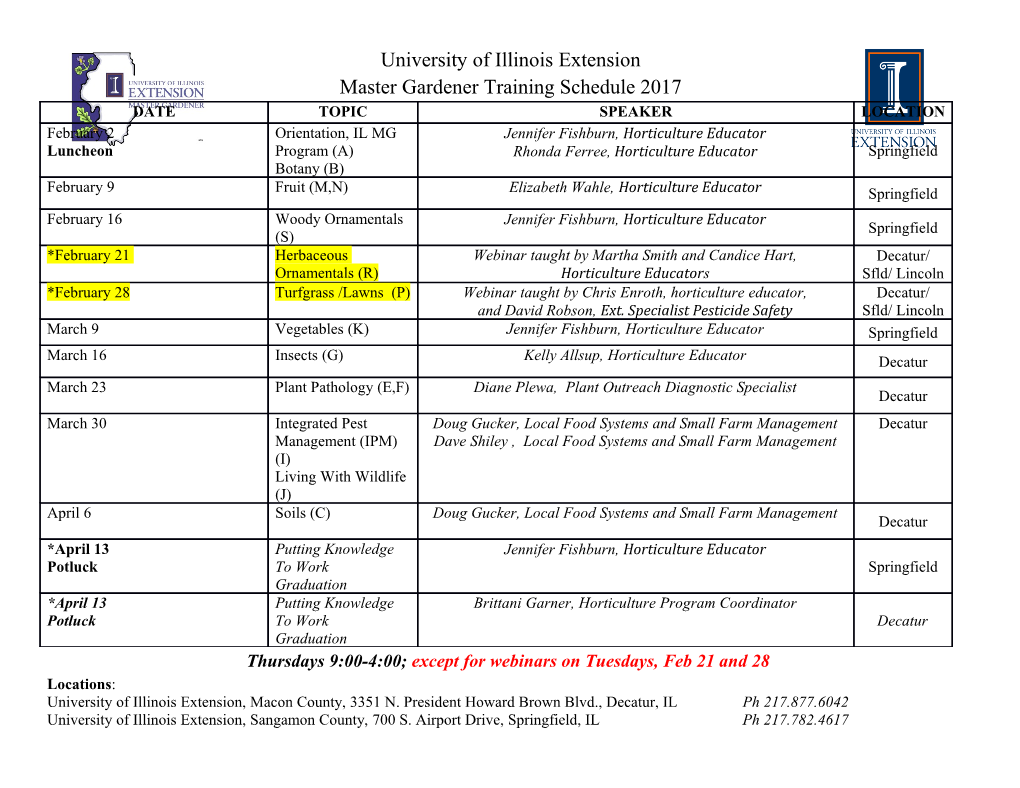
EXTREME RISK INITIATIVE —NYU SCHOOL OF ENGINEERING WORKING PAPER SERIES On the Super-Additivity and Estimation Biases of Quantile Contributions Nassim Nicholas Taleb∗, Raphael Douadyy ∗School of Engineering, New York University yRiskdata & C.N.R.S. Paris, Labex ReFi, Centre d’Economie de la Sorbonne Abstract—Sample measures of top centile contributions to the For a given sample (Xk)1≤k≤n, its "natural" estimator qthpercentile total (concentration) are downward biased, unstable estimators, κ ≡ , used in most academic studies, can be extremely sensitive to sample size and concave in accounting for bq total large deviations. It makes them particularly unfit in domains expressed, as Pn with power law tails, especially for low values of the exponent. 1 ^ Xi κ ≡ i=1 Xi>h(q) These estimators can vary over time and increase with the bq Pn X population size, as shown in this article, thus providing the i=1 i illusion of structural changes in concentration. They are also where h^(q) is the estimated exceedance threshold for the inconsistent under aggregation and mixing distributions, as the weighted average of concentration measures for A and B will probability q : tend to be lower than that from A [ B. In addition, it can be n 1 X shown that under such fat tails, increases in the total sum need h^(q) = inffh : 1 ≤ qg to be accompanied by increased sample size of the concentration n x>h measurement. We examine the estimation superadditivity and i=1 bias under homogeneous and mixed distributions. We shall see that the observed variable κbq is a downward Fourth version, Nov 11 2014 biased estimator of the true ratio κq, the one that would hold out of sample, and such bias is in proportion to the fatness of I. INTRODUCTION tails and, for very fat tailed distributions, remains significant, even for very large samples. Vilfredo Pareto noticed that 80% of the land in Italy belonged to 20% of the population, and vice-versa, thus both giving birth to the power law class of distributions and the II. ESTIMATION FOR UNMIXED PARETO-TAILED popular saying 80/20. The self-similarity at the core of the DISTRIBUTIONS property of power laws [1] and [2] allows us to recurse and Let X be a random variable belonging to the class of reapply the 80/20 to the remaining 20%, and so forth until one distributions with a "power law" right tail, that is: obtains the result that the top percent of the population will −α own about 53% of the total wealth. P(X > x) ∼ L(x) x (1) It looks like such a measure of concentration can be where L :[xmin; +1) ! (0; +1) is a slowly varying seriously biased, depending on how it is measured, so it is L(kx) very likely that the true ratio of concentration of what Pareto function, defined as limx!+1 L(x) = 1 for any k > 0. observed, that is, the share of the top percentile, was closer There is little difference for small exceedance quantiles to 70%, hence changes year-on-year would drift higher to (<50%) between the various possible distributions such as converge to such a level from larger sample. In fact, as we Student’s t, Lévy α-stable, Dagum,[3],[4] Singh-Maddala dis- arXiv:1405.1791v3 [stat.AP] 12 Nov 2014 will show in this discussion, for, say wealth, more complete tribution [5], or straight Pareto. samples resulting from technological progress, and also larger For exponents 1 ≤ α ≤ 2, as observed in [6], the law of population and economic growth will make such a measure large numbers operates, though extremely slowly. The problem converge by increasing over time, for no other reason than is acute for α around, but strictly above 1 and severe, as it expansion in sample space or aggregate value. diverges, for α = 1. The core of the problem is that, for the class one-tailed fat-tailed random variables, that is, bounded on the left and A. Bias and Convergence unbounded on the right, where the random variable X 2 1) Simple Pareto Distribution: Let us first consider φα(x) [xmin; 1), the in-sample quantile contribution is a biased estimator of the true value of the actual quantile contribution. the density of a α-Pareto distribution bounded from below by x > 0; φ (x) = αxα x−α−11 Let us define the quantile contribution min in other words: α min x≥xmin , and xmin α P(X > x) = x . Under these assumptions, the cutpoint