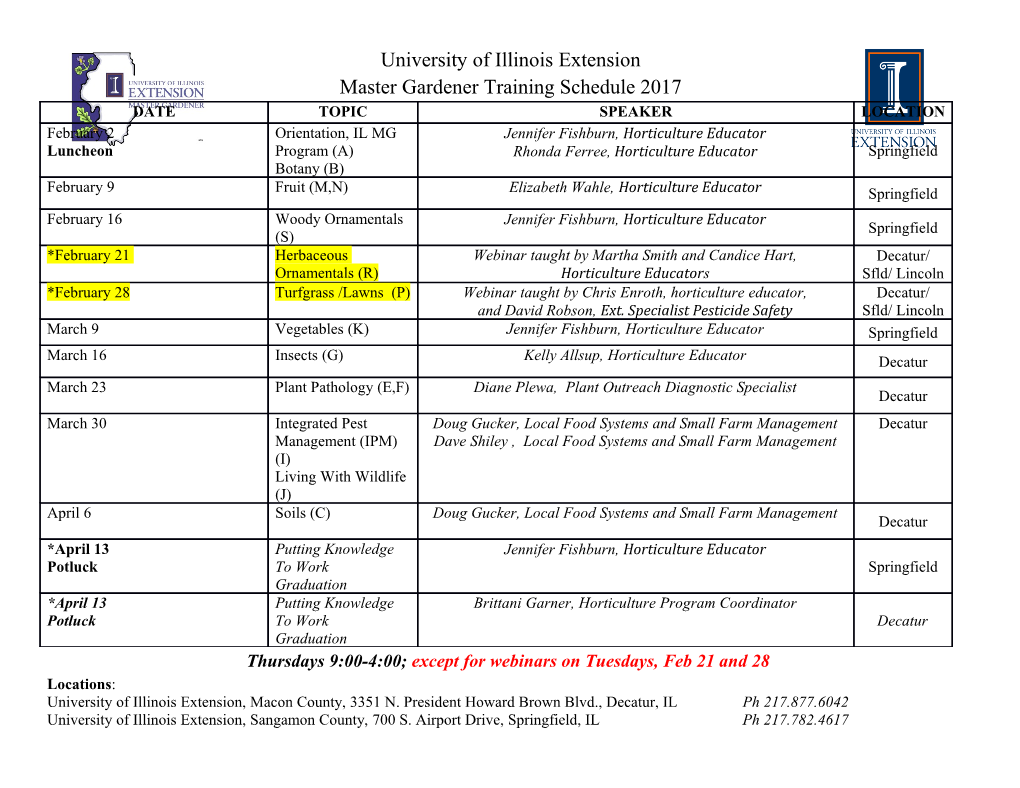
FUNDAMENTALS OF MEDICAL RESEARCH Ed J. Gracely, Ph.D. Family, Community, and Preventive Medicine Multivariate statistics July 16, 2014 Handout 4 of 4 Goals: After this session, you should be able to: 1. Explain the concept of “holding a variable constant” to identify independent associations. 2. Explain how multivariate techniques help identify independent associations. 3. Interpret the results of a multivariate analysis. 4. Describe the chief limitations with multivariate techniques. A) The concept of holding variables constant Ex: An agricultural researcher who is trying to see the effect of amount of fertilizer on plant growth will make sure that different fertilizer quantities are compared between groups of plants that have the same amount of water, exposure to sunshine, soil conditions, and temperature. In this way, because the other potential influences on growth are held constant, the effect of the fertilizer can directly be observed. Ex: A medical researcher testing the benefits of treatment A vs. treatment B will often randomly assign subjects to groups. The assignment at random will not make every subject the same on other relevant variables but should make the two groups similar. For example, they should, on the average, end up similar in mean age, mean severity, percent male and female, etc... Thus there should be no important differences between the groups that could influence the results other than the treatment given to each group. This is a basic principle of science: To see the effect of one variable, hold constant or make groups comparable on (as much as possible) the other variables that could affect the outcome. In the above examples the researcher is manipulating conditions directly. This kind of research is the most powerful, when it can be done. The techniques in this session are mainly applicable to designs in which direct manipulation and assignment of independent variables is not possible. Ex: A researcher is studying children 4 - 8 years old. The researcher finds a highly significant association between height and skill on a task, p < 0.001. In truth, height has nothing to do with ability on this task. Q: Why might there be an association between height and skill in these children? a Q: If the researcher looks at children who are all exactly the same age (4 years, 6 months, for example) would you expect to see a relationship between height and skill? Vote: Y /N. b 1 Q: If the researcher looks only at the children who are exactly 3 feet tall, would you expect to see an association between AGE and ability? Vote: Y/N c Key point: In observational research, where manipulation and assignment to conditions are not possible, one important way to "hold constant" variables is by selection of subjects. If I want to study the effect of height on a skill task, I would try to get subjects who were the same age, sex, and perhaps weight and even intelligence level, while varying in height. Then I could see the effect of height un-confounded by those other variables. A variable that predicts the outcome when considered alone, but no longer predicts when other variables are held constant, probably has little or no independent effect on the outcome. Ex: A researcher finds that compliance with medical recommendations is higher among people who have a higher income and concludes that having money is conducive to following recommendations. Suppose that in truth, income has no direct effect on compliance. Income is merely indicating level of education which in turn indicates understanding of the recommendations. Q: If the supposition is true, and if the researcher carefully tested everyone's understanding of the regimen and the need for it, then found a group of subjects who all fully understood, would there still be differences in compliance due to income differences within that well-informed subgroup? Vote: Y / N. d Q: Suppose a group of subjects was selected who were all at the same income level. Would level of understanding still predict compliance even in this group? Vote: Y / N. e Why is this important in a multivariate class? Because multivariate statistics control variables too, in ways that have logical similarities to what we did above. These techniques are said to statistically "control" variables or "hold them constant". The difference is that multivariate statistics do this mathematically, without actually requiring the researcher to restrict levels of the variables being measured. B) Some important terms 1) Multivariate: a wide variety of different techniques, difficult to categorize. Virtually all techniques that incorporate several variables simultaneously in the analysis are considered multivariate, except for analysis of variance. These techniques are used to statistically control variables, as mentioned above. Many of them also produce sophisticated prediction equations that can be used to combine several variables in predicting an outcome. 