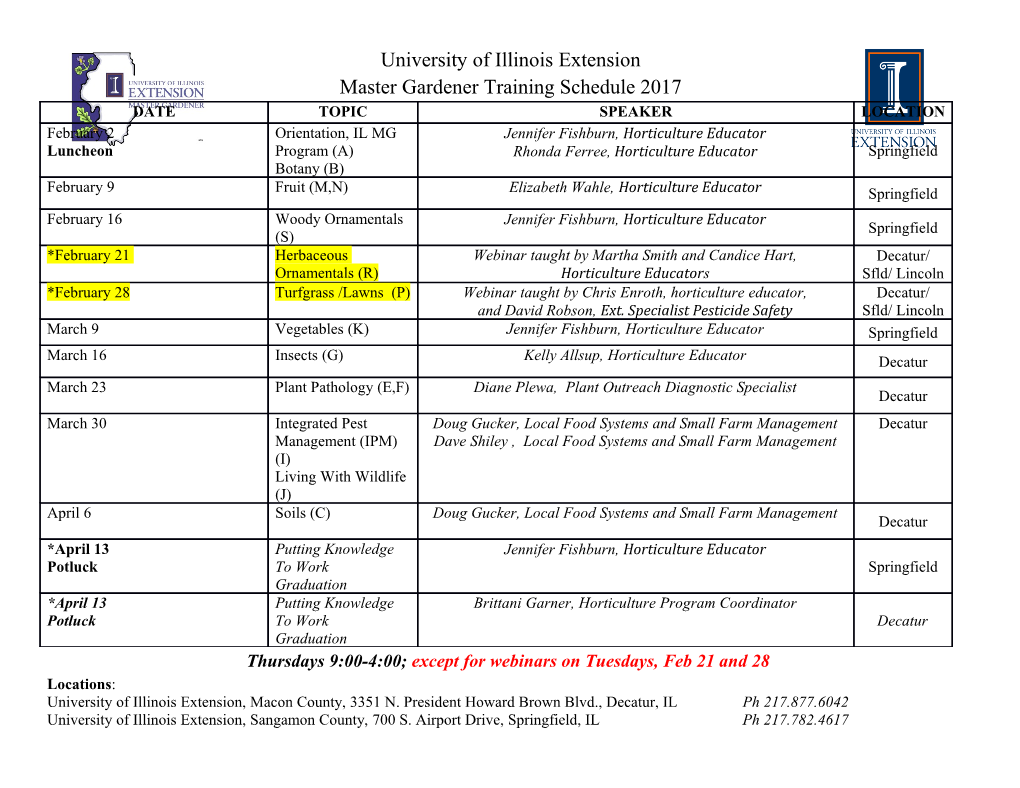
Journal of Sports Analytics 4 (2018) 121–131 121 DOI 10.3233/JSA-170225 IOS Press A weighted plus/minus metric for individual soccer player performance Steven R. Schultzea and Christian-Mathias Wellbrockb,∗ aUniversity of South Alabama, Mobile, AL, USA bUniversity of Cologne, Cologne, Germany Abstract. Despite soccer being the number one sport in the world in many respects, the “beautiful game” still lags behind other sports in terms of analytics. We propose a weighted plus/minus metric to be used as an instrument to evaluate player performance. An unweighted plus/minus metric subtracts goals conceded from goals scored for each player while on the field of play and are regularly used in hockey or basketball. Key improvements to this established, unweighted +/– metric include control for opponents’ strength, the importance of a particular goal, and considerations for the fact that scoring is less frequent in soccer. The results from three teams (Bayern Munich, VfL Wolfsburg, Werder Bremen) in the German Bundesliga from the 2012-13 season were used as a demonstration and comparison of the two metrics. In addition to the creation of a weighted +/– system, a spatial mapping system of team shots was developed to give a potential visual explanation of why certain players were a net positive/negative influence for their team. Keywords: Soccer, football, analytics, plus/minus, spatial analysis in football 1. Introduction statistics in the field of soccer beyond basic statis- tics such as goals, assists or tackles. As such, the As analytics continue to grow in nearly all sports, +/– system adds a quantitative insight into a player’s it is surprising that soccer, the number one sport in the performance which could improve coaches’ and man- world, lags behind. Although soccer analytics are an ager’s decisions both in the training and scouting emerging field, they have not yet reached the levels realms of the game. It also extends +/– metrics in to which baseball or basketball have assimilated such other sports in two ways: (1) by controlling for the analysis on the level of Sabermetrics (James, 1982) importance of goals (a go-ahead goal, for instance, or Goldsberry’s Courtvision (Goldsberry, 2012). We should be more valuable than a goal that makes it 5-0) introduce a +/– system to evaluate player perfor- and (2) by controlling for opponents’ strength (scor- mance, a task considered to be difficult due to the ing against a heavy favorite is harder than against game’s complex nature. In one example, a team’s a weak opponent). Examples of positive and nega- success may be linked to a certain player’s perfor- tive influences will be displayed in the results and mance over the course of a season. The +/– system discussion sections of the paper. would show whether a player’s presence on the field Analytics in soccer have been utilized before, but is a net positive or negative for his team, regardless typically in a more general theme. Anderson and of position. It allows for the comparison of a player’s Sally’s 2013 book examined statistics on a large scale net influence on team performance with other team- by responding to questions like “does possession mat- mates. This approach is a significant advancement in ter?” and asks questions such as what role total team salaries or coaches have on a team’s success (Ander- ∗ son & Sally, 2013). It also summarized several other Corresponding author: Christian-Mathias Wellbrock, Univer- papers from previous decades starting with Britain’s sity of Cologne, Pohligstr. 1, 50969 Cologne, Germany. Tel.: +49 221 470 5367; E-mail: [email protected]. Charles Reep in the middle of the 20th Century (Reep 2215-020X/18/$35.00 © 2018 – IOS Press and the authors. All rights reserved This article is published online with Open Access and distributed under the terms of the Creative Commons Attribution Non-Commercial License (CC BY-NC 4.0). 122 S.R. Schultze and C.-M. Wellbrock / A weighted plus/minus metric & Benjamin, 1968). In terms of more recent research, +/– metric, highlighting three teams (Bayern Munich, there have been advances from Ian McHale, where in VfL Wolfsburg and Werder Bremen) with very dif- 2007, McHale and Scarf developed a match predic- ferent end season outcomes in the 2012-13 German tion model, and in McHale et al. (2012), a player Bundesliga season. evaluation system was developed for the Barclay’s Premier League using several sub-indices. While that approach uses a variety of individual player perfor- 2. Methodology mance data (such as goals scored, shots, assists, clean sheets), the +/– metric we introduce focuses on the Plus/minus metrics are well established, especially one most crucial team performance measure – goals in hockey (Macdonald, 2011), but are also used to – and uses it to determine individual contribution evaluate player performance in other team sports, e.g. to team success. From a team