E[XjX > h(q)] −1/α κq = q of exceedance is h(q) = xmin q and we have: E[X] R 1 x φ(x)dx h(q) h(q) 1−α α−1 where h(q) = inffh 2 [xmin; +1) ; P(X > h) ≤ qg is the α κq = R 1 = = q (2) exceedance threshold for the probability q: x φ(x)dx xmin xmin 1 EXTREME RISK INITIATIVE —NYU SCHOOL OF ENGINEERING WORKING PAPER SERIES 2 If the distribution of X is α-Pareto only beyond a cut-point of concavity of the concentration measure with respect to xcut, which we assume to be below h(q), so that we have an innovation (a new sample value), whether it falls below λ α Pn 1 P(X > x) = x for some λ > 0, then we still have or above the threshold. Let Ah(n) = i=1 Xi>hXi and h(q) = λq−1/α n Ah(n) and S(n) = P X ; so that κ (n) = and assume a i=1 i bh S(n) α λ α−1 κq = q α frozen threshold h. If a new sample value Xn+1 < h then the α − 1 E [X] Ah(n) new value is κbh(n+1) = : The value is convex The estimation of κ hence requires that of the exponent α as S(n) + Xn+1 q in X so that uncertainty on X increases its expectation. well as that of the scaling parameter λ, or at least its ratio to n+1 n+1 At variance, if the new sample value X > h, the new value X n+1 the expectation of . Ah(n)+Xn+1−h S(n)−Ah(n) κh(n + 1) ≈ = 1 − ; which is Table I shows the bias of κbq as an estimator of κq in the b S(n)+Xn+1−h S(n)+Xn+1−h case of an α-Pareto distribution for α = 1:1, a value chosen now concave in Xn+1; so that uncertainty on Xn+1 reduces its to be compatible with practical economic measures, such as value. The competition between these two opposite effects is in the wealth distribution in the world or in a particular country, favor of the latter, because of a higher concavity with respect including developped ones.1 In such a case, the estimator is to the variable, and also of a higher variability (whatever its extemely sensitive to "small" samples, "small" meaning in measurement) of the variable conditionally to being above practice 108. We ran up to a trillion simulations across varieties the threshold than to being below. The fatter the right tail of the distribution, the stronger the effect. Overall, we find of sample sizes. While κ0:01 ≈ 0:657933, even a sample size E [Ah(n)] of 100 million remains severely biased as seen in the table. that [κ (n)] ≤ = κ (note that unfreezing the E bh [S(n)] h Naturally the bias is rapidly (and nonlinearly) reduced for α E threshold h^(q) also tends to reduce the concentration measure further away from 1, and becomes weak in the neighborhood estimate, adding to the effect, when introducing one extra of 2 for a constant α, though not under a mixture distribution sample because of a slight increase in the expected value of for α, as we shall se later. It is also weaker outside the top the estimator h^(q), although this effect is rather negligible). 1% centile, hence this discussion focuses on the famed "one We have in fact the following: percent" and on low values of the α exponent. n Proposition 1. Let X = (X)i=1 a random sample of size TABLE I: Biases of Estimator of κ = 0:657933 From 1012 1 n > q , Y = Xn+1 an extra single random observation, and Monte Carlo Realizations Pn 1 X + 1 Y define: κ (X t Y ) = i=1 Xi>h i Y >h . We remark κ(n) Mean Median STD bh Pn b i=1 Xi + Y across MC runs that, whenever Y > h, one has: κ(103) 0.405235 0.367698 0.160244 b 4 κb(10 ) 0.485916 0.458449 0.117917 5 2 κb(10 ) 0.539028 0.516415 0.0931362 @ κh(X t Y ) 6 b ≤ 0: κb(10 ) 0.581384 0.555997 0.0853593 2 κ(107) 0.591506 0.575262 0.0601528 @Y b 8 κb(10 ) 0.606513 0.593667 0.0461397 ^ This inequality is still valid with κbq as the value h(q; X t Y ) doesn’t depend on the particular value of Y > h^(q; X): In view of these results and of a number of tests we have performed around them, we can conjecture that the bias κq − We face a different situation from the common small sample −b(q)(α−1) κbq(n) is "of the order of" c(α; q)n where constants effect resulting from high impact from the rare observation b(q) and c(α; q) need to be evaluated. Simulations suggest that in the tails that are less likely to show up in small samples, b(q) = 1; whatever the value of α and q, but the rather slow a bias which goes away by repetition of sample runs.
Details
-
File Typepdf
-
Upload Time-
-
Content LanguagesEnglish
-
Upload UserAnonymous/Not logged-in
-
File Pages6 Page
-
File Size-