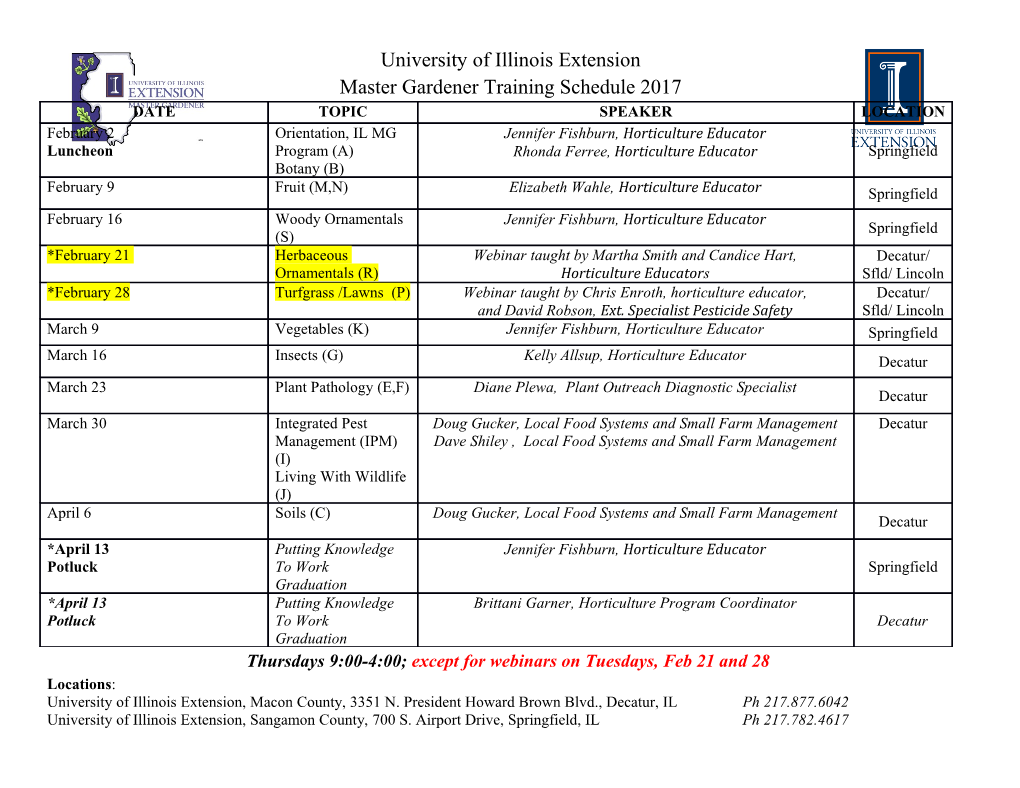
To Kavanaugh or Not to Kavanaugh: That is the Polarizing Question Kareem Darwish Qatar Computing Research Institute, HBKU Doha, Qatar [email protected] Abstract tice Anthony Kennedy2. His nomination was marred by con- troversy with Democrats complaining that the White House On October 6, 2018, the US Senate confirmed Brett Ka- withheld documents pertaining to BK’s record and later a vanaugh with the narrowest margin for a successful confir- few women including a University of California profes- mation since 1881 and where the senators voted overwhelm- sor accused him of sexual assault. The accusations of sex- ingly along party lines. In this paper, we examine whether ual misconducted led to a public congressional hearing on the political polarization in the Senate is reflected among the general public. To do so, we analyze the views of more than September 27, 2018 and a subsequent investigation by the 128 thousand Twitter users. We show that users supporting or Federal Bureau of Investigation (FBI). The US Senate voted opposing Kavanaugh’s nomination were generally using di- to confirm BK to a seat on the Supreme Court on October vergent hashtags, retweeting different Twitter accounts, and 6 with a 50–48 vote, which mostly aligned with party loyal- sharing links from different websites. We also examine char- ties. BK was sworn in later the same day. acterestics of both groups. Data Collection We collected tweets pertaining to the nomination of BK in Introduction two different time epochs, namely September 28-30, which On October 6, 2018, the US senate confirmed Brett Ka- were the three days following the congressional hearing con- vanaugh to become a justice on the US Supreme Court cerning the sexual assault allegation against BK, and Octo- with a 50 to 48 vote that was mostly along party lines. ber 6-9, which included the day the Senate voted to confirm This was the closest successful confirmation since the Stan- BK and the following three days. We collected tweets using 3 ley Matthews confirmation in 18811. In this paper, we ex- the twarc toolkit , where we used both the search and fil- amine whether the political polarization at play in the US tering interfaces to find tweets containing any of the follow- Senate between Republicans, who overwhelmingly voted ing keywords: Kavanaugh, Ford, Supreme, judiciary, Blasey, for Kavanaugh, and Democrats, who overwhelmingly voted Grassley, Hatch, Graham, Cornyn, Lee, Cruz, Sasse, Flake, against him, reflects polarization among the general pub- Crapo, Tillis, Kennedy, Feinstein, Leahy, Durbin, White- lic. We analyze more than 23 million tweets related to the house, Klobuchar, Coons, Blumenthal, Hirono, Booker, or Kavanaugh confirmation. Initially, we semi-automatically Harris. The keywords include the BK’s name, his main ac- tag more than 128 thousand Twitter users as supporting cuser, and the names of the members of the Senate’s Judi- or opposing his confirmation. Next, we bucket hashtags, ciary Committee. The per day breakdown of the collected retweeted accounts, and cited websites according to how tweets is as follows: strongly they are associated with those who support or op- Date Count arXiv:1810.06687v1 [cs.SI] 15 Oct 2018 pose. Further, we visualize users according to their similarity 28-Sep 5,961,549 based on their hashtag usage, retweeted accounts, and cited 29-Sep 4,815,160 websites. All our analysis show strong polarization between 30-Sep 1,590,522 both camps. Lastly, we highlight some of the main differ- subtotal 12,367,231 ences between both groups. 