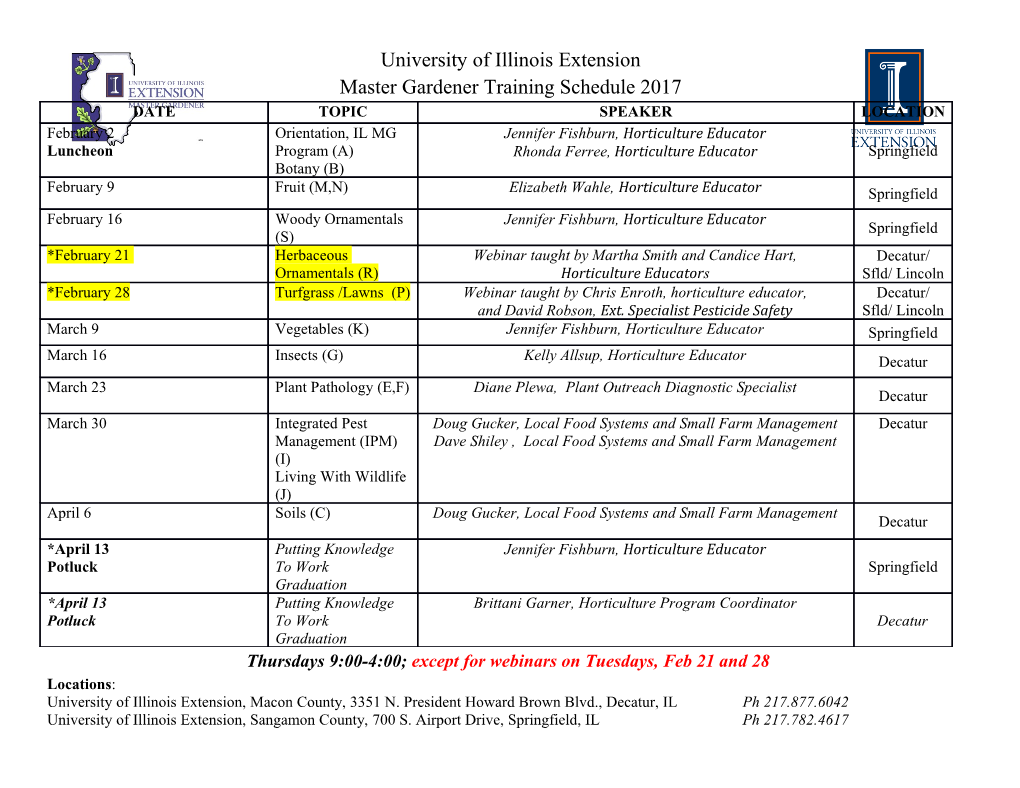
Data Warehouse 1. Methodological Framework Logical Modeling and Design • Conceptual Design & Logical Design (6) • Design Phases and schemata derivations 2. Logical Modelling: The Multidimensionnal Model Bernard ESPINASSE • Problematic of the Logical Design Professeur à Aix-Marseille Université (AMU) • The Multidimensional Model: fact, measures, dimensions Ecole Polytechnique Universitaire de Marseille 3. Implementing a Dimensional Model in ROLAP • Star schema January, 2020 • Snowflake schema • Aggregates and views 4. Logical Design: From Fact schema to ROLAP Logical schema Methodological framework • From fact schema to relational star-schema: basic rules Logical Modeling: The Multidimensional Model • Examples towards Relational Star Schema • Examples towards Relational Snowflake Schema Logical Design : From Fact schema to ROLAP Logical schema • Advanced logical modelling ROLAP schema in MDX for Mondrian 5. ROLAP schema in MDX for Mondrian Bernard ESPINASSE - Data Warehouse Logical Modelling and Design 1 Bernard ESPINASSE - Data Warehouse Logical Modelling and Design 2 • Livres • Golfarelli M., Rizzi S., « Data Warehouse Design : Modern Principles and Methodologies », McGrawHill, 2009. • Kimball R., Ross, M., « Entrepôts de données : guide pratique de modélisation dimensionnelle », 2°édition, Ed. Vuibert, 2003, ISBN : 2- 7117-4811-1. • Franco J-M., « Le Data Warehouse ». Ed. Eyrolles, Paris, 1997. ISBN 2- 212-08956-2. • Conceptual Design & Logical Design • Cours • Life-Cycle • Course of M. Golfarelli M. and S. Rizzi, University of Bologna • Design Phases • Course of M. Böhlen and J. Gamper J., Free University of Bolzano • Schemata derivations • … Bernard ESPINASSE - Data Warehouse Logical Modelling and Design 3 Bernard ESPINASSE - Data Warehouse Logical Modelling and Design 4 Methodological frameworkframework analysis of the db administrator operational db requirement specification designer conceptual design workload % refinement • Entite-Relation models are not very useful in modeling DWs logical • Is now universally recognized that a DW is based on a final 0user db administrator multidimensional view of data : design § But there is still no agreement on HOW to implement its 3 conceptual design ! 0 physical • Most of the time, DW design is at the logical level : a DWs are based on' a pre-existing design multidimensional model (star/snowflake schema) is directly designed : designer information system - 2 § But a star schema is nothing but a relational schema: it contains only the definition of a set of relations and integrity business user 51 & constraints ! • A better approach: "$& § 1) Design first a conceptual model : Conceptual Design § 2) Then translate this conceptual model into a logical !0 model : Logical Design J. Gamper, Free University of Bolzano, DWDM 2012/13 40 Methodological framework (2) Bernard ESPINASSE - Data Warehouse Logical Modelling and Design 5 Bernard ESPINASSE - Data Warehouse Logical Modelling and Design 6 A The 6 Logical Design transforms the Conceptual Schema for a DM into a Logical E/R Conceptual Logical Physical Schema 7 : Scheme Scheme Scheme Scheme • Choice of the type of logical schema • Tr anslation6 of conceptual schema to logical schema Relational & 1 4 1 5 Scheme • Optimization (view materialization, fragmentation) chiave ne gozio negoz io ci ttà re gione indirizzo resp. vendit e N1 ….