perspective, Ober- basketball (Sill, 2010). The idea is simple: whenever stone (2009) performed regression analysis on team’s the team scores, all players on the court at the time of actual vs. model predicted season success rates in the the event receive positive points. Whenever the team Barclay’s Premier League. The 2014 Sloan Sports is scored against, the players who are currently play- Analytics conference, the United States’ foremost ing receive negative points. Combining the negative meeting on sports analytics, featured one paper on and positive values over time yields the “plus/minus” formation analysis that confirmed the old adage that statistic. Consequently, players who have positive teams play for the draw on the road and play for the scores in this metric were on the court when the team win at home (Bialkowski et al., 2014). performed well (scored more often than the oppo- We also introduce a spatial mapping system of nents), while players with negative values were on events to visualize the “how” and “why” a player’s net the court when their team was outscored by the oppo- influence is positive or negative. In terms of spatial nents. The plus/minus metric implicitly assumes that analysis in soccer, there has been very little literature all players on the pitch/court contribute to the team’s on the matter. There have been studies on the spa- success to the same extent. In sports with frequent for- tiality of socio-economic impacts of soccer (Forrest mation changes, this metric can also be interpreted et al., 2002) and studies on media and spatial events as an individual player’s contribution to the team’s in soccer (Yow et al., 1995; Ekin, 2003). In terms of success. specific spatial statistics of events on the pitch, studies The simplest version of the metric (subtracting have been limited in scope (Kim et al., 2011). McHale points/goals against from points/goals for) also treats and Szczepanski´ (2014) performed a statistical and all scoring events equally. A strong objection against spatial based mixed effect model for predicting goal this assumption is that scores in already decided scorer success. However, this paper was focused more games (in “garbage time”) are less valuable than on direct prediction of events. It only addresses goal scores in tight games or in “crunch time”. Another scoring ability of footballers, which without a doubt objection is that winning against weak opponents is is an important skill, but leaves out other important not as valuable as winning (or tying) against heavy features such as the avoidance of goals against. By favorites. The simple version of the plus/minus metric using quantitative methods that are backed up by does not account for either of the two concerns. spatial visualizations to evaluate player performance, While in-game formation changes are not as fre- one can understand a player’s net effect on a team’s quent in soccer as they are in hockey or basketball success. (mainly because substitutions are limited to three per We first summarize the methods behind the cre- game), they do occur more often between games due ation of the +/– metric, in which the justification and to injuries, suspensions, and coach’s decisions. Scor- mathematics will be presented. This is followed by a ing events also occur less frequently in soccer, but section including the results of the plus-minus met- often enough to assume acceptable levels of statisti- ric for the case studies used in this paper. We then cal validity (around 100 events per team per season on present a discussion of the results, including visu- average). What makes this plus/minus metric unique alizations of potential reasons why certain players is that it addresses the two objections mentioned are net positives or negatives on their teams. This is above by controlling for goal importance and for rel- followed by a brief conclusion considering the impli- ative opponent’s strength. The +/– metric for Player x cations of advanced analytics in soccer. The main for the entire season is calculated with the following objective of this paper is to display the utility of the formula: S.R. Schultze and C.-M. Wellbrock / A weighted plus/minus metric 123 pmx(t, w) = that happened recently that might affect the game. W T There has been quite extensive research on the effec- wp opp − wp own w w tiveness of league football betting markets in the Tw w=1 t=1 sense that betting odds reflect the available informa- tion (e.g. Croxson & Reade, 2014; Deschamps & Ger- + goalst,w − goalst−1,w gaud, 2007; Dobson & Goddard, 2011; Forrest et al., 2 2005; Goddard, 2005; Goddard & Asimakopoulos, × × x onw,t (1) 1 +|goalst,w|+|goalst−1,w| 2004; Spann & Skiera, 2009) and consequently on the quality of the information when using betting quotas as a proxy for team strength.
Details
-
File Typepdf
-
Upload Time-
-
Content LanguagesEnglish
-
Upload UserAnonymous/Not logged-in
-
File Pages11 Page
-
File Size-