6-Oct 2,952,581 7-Oct 3,448,315 Timeline 8-Oct 2,761,036 9-Oct 1,687,433 On July 9, 2018, Brett Kavanaugh (BK), a US federal judge, subtotal 10,849,365 was nominated by the US president Donald Trump to serve Total 23,216,596 as a justice on the US supreme court to replace outgoing Jus- 2https://en.wikipedia.org/wiki/Brett_ 1https://www.senate.gov/pagelayout/ Kavanaugh reference/nominations/Nominations.htm 3https://github.com/edsu/twarc In the process we collected 23 million tweets that were au- Data Analysis thored by 687,194 users. Our first step was to label as many Next we analyzed the data to ascertain the differences in in- users as possible by their stance as supporting (SUPP) or op- terests and focus between both groups as expressed using posing (OPP) the confirmation of BK. The labeling process three elements, namely the hashtags that they use, the ac- was done in three steps, namely: counts they retweet, and the websites that they cite (share content from). Doing so can provide valuable insights into • Manual labeling of users. We manually labeled 43 users both groups (Darwish et al. 2017b; Darwish, Magdy, and who had the most number of tweets in our collection. Of Zanouda 2017). For all three elements, we bucketed them them, the SUPP users were 29 compared to 12 OPP users. into five bins reflecting how strongly they are associated The two remaining users were either neutral or spammers. with the SUPP and OPP groups. These bins are: strong SUPP, SUPP, Neutral, OPP, and strong OPP. To perform the • Label propagation. Label propagation automatically la- bucketing, we used the so-called valence score (Conover et bels users based on their retweet behavior (Darwish et al. al. 2011). The valence score for an element e is computed as 2017b; Kutlu, Darwish, and Elsayed 2018; Magdy et al. follows: 2016). The intuition behind this method is that users that retweet the same tweets on a topic most likely share the tfSUP P same stance. Given that many of the tweets in our collec- V (e) = 2 totalSUP P − 1 (1) tfSUP P + tfOP P tion were actually retweets or duplicates of other tweets, totalSUP P totalOP P we labeled users who retweeted 15 or more tweets that where tf is the frequency of the element in either the SUPP were authored or retweeted by the SUPP group or 7 or or OPP tweets and total is the sum of all tfs for either the more times by OPP group and no retweets from the other SUPP or OPP tweets. We accounted for all elements that side as SUPP or OPP respectively. We elected to increase appeared in at least 100 tweets. Since the value of valence the minimum number for the SUPP group as they were varies between -1 (strong OPP) to +1 (strong SUPP), we over represented in the initial manually labeled set. We it- divided the ranged into 5 equal bins: strong OPP [-1.0 – - eratively performed such label propagation 4 times, which 0.6), OPP [-0.6 – -0.2), Neutral [-0.2 – 0.2), SUPP [0.2 – is when label propagation stopped labeling new accounts. 