2) Univariate: Other designs. t-tests, all ordinary ANOVAs, almost all non-parametric tests, simple linear correlation/regression etc... 2 Three common techniques used for controlling variables 1) Multiple linear regression: Predicting a numeric variable from two or more predictor variables. This provides a regression (prediction) equation with a multiplier ("regression weight") for each of the predictors. Severity = Duration x 2.5 + Age x 1.3 + Initial severity x 0.8 + 2, for example. 2) Logistic regression: a method employed when the dependent variable has exactly 2 levels, such as yes/no, success/failure, live/die. The primary measure of association is an odds ratio. 3) Proportional hazards (Cox Model): a method used when the dependent variable is time till some event occurs (often, but not always, death) especially when not all subjects in the study reach the endpoint. The event can be good (for example release from the OR) or bad (death, relapse). The time variable can be a true time (days, years) or some other measure of process elapsed (number of tries). They key is that some subjects get a true time of event whereas others ("censored observations") had not yet experienced the event when last seen. Survival curves, Kaplan-Meier curves for example, are a univariate aspect of the same kind of data. The primary measure of association is a hazard ratio, interpreted mostly like a relative risk. These three regression-type techniques are all interpreted in the same way. The effect of each variable is reported with all other variables controlled (statistically "held constant"). They also all give equations that can be used to *predict* the outcome variable from the predictors. Thus, a variable that is significant (p < 0.05) as a predictor in a multivariate analysis may be considered to have an association with the dependent variable that is not due to its associations with the other predictors. Q: Does this prediction independent of the other (included) variables prove that the significant predictor is a cause of the dependent variable? f Key point: When several predictors of an outcome are used together in a multivariate analysis, the p values (and other statistics) for each predictor are calculated as if all of the other predictors were constant. Thus the effect of each variable is reported in a way that is independent of its associations with the other variables. A variable that is statistically significant as a predictor in a multivariate analysis may be considered as having an association with the outcome, that is NOT dependent on any of the other variables. It is a candidate for being a cause of the outcome (although this cannot be proven by statistical analyses of this sort). A variable that is significant univariate, but NOT significant multivariate, is probably only predictive because of its associations with other predictors. Ex: Using the height and skill example, a researcher reports: "When placed into a multivariate analysis, specifically a (because the dependent variable was numeric), age was predictive (p < 0.001), while height was not (p = 0.75). This indicates that (choose all that apply): g 3 a. When height was held constant, age still predicted significantly. b. When height was held constant, age no longer predicted significantly. c. When age was held constant, height still predicted significantly. d. When age was held constant, height no longer predicted significantly. e. Age appears to be predicting independently of height. f. Height appears to be predicting independently of age. Ex Orchard TJ. et al., Diabetes Research & Clinical Practice. 34 Suppl: S 165-71, 1996 Oct. studied predictors of and outcomes of diabetic autonomic neuropathy (DAN). For predicting 2-year mortality they say: "Mortality was increased four-fold in those with DAN (P = 0.005), although this difference no longer was significant after adjustment for baseline nephropathy or hypertension." h Q: The DAN and mortality relationship is best described as: a. DAN is predicting completely independently of the other variables studied. b. DAN predicts largely because it is a marker for other serious conditions. c. The authors should rely on the univariate results, which are more significant. Q: Your patient, who has DAN but not nephropathy or hypertension, has read that DAN patients have a substantially elevated mortality, even over the next few years. He asks whether he should be concerned about his short-term mortality risk. You reply, based on the above: i a. Yes, sadly, you should be. Your DAN puts you at significantly elevated risk of early mortality. b. No, in the short term, increased mortality in DAN patients is mainly related to their also having other dangerous conditions, which you don't. For predicting DAN, they say: "Duration of diabetes, the cardiovascular risk profile (hypertension, elevated LDL cholesterol and triglycerides), and other complications (e.g. nephropathy) were all univariately associated with subsequent DAN (P < 0.01). Smoking status and hemoglobin A1 (HbA1) were less strongly, related (P < 0.05).
Details
-
File Typepdf
-
Upload Time-
-
Content LanguagesEnglish
-
Upload UserAnonymous/Not logged-in
-
File Pages8 Page
-
File Size-