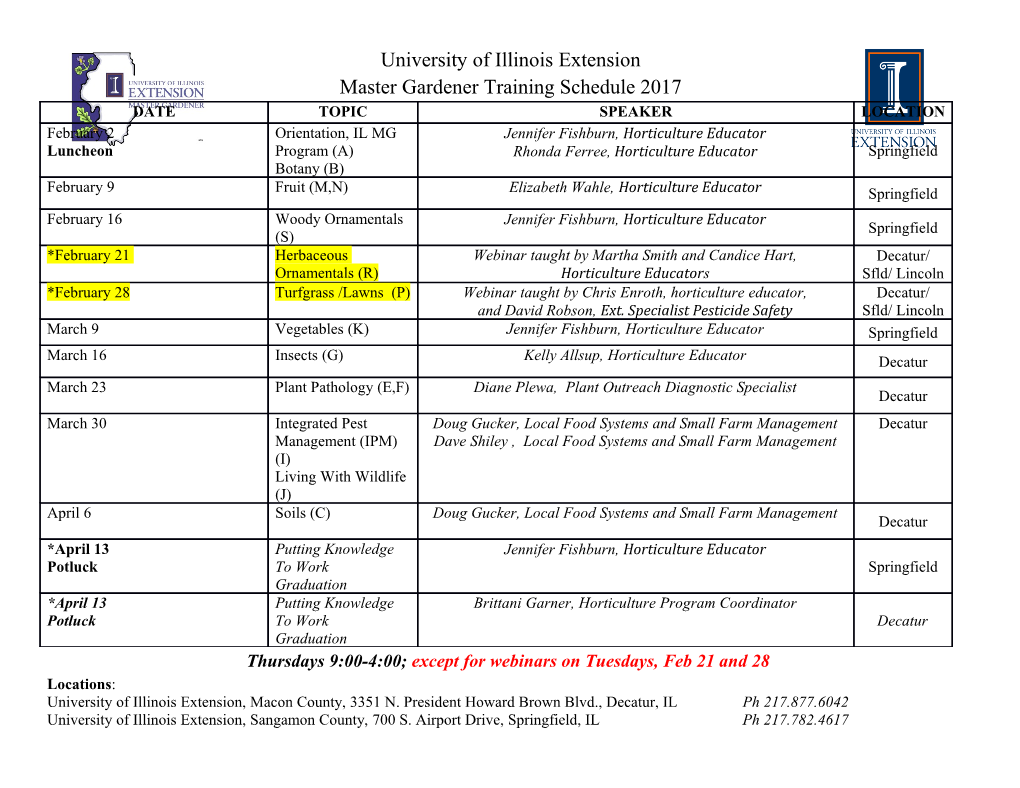
Multi-Model Ensemble Forecasts of Regional Air Quality in China Idir Bouarar, Guy P. Brasseur, Katinka Petersen Max Planck Institute for Meteorology, Hamburg, Germany With contribution from Bas Meijling (KNMI), Mikhail Sofiev and D. Rostislav (FMI) Outline qBackground qEnsemble Model Forecasting System qResults qImproving Model Performance qNext Steps Forecasting Air Quality in China: A Challenging Task • Air quality forecast systems rely also on meteorological models which still contain some inaccuracies in the formulation of physical processes • Chemical (and Physical) processes are not always carefully formulated: simplified assumptions are sometimes adopted • Large uncertainties in current emissions inventories • Urban environment “every where!”: - 9 megacities of 10-25 Million inhabitants - 37 Cities over 3 Million inhabitants • The formation mechanisms leading to severe haze episodes remain uncertain • Complex landscape and conditions in some cities: e.g. Beijing is prone to develop stagnant conditions, because of surrounding mountains to the north of the city • Coupling interactions between complex meteorological conditions, pollution sources, and atmospheric transformation processes • … An Ensemble of 7 Models to Forecast Air Quality in China ECMWF 40km Institute Model Emissions Domain Res. KNMI (NL) CHIMERE MEIC E. China: 0.25 v2013 INTEX-B 18-50N, 102- MEGAN 132E ECMWF C-IFS MACCity China: 10-49N, 0.4 (UK) 75-135E SCUEM SCUEM WRF- MEIC2010 East China: 6km (CN) Chem 21-44N, 104- 6km 132E FMI (FI) SILAM MACCity E. Asia: 0.25 GFAS 7-54N, 67-147E MEGAN MPI-M WRF- HTAPv2 E. China: 0.2 (DE) Chem FINN 18-45N, 95-125E MPI-M MEGAN 60km Met.NO EMEP HTAP+MEI China: 0.125 20km (NO) C 15-55N, 90 (PanHam) TNO (NL) LOTOS- MEIC E. China: 0.125 Euros Edgar 20-45N, 105- 130E Selected cities – Beijing – Harbin – Hefei – Shanghai – Suzhou – Changzhou – Guangzhou – Qingdao – Tangshan – Shenzhen – Jinan – Changsha – Hangzhou – Zhengzhou – Xuzhou – Tianjin – Dalian – Wenzhou – Chengdu – Kunming – Guiyang – Nanjing – Wuxi – Ürümqi – Xi’an – Xiamen – Zibo – Wuhan – ChangChun – Fuzhou – Shenyang – Ningbo – Shijiazhuang – Dongguan – Nanning – Chongqing – Taiyuan • Cities with over 3 million inhabitants (37), according to 2010 census • Most cities are covered by all models Surface Observations • Currently, collected from websites (e.g. www.pm25.in): – 367 different cities – 1526 stations • Species: O3, NO2, PM2.5, PM10 (soon CO, NO, SO2?) • Hourly data automatically copied to KNMI database, starting from April 2015 • Ground value given is an area average, typically based on 5-12 stations Schematic Overview Data Flow Courtesy B. Meijling Forecast ... Forecast Retrieval hourly model #1 model #N station measurements Postprocessing Postprocessing Retrieval from local server Central database (KNMI) www.marcopolo-panda.eu/forecast Calculation ensemble Generation images (time series and maps) Publication on webserver Operational Forecasting System at MPI-M with WRF-Chem (DKRZ, Hamburg, Germany) KNMI 10pm (LT) 6pm (LT) www.marcopolo-penda.eu Meteo. WRF NCEP GFS Meteo Forecasts spinup 10pm (LT) 00:00 (LT): Chem. BC: 3d Forcasts ECMWF Dissemination CAMS aqicn.org/forecast Chem. IC: Day -1 Forecast HTAPv2 FINN Emissions MarcoPolo-PANDA Forecasting Systems www.marcopolo-panda.eu/forecast Results q Cases: 1. All/Most Models Agree 2. All/Most Models Fail 3. Models Show Substantial Differences q Ensemble Model Performance Case 1: All/Most Models Agree CHIMERE WRF-Chem/SCUEM SILAM WRF-Chem /MPI Case 1 WRF-Chem Clean air CHIMERE Polluted air Case 1 WRF-Chem PM2.5 Prediction 28Nov.