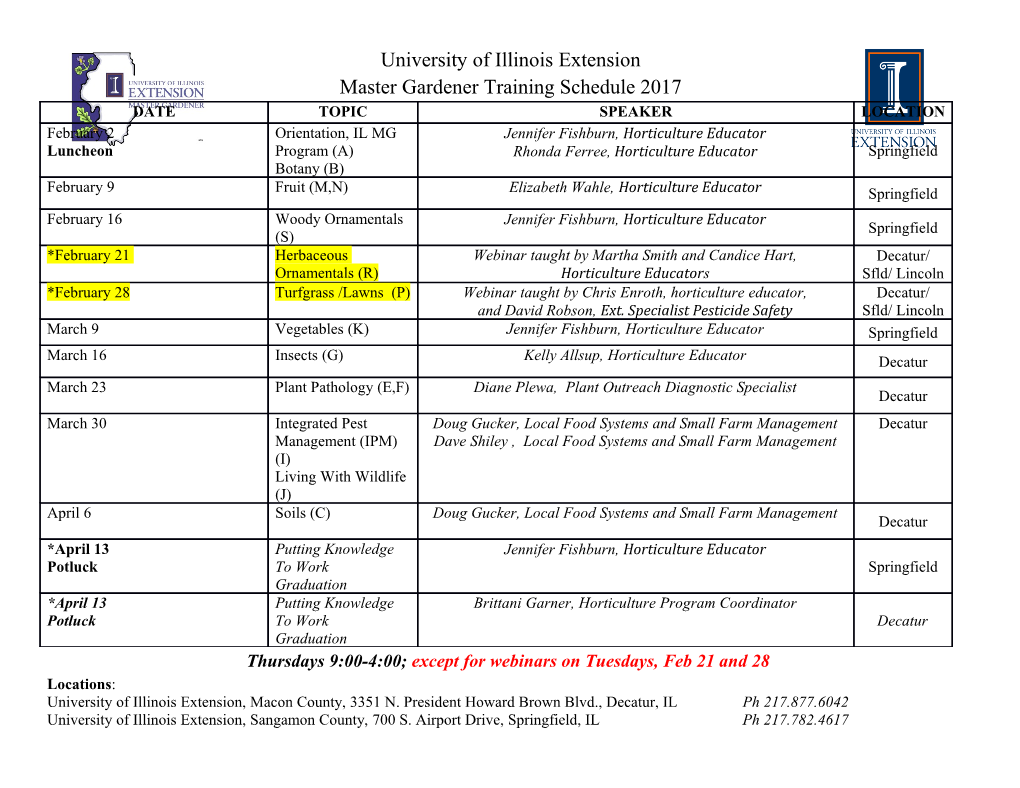
Convex relaxation for the planted clique, biclique, and clustering problems by Brendan P. W. Ames A thesis presented to the University of Waterloo in fulfillment of the thesis requirement for the degree of Doctor of Philosophy in Combinatorics & Optimization Waterloo, Ontario, Canada, 2011 c Brendan P.W. Ames 2011 I hereby declare that I am the sole author of this thesis. This is a true copy of the thesis, including any required final revisions, as accepted by my examiners. I understand that my thesis may be made electronically available to the public. ii Abstract A clique of a graph G is a set of pairwise adjacent nodes of G. Similarly, a biclique (U; V ) of a bipartite graph G is a pair of disjoint, independent vertex sets such that each node in U is adjacent to every node in V in G. We consider the problems of identifying the maximum clique of a graph, known as the maximum clique problem, and identifying the biclique (U; V ) of a bipartite graph that maximizes the product U V , known as the maximum edge biclique problem. We show that finding a clique orj bicliquej · j j of a given size in a graph is equivalent to finding a rank one matrix satisfying a particular set of linear constraints. These problems can be formulated as rank minimization problems and relaxed to convex programming by replacing rank with its convex envelope, the nuclear norm. Both problems are NP-hard yet we show that our relaxation is exact in the case that the input graph contains a large clique or biclique plus additional nodes and edges. For each problem, we provide two analyses of when our relaxation is exact. In the first, the diversionary edges are added deterministically by an adversary. In the second, each potential edge is added to the graph independently at random with fixed probability p. In the random case, our bounds match the earlier bounds of Alon, Krivelevich, and Sudakov, as well as Feige and Krauthgamer for the maximum clique problem. We extend these results and techniques to the k-disjoint-clique problem. The maximum node k-disjoint-clique problem is to find a set of k disjoint cliques of a given input graph containing the maximum number of nodes. Given input graph G and nonnegative edge E weights w R+, the maximum mean weight k-disjoint-clique problem seeks to identify the set of k disjoint2 cliques of G that maximizes the sum of the average weights of the edges, with respect to w, of the complete subgraphs of G induced by the cliques. These problems may be considered as a way to pose the clustering problem. In clustering, one wants to partition a given data set so that the data items in each partition or cluster are similar and the items in different clusters are dissimilar. For the graph G such that the set of nodes represents a given data set and any two nodes are adjacent if and only if the corresponding items are similar, clustering the data into k disjoint clusters is equivalent to partitioning G into k-disjoint cliques. Similarly, given a complete graph with nodes corresponding to a given data set and edge weights indicating similarity between each pair of items, the data may be clustered by solving the maximum mean weight k-disjoint-clique problem. We show that both instances of the k-disjoint-clique problem can be formulated as rank constrained optimization problems and relaxed to semidefinite programs using the nuclear norm relaxation of rank. We also show that when the input instance corresponds to a collection of k disjoint planted cliques plus additional edges and nodes, this semidefinite relaxation is exact for both problems. We provide theoretical bounds that guarantee ex- iii actness of our relaxation and provide empirical examples of successful applications of our algorithm to synthetic data sets, as well as data sets from clustering applications. iv Acknowledgements I wish to thank my advisor and collaborator, Stephen Vavasis. Thank you for your guidance, encouragement, and assistance throughout the research and preparation of this thesis, and for your wise and thoughtful career advice. I am extremely fortunate to have had you as an advisor and mentor during my tenure at the University of Waterloo. I am grateful to Henry Wolkowicz, Levent Tun¸cel,Shai Ben-David, and Inderjit Dhillon for serving on my examination committee and for their helpful comments and suggestions on my thesis. I would especially like to thank both Henry and Inderjit, for independently suggesting I take a look at the graph-partitioning problem, and Shai for his insight on the clustering problem. I would also like to