0.6), and strong SUPP [0.6 – 1.0). After the last iteration, we were able to label 65,917 users Figures 1, 2, and 3 respectively provide the number of dif- of which 26,812 were SUPP and 39,105 were OPP. Since ferent hashtags, retweeted accounts, and cited websites that we don’t have golden labels to compare against, we opted appear for all five bins along with the number of tweets in to spot check the results. Thus, we randomly selected which they are used. As the figures show, there is strong po- 10 automatically labeled accounts, and all of them were larization between both camps. Polarization is most evident labeled correctly. This is intended as a sanity check. A in the accounts that they retweet and the websites that they larger random sample is required for a more thorough share content from, where “strong SUPP” and “strong OPP” evaluation. Further, this labeling methodology naturally groups dominate in terms of the number of elements and favors users who actively discuss a topic and who are their frequency. likely to hold strong views. Tables 1, 2, and 3 respectively show the 15 most com- monly used hashtags, retweeted accounts, and most cited • Retweet based-classification. We used the labeled users websites for each of the valence bins. Since the “Strong to train a classification model to guess the stances of users SUPP” and “strong OPP” groups are most dominant, we fo- who retweeted at least 20 different accounts, which were cus here on their main characteristics. users who were actively tweeting about the topic. For For the “Strong SUPP” group, the hashtags can be split classification, we used the FastText classification toolkit, into the following topics (in order of importance as deter- which is an efficient deep neural network classifier that mined by frequency): has been shown to be effective for text classification (Joulin et al. 2016). We used the accounts that each user • Trump related: #MAGA (Make America Great Again), retweeted as features. Strictly using the retweeted ac- #Winning. counts has been shown to be effective for stance classi- • Pro Kavanaugh confirmation: #ConfirmKavanaugh, fication (Magdy et al. 2016). To keep precision high, we #ConfirmKavanaughNow, #JusticeKavanaugh. only trusted the classification of users where the classifier was more than 90% confident. Doing so, we increased • Anti-Democratic Party: #walkAway (campaign to walk- the number of labeled users to 128,096, where 57,118 away from liberalism), #Democrats, #Feinstein belonged to the SUPP group with 13,095,422 tweets • Conspiracy theories: #QAnon (an alleged Trump admin- and 70,978 belonged to the OPP group with 12,510,134 istration leaker), #WWG1WGA (Where We Go One We tweets. Again, we manually inspected 10 random users Go All) who were automatically tagged and all of them were clas- sified correctly. It is noteworthy that the relative number • Midterm elections: #TXSen (Texas senator Ted Cruz), of SUPP to OPP users in not necessarily meaningful.
Details
-
File Typepdf
-
Upload Time-
-
Content LanguagesEnglish
-
Upload UserAnonymous/Not logged-in
-
File Pages8 Page
-
File Size-