….….…………… N2 chiave tempo chiave negozi o chi ave_pr odot to quant venduta incasso num_cli enti T1 N1P110 1000000 2 T1 N1P281200000 8 T1 N2P515 1500000 5 …….. …………. CONCEPTUAL LOGICAL PHYSICAL DESIGN DESIGN DESIGN Facts Workload Preliminary Target Workload Target Different principles from the one used in operational databases : workload logical DBMS • data redundancy model • denormalization 1 of tables 3 4 5 Bernard ESPINASSE - Data Warehouse Logical Modelling and Design 7 Bernard ESPINASSE - Data Warehouse Logical Modelling and Design 8 J. Gamper, Free University of Bolzano, DWDM 2012-13 10 52 Multidimensional Model : • is a logical model • has one purpose: Data analysis • is the most popular data model for DW • is more built in “meaning” : § What is important • The Multidimensional Model: § What describes the important § Fact, § What we want to optimize § Measures, § Dimensions § Automatic aggregations means easy querying • Star and Snowflake Schemata • is recognized by OLAP/BI tools : Tools offer powerful query facilities • Aggregates and Views based on MD design Bernard ESPINASSE - Data Warehouse Logical Modelling and Design 9 Bernard ESPINASSE - Data Warehouse Logical Modelling and Design 10 • Data is divided into facts (with measures) and dimensions Facts represent the subject of the desired analysis : the ”important” in the • Facts business that should be analyzed : § are the important entity, e.g., a sale • A fact is most often identified via its dimension values : § have measures that can be aggregated, e.g., sales price • Dimensions : § A fact is a non-empty cell § describe facts § Some models give facts an explicit identity § Ex : a sale has the dimensions Product, Store and Time • Generally, a fact should : • Goal for dimensional modeling: § Surround facts with as much context/dimensions as possible § be attached to exactly one dimension value in each dimension; (redundancy may be ok in well-chosen places) § only be attached to dimension values in the bottom levels § But you should not try to model all relationships in the data (unlike E/R and OO modeling!) § Ex : if the lowest time granularity is day, for each fact the exact day should be specified Facts (data) “live” in a multidimensional « cube » § some models do not require this. Bernard ESPINASSE - Data Warehouse Logical Modelling and Design 11 Bernard ESPINASSE - Data Warehouse Logical Modelling and Design 12 Different types of facts are distinguished : • Dimensions are the core of multidimensional databases • Event facts (transaction) : a fact for every business event (Ex : sale) • Other types of databases do not support dimensions • « Fact-less » facts : • Dimensions are used for : § A fact per event (Ex : customer contact) § Selection of data § No numerical measures § Grouping of data at the right level of detail § An event happened for a dimension value combination • Dimensions consist of dimension values • Snapshot fact : Ex : § A fact for every dimension combination at given time interval • Product dimension has values ”milk”, ”cream”, ... § Captures current status (Ex : inventory) • Time dimension has values ”1/1/2001”, ”2/1/2001”,.. • Cumulative snapshot facts : • Dimension values may have an ordering : § A fact for every dimension combination at given time interval § Used for comparing cube data across values § Captures cumulative status up to now (Ex : sales to date) § Especially used for Time dimension Every type of facts answers different questions : often event facts and Ex : percentage of sales increase compared with last month snapshot facts exist Bernard ESPINASSE - Data Warehouse Logical Modelling and Design 13 Bernard ESPINASSE - Data Warehouse Logical Modelling and Design 14 • Dimensions encode hierarchies with levels : Typically 3-5 levels (of • A location dimension with attributes street, city, province_or_state, and detail) country encodes implicitly the following hierarchy : • Dimension values are organized in a tree structure or lattice : Instance § Ex : Product: Product -> Type -> Category country Store: Store -> Area -> City > County All Time: Day -> Month -> Quarter -> Year Canada USA • Dimensions have a bottom level and a top level (ALL) Province_or_state • Levels may have attributes simple, non-hierarchical information British Colombia Quebec New York Illinois § Ex : Day has Workday as attribute • General rule: dimensions should contain much information : § Ex : city Vancouver ... Victoria Montréal ... Québec City New York ... Buffalo Chicago ... Urbana Time dimensions may contain holiday, season, events,... Good dimensions have 50-100 or more attributes/levels Bernard ESPINASSE - Data Warehouse Logical Modelling and Design 15 Bernard ESPINASSE - Data Warehouse Logical Modelling and Design 16 • A cube may have many dimensions : • Granularity of facts is important : § More than 3 – the term ”hypercube” is sometimes used § What does a single fact mean? § Theoretically no limit for the number of dimensions § Determines the level of detail § Typical cubes have 4-12 dimensions § Given by the combination of bottom levels • But only 2-3 dimensions can be viewed at a time : § Ex : ”total sales per store per day per product” § Dimensionality reduced by queries via projection/aggregation • Important for number of facts : Scalability • A cube consists of cells : • Often the granularity is a single business transaction : § A given combination of dimension values § A cell can be empty (no data for this combination) § Ex : sale § A sparse cube has many empty cells § Sometimes the data is aggregated (total sales per store per day § A dense cube has few empty cells per product) § Cubes become sparser for many/large dimensions. § Aggregation might be necessary for scalability Bernard ESPINASSE - Data Warehouse Logical Modelling and Design 17 Bernard ESPINASSE - Data Warehouse Logical Modelling and Design 18 • Measures represent the fact property that users want to study and 3 types of measures are distinguished : analyze : • Additive measures : § Ex : the total sales price § Can be aggregated over all dimensions
Details
-
File Typepdf
-
Upload Time-
-
Content LanguagesEnglish
-
Upload UserAnonymous/Not logged-in
-
File Pages15 Page
-
File Size-