thank Hristo Sendov for providing me with a necessary distraction when I needed a break from thesis work, and for nudging me towards the pursuit of mathematics many years ago. I owe the staff members, faculty members, and my fellow graduate students in the Department of Combinatorics & Optimization a tremendous amount of gratitude. I truly enjoyed my time at the University of Waterloo and have all of you to thank. I am grateful to have received financial support in the form of an NSERC Doctoral Postgraduate Scholarship and an Ontario Graduate Scholarship. Mom and Dad, thank you for your constant support and encouragement, and imparting on me the value of hard work and education. I also thank my sisters Kim and Megan, grandmother Pauline, parents-in-law Keith and Denise, brother-in-law Matthew, and soon to be brother-in-law Ross for all of their love and support. Most of all, I would like to thank my wife, Lauren, for her proofreading expertise, patience, support, and love. I would not have been able to do this without you. v Table of Contents List of Tables x List of Figures xii 1 Introduction 1 1.1 Outline of dissertation . 2 2 Background 3 2.1 Vector and matrix norms . 3 2.2 Rank and the singular value decomposition . 5 2.3 Convex sets, functions, hulls, and cones. 6 2.4 Convex programming . 7 2.5 Semidefinite programming . 9 2.6 Bounds on the norms of random matrices and sums of random variables . 11 2.6.1 Bounds on the tail of the sum of random variables . 11 2.6.2 Bounds on the norms of random matrices . 12 3 Nuclear Norm Relaxation for the Affine Rank Minimization Problem 14 3.1 The Affine Rank Minimization Problem . 14 3.2 Properties of the Nuclear Norm . 16 3.2.1 Convexity . 16 vi 3.2.2 Formulation as a dual norm . 17 3.2.3 Optimality conditions for the affine nuclear norm minimization problem 18 3.3 Cardinality Minimization and Compressed Sensing . 19 3.4 Theoretical guarantees for the success of the nuclear norm relaxation . 22 3.4.1 The restricted isometry property and nearly isometric random matrices 22 3.4.2 Nuclear norm minimization and the low-rank matrix completion prob- lem.................................... 24 3.4.3 Nullspace conditions . 26 3.4.4 Minimizing rank while maximizing sparsity . 27 4 Convex Relaxation for the Maximum Clique and Maximum Edge Bi- clique Problems 29 4.1 The maximum clique problem . 29 4.1.1 Existing approaches for the maximum clique problem . 30 4.1.2 The maximum clique problem as rank minimization . 34 4.1.3 Theoretical guarantees of success for nuclear norm relaxation of the planted clique problem . 36 4.2 The maximum edge biclique problem . 38 4.2.1 Relaxation of the maximum edge biclique problem as nuclear norm minimization . 38 4.3 A general instance of nuclear norm minimization . 40 4.4 Proofs of the theoretical guarantees . 43 4.4.1 The adversarial noise case for the maximum clique problem . 43 4.4.2 The random noise case for the maximum clique problem . 46 4.4.3 The adversarial noise case for the maximum edge biclique problem . 48 4.4.4 The random noise case for the maximum edge biclique problem . 50 vii 5 Semidefinite Relaxations for the Clustering Problem 52 5.1 The clustering and k-disjoint clique problems . 52 5.1.1 Graph-partitioning approaches to clustering . 54 5.2 Theoretical guarantees for the success of convex relaxation of the k-disjoint clique problem . 57 5.2.1 A convex relaxation for the maximum node k-disjoint clique problem 57 5.2.2 A convex relaxation for the maximum mean weight k-disjoint clique problem . 62 5.3 Proof of the theoretical bound for the maximum node k-disjoint-clique problem 66 5.3.1 Optimality conditions for the maximum node k-disjoint-clique problem 66 5.3.2 An upper bound on S~ in the adversarial noise case . 74 k k 5.3.3 An upper bound on S~ in the random noise case . 81 k k 5.4 Proof of Theorem 5.2.3 . 94 5.4.1 Optimality conditions for the maximum mean weight k-disjoint clique problem . 94 5.4.2 A lower bound on λ and η in the planted case . 100 5.4.3 A bound on S~ in the planted case . 106 k k 6 Numerical results 111 6.1 The Maximum Clique Problem . 111 6.2 Random data for KDC ............................ 117 6.3 Random data for WKDC . 121 6.4 Clustering data sets . 125 6.4.1 The Supreme Court data set . 125 6.4.2 Birth and death rates . 127 6.4.3 Image segmentation . 129 7 Conclusions 132 References 135 viii Appendix 151 A Proofs of probabilistic bounds 151 A.1 Proof of Theorem 4.4.2 . 151 A.2 Proof of Theorem 5.3.5 .
Details
-
File Typepdf
-
Upload Time-
-
Content LanguagesEnglish
-
Upload UserAnonymous/Not logged-in
-
File Pages171 Page
-
File Size-