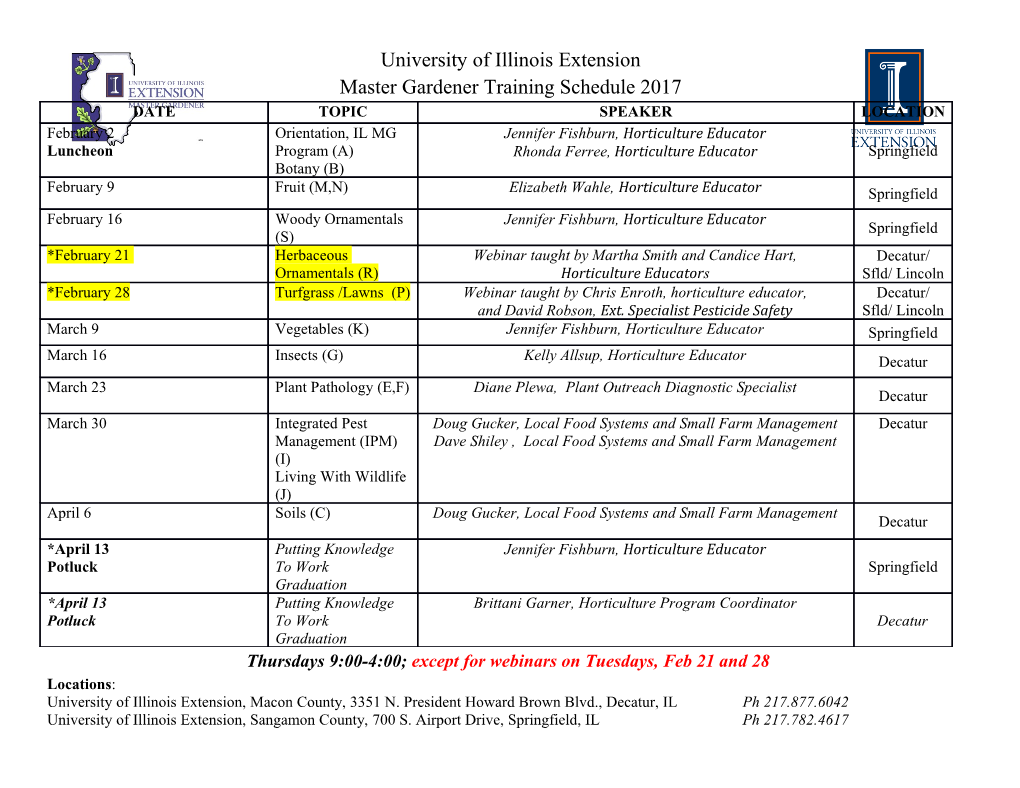
Magnetic Resonance Imaging 32 (2014) 736–746 Contents lists available at ScienceDirect Magnetic Resonance Imaging journal homepage: www.mrijournal.com A novel approach for fMRI data analysis based on the combination of sparse approximation and affinity propagation clustering Tianlong Ren, Weiming Zeng ⁎, Nizhuan Wang, Lei Chen, Chenglin Wang Digital Image and Intelligent computation Laboratory, College of Information Engineering, Shanghai Maritime University, Shanghai 201306, China article info abstract Article history: Clustering analysis has been widely used to detect the functional connectivity from functional magnetic Received 24 August 2013 resonance imaging (fMRI) data. However, it has some limitations such as enormous computer memory Revised 16 February 2014 requirement, and difficulty in estimating the number of clusters. In this study, in order to effectually Accepted 17 February 2014 resolve the deficiencies mentioned above, we have proposed a novel approach (SAAPC) for fMRI data analysis, which combines sparsity, an effective assumption for analyzing fMRI signal, with affinity Keywords: propagation clustering (APC). Sparse approximation fi fMRI The SAAPC method is composed of three parts: to obtain the sparse approximation coef cients set through Affinity propagation clustering wavelet packet decomposition and sparsity measuring and selection, which contributes a lot in the brain APC functional connectivity detection accuracy; to implement a split APC algorithm, which is put forward in this paper to overcome the computer memory shortage problem and to reduce the time cost in basic APC; to reconstruct the source signal by unmixing the mixed fMRI data using the time courses which are derived from the ultimate exemplars. In the task-related experiments, we can see that SAAPC is more accurate to detect the functional networks than basic APC, and it significantly reduces the time cost relative to basic APC. In addition, in the resting- state data experiments, the SAAPC method can successfully identify typical resting-state networks from the resting-state data set, while this performance is seldom reported by the classical cluster method and the basic APC method. This proposed clustering analysis method is expected to have wide applicability. © 2014 Elsevier Inc. All rights reserved. 1. Introduction independent components (ICA), but the meaningful decomposed components are hard to choose. Fuzzy clustering analysis (FCA) The blood oxygenation level dependent (BOLD) based function [18], k-centers clustering analysis [19,20] and hierarchical clus- magnetic resonance imaging (fMRI) is a powerful modality in the tering analysis (HCA) [21] are based on clustering analysis, and study of detecting the brain functional connectivity which is defined as their main drawback is that their reliability can only be established the “temporal correlations between spatially remote neurophysiolog- with repeated runs. ical events” [1,2]. Many researchers have already studied this kind of Recently, a novel data-driven method called affinity propagation connectivity through experimental approaches [3–7]. As the fMRI clustering (APC) has been proposed [22]. This algorithm simulta- technology draws attention from researchers in the field of computer neously considers all data points as potential exemplars, and science recently, more and more numerical methods and models from exchanges messages between data points until a good set of statistics, pattern recognition and signal processing, have been exemplars and clusters emerge. APC algorithm has been found to successfully applied to fMRI data analysis. perform well in clustering images of faces, identifying representative Data-driven methods [8] for detecting the brain functional sentences, and detecting genes. In addition, unlike the k-centers connectivity include two kinds: decomposition technique based clustering which is sensitive to the initial selection of exemplars methods and clustering based methods. The decomposition technique [19,20], in APC algorithm, the number of clusters (NC) need not be based methods, like principal component analysis (PCA) [9,10], pre-specified because of the influence of the input preference values. singular value decomposition (SVD) [11] and independent component Zhang et al. [23,24] has successfully applied APC algorithm to analysis (ICA) [12–17], try to express the original fMRI dataset as a detecting functional connectivity on task-related fMRI data. How- linear combination of basis vectors (PCA/SVD) or statistically ever, the intrinsic functional connectivity has not yet been revealed using the basic APC algorithm. ⁎ Corresponding author. Tel.: + 86 21 3828 2873; fax: +86 21 3828 2873. The assumption of sparisity has demonstrated that it can E-mail address: [email protected] (W. Zeng). significantly enhance signal separation