-02Dec. 2015 Case 2: All/Most Models Fail Special Haze Events in N. China PM2.5 Beijing 3 March 2016 WRF-Chem/SCUEM CHIMERE WRF-Chem MPI C-IFS SILAM Case 3: Models Show Substantial Differences CHIMERE C-IFS EMEP Lotos-Euros WRF-Chem WRF-Chem MPI-M SCUEM SILAM Mean Mediam Courtesy K. Rostislav Case 3 PM10 14 Apr. 2016 X’ian WRF-Chem CHIMERE Case 3 Observations 13 April 2016 05-06 May 2016 WRF-Chem SILAM SCUEM CIFS CHIMERE 28Nov.-02Dec. 2015 15-18 March 2016 Clear N Clear PM2.5 Ice Sunny Beijing Haze Fog 9C N S N S -3C NO2 Beijing Median Values O3 Beijing CHIMERE C-IFS EMEP Courtesy K. Rostislav O3 June 2016 LOTOS WRF/MPI WRF/SCUEM Bias r CHIMERE 25.8 0.83 C-IFS 19.1 0.79 EMEP 25.1 0.71 SILAM Mean Median LOTOS 44.1 0.74 MPI 45.5 0.72 SCUEM 74.7 0.78 SILAM 36.1 0.73 Mean 30.5 0.84 Median 29.2 0.83 CHIMERE C-IFS EMEP Courtesy K. Rostislav PM2.5 June 2016 LOTOS WRF/MPI WRF/SCUEM Bias r CHIMERE 37 0.63 C-IFS 74 0.47 EMEP 41 0.53 SILAM Mean Median LOTOS 20.8 0.65 MPI 28.8 0.4 SCUEM 14.7 0.54 SILAM 41.6 0.63 Mean 33.3 0.67 Median 25 0.64 Other challenging cases Beijing Coastal Cases X’ian Shanghai Chengdou Shanghai Guangzhou Regional + Urban Dust Complex Terrain X’ian Guangzhou Chinese new year! (Coincidence?) PM2.5 in Beijing [ug/m3], 8 February 2016 Diurnal Variability NO2 • Significant differences among models • Increased bias at nighttime • Mean/median ensemble forecast affected by individual model skills O3 Nighttime Boundary Layer in Urban Environments Daytime Nighttime NO2 Daytime O3 Nighttime WRF-Chem Beijing Boundary Layer Effect in Urban Environments Sensitivity to Emissions T1: CONTROL NOx T2: -50% NOx T3: MACCity Emissions (0.5x0.5) T4: Other PBL O3 Diurnal Variability of Emissions With diurnal variation Without diurnal variation 0.0800 0.0700 NOx 0.0600 0.0500 power 0.0400 industry 0.0300 residential 0.0200 transportation 0.0100 0.0000 0 2 4 6 8 10 12 14 16 18 20 22 hour O3 CO Effect of Vertical Diffusion CONTROL -50% NOx Kz >=10 CO NOx O3 Beijing O3 forecast Before After Perspectives • Keep Running the Systems : see, in long-term, how current measures to reduce emissions are efficient • Consistent Scientific Evaluation: Model-Intercomparison Exercise • Get Access to Monitoring Stations and Other Observations • Provide Other Products/Information: AQI, Other Chemical Species, Visibility, Haze • Further Investigate Boundary Layer Impact on Pollution in Urban Environment • Improve key model processes (e.g. SOA formation, key Met. Parameters…) • Update anthropogenic emissions (e.g. with satellite inversions) • Downscale to City-level • …… Improving Model Predictions Assimilation Meteorology Chemical IC : WRF-Chem/DART CTM CAMS BC Chemical Post-processing Analog-Based / Bias Correction BC Delle Monache et al. 2013 Emissions Life must go on ….
Details
-
File Typepdf
-
Upload Time-
-
Content LanguagesEnglish
-
Upload UserAnonymous/Not logged-in
-
File Pages32 Page
-
File Size-