accuracy and computation http://dx.doi.org/10.1016/j.mri.2014.02.023 0730-725X/© 2014 Elsevier Inc. All rights reserved. T. Ren et al. / Magnetic Resonance Imaging 32 (2014) 736–746 737 efficiency of existing ICA methods on MEG data [25]. In terms of fMRI (3) And the availabilities are computed using the rules: data, the sparse property is also a general assumption, and many 8 9 scholars have researched on it to help decode the functional < X ÈÉÀÁ= ; ← ; ; ; 0; connectivity among the cortical regions of the human brain. It has aiðÞk min: 0 rkðÞþk max 0 ri k ; ð3Þ 0 : : 0∉ ; been demonstrated that the optimal sparse representation is i s t i fgi k significantly beneficial to the de-noising of fMRI time courses [26]. X ÈÉÀÁ ; ← ; 0; Flandin and Penny [27] have proposed a kind of Bayesian fMRI data akðÞk max 0 ri k ð4Þ 0 : : 0≠ analysis with sparse spatial basis functions, which firstly projected i s t i k the original to wavelet space, followed by threshing the small (4) Finally, assignments are made: coefficients to make the projected-back data sparser. Sparse component analysis (SCA) introduced by Georgiev et al. [28] can ← ; ; ci arg maxfgriðÞk þ aiðÞk ð5Þ reveal a stronger potential ability of dependent fMRI sources k separation compared with ICA. Ye et al. [29] have put forward a data-driven sparse geo-statistical analysis in clustering method and In the basic APC, another important parameter, damping factor gained better performance compared with GLM analysis. Sparse λ ∈ (0,1), is designed to avoid numerical oscillations that arise in approximation technique is based on the sparse property of source some circumstances. During the iterations, the responsibility and signal, which can be used to obtain a dataset with less redundancy availability are updated with the value produced in the and improve the separation accuracy of source signal. Wang et al. previous iteration: [30] have introduced an effective sparse approximation coefficient- based ICA (SACICA) model which performed better than FastICA. All −λ λ ri ¼ ðÞÂ1 ri þ Â ri−1 the studies mentioned above demonstrated that the sparsity of the −λ λ ð6Þ ai ¼ ðÞÂ1 ai þ Â ai−1 fMRI signal is a useful feature for source separation. In this study, a novel approach for fMRI data analysis based on the The whole procedure of basic APC may be terminated after a fixed fi combination of sparse approximation and af nity propagation clustering number of iterations, after changes in the messages fall below a (SAAPC)isproposed.Theremainder of this paper is organized as threshold, or after the local decisions stay constant for some number fi follows: rstly, the theory and method associated with SAAPC will be of iterations. presented; and then, the task-related data experiments on testing the However, the basic APC has two limitations: oscillations cannot performance of SAAPC will be showed, followed by the resting-state be eliminated automatically when they occur, and it is hard to fi network detection experiment on resting-state data set; nally, some estimate the value of parameter p which will influence the producing discussions related to the advantages and limitations of this new method of optimal clustering outcomes. An adaptive APC proposed by Wang on analyzing fMRI data will be presented. et al. [31] which assumes λstep and pstep are the adaptive factors, has the ability to well overcome these limitations. The values of adaptive 2. Theory and method factors can be determined empirically. Assuming that Num(i) is the number of clusters during the iterations and Num is the expected fi min 2.1. Af nity propagation clustering (APC) minimal number of clusters. Then, the steps of adaptive APC are presented as follows: The specific framework of basic APC [22] is presented in the following: consider Y ={y1,y2,⋯,yN} as the set of data points to be (1) Execute basic APC procedure and get Num(i); λ ← λ λ clustered into different types. The basic APC takes as input a (2) If there exists numerical oscillations, then + step, else collection of real-valued similarities between data points of Y, where execute basic APC continually; ≤ ← ← the similarity s(i, k)isdefined as a negative value. (3) If Num(i) Num(i + 1), then p p + pstep, and s(i, i) p; ≤ Note that, when k = i, the s(k, k), which is referred to as (4) If i = max iteration or Num(i) Nummin, then the algorithm “preference (denoted as p)”, is often set as a constant by hand. The terminates. Else, go to step (2). preferences p are important parameters in basic APC, which influence The value ofpNumffiffiffiffiffiffiffiffiffiffiffiffiffiffiffiffiffiffiffiffimin can be determined empirically, and pstep is the final number of clusters. When p are larger, the number of set to 0:1×p = KiðÞþ50; where p denotes the median
Details
-
File Typepdf
-
Upload Time-
-
Content LanguagesEnglish
-
Upload UserAnonymous/Not logged-in
-
File Pages11 Page
-
File Size-