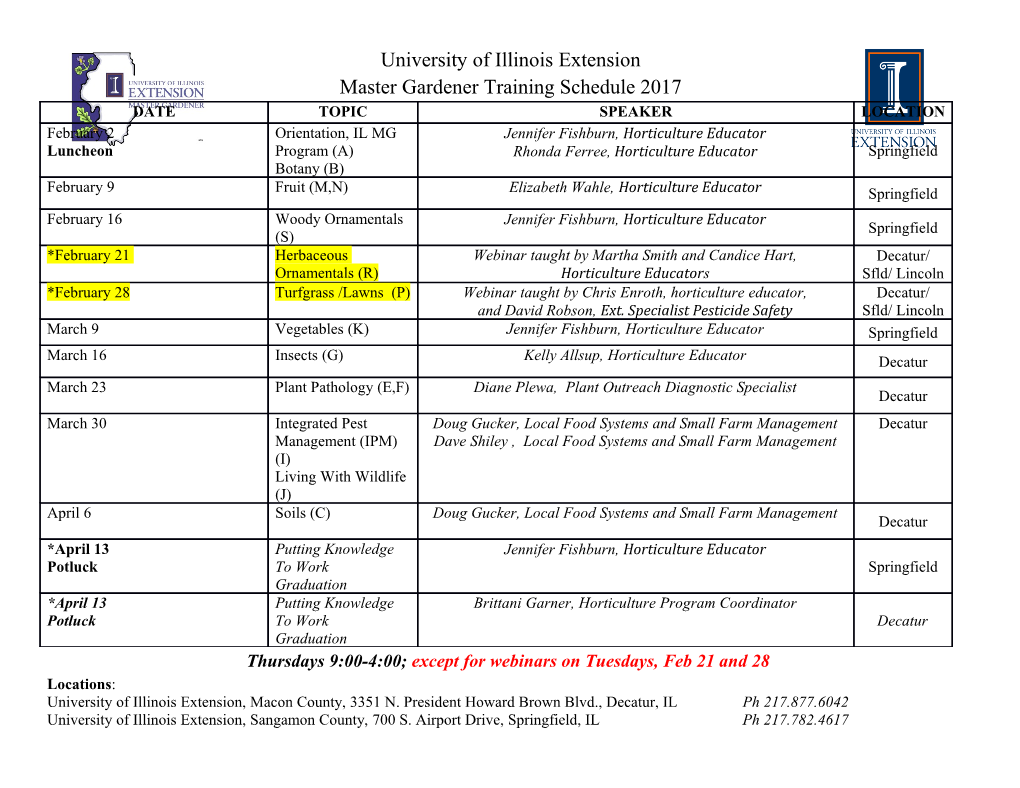
Cellular Wave Computers and CNN Technology – a SoC architecture with xK processors and sensor arrays* Tamás ROSKA1 Fellow IEEE 1. Introduction and the main theses 1.1 Scenario: Architectural lessons from the trends in manufacturing billion component devices when crossing the threshold of 100 nm feature size Preliminary proposition: The nature of fabrication technology, the nature and type of data to be processed, and the nature and type of events to be detected or „computed” will determine the architecture, the elementary instructions, and the type of algorithms needed, hence also the complexity of the solution. In view of this proposition, let us list a few key features of the electronic technology of Today and its consequences.. (i) Convergence of CMOS, NANO and OPTICAL technologies towards a cellular architecture with short and sparse wires CMOS chips: * Processors: K or M transistors on an M or G transistor die =>K processors /chip * Wires: at 180 nm or below, gate delay is smaller than wire delay NANO processors and sensors: * Mainly 2D organization of cells integrating processing and sensing * Interactions mainly with the neighbours OPTICAL devices: * parallel processing * optical correlators * VCSELs and programable SLMs, Hence the architecture should be characterized by * 2 D layers (or a layered 3D) * Cellular architecture with * mainly local and /or regular sparse wireing leading via Î a Cellular Nonlinear Network (CNN) Dynamics 1 The Faculty of Information Technology and the Jedlik Laboratories of the Pázmány University, Budapest and the Computer and Automation Institute of the Hungarian Academy of Sciences, Budapest, Hungary ([email protected], www.itk.ppke.hu) * Research supported by the Office of Naval Research, Human Frontiers of Science Program, EU Future and Emergent Technologies Program, the Hungarian Academy of Sciences, and the Jedlik Laboratories of the Pázmány University, Budapest 0-7803-9254-X/05/$20.00 ©2005 IEEE. 557 towards Î Cellular Wave Computers (ii) The DATA and EVENTS are: DATA: Multimodal data flows: 2D topographicFLOWS, combined with non- topographic signals, „flowing” continuously from the sensor arrays, tuned interactively with the processor array EVENTS: Spatial-temporal multimodal events - spatial-temporal PATTERNS (iii) The algorithms and logic: *Spatial-temporal Non-Boolean logic on dynamic patterns via *α recursive functions A new world of software with elementary instructions as nonlinear waves (PDE solutions) is emerging implemented in the stored programmable sensory cellular wave computers. 1.2 Mind-like versus brain-like computing Present day classical computers, developed during the last sixty years are essentially logic machines, based on binary logic and arithmetic, acting on discrete valued (binary coded) data. Its unique property is algorithmic (stored) programmability, invented by John von Neumann. The mathematical concept is based on a Universal Machine on integers (Turing Machine). We call it a Mind-like computer, since the elementary instructions are based on arithmetic-and-logic operations, abstract notions reflecting our mind, naturally abstracted from the world. Their algorithms are logic sequences on these operations. When these machines have been invented, this was in complete agreement with the view how neurons and the brain were envisaged, as threshold logic. The invention of the transistor (1948) and later the integrated circuit (1960) made this computer architecture not only practical, but also cheap and, nowadays, an ubiquitous commodity. However, now we know that neurons and the nervous tissues operate differently. Today, a brain-like system has the following properties: • Continuous time continuous valued (analog) signal arrays (flows) • Several 2 Dimensional strata of analog „processors” (neurons)Typically, mainly local, or sparse global (bus-like) interconnectionsSensing and processing are integratedVertical interconnections between a few strata of neuron “processors”Variable delaysSpatial-temporal active waves with Events as patterns in space and/or timeThese features are seemingly in almost complete agreement with the properties concluded in the previous section. Hence, they are strongly modifying our view and practice in building complex electronic systems, including sensing, computing, activating and communicating devices and systems. This way of thinking, however, is supposing a completely different architecture, physical and algorithmic alike, and supposes tens of thousands or millions of parallel physical processing devices. 2 An axiomatic introduction of adaptive sensory Cellular Wave Computers and Wave-Logic 558 A universal and canonical computing architecture, after the forms of data are set, contains the simplest possible building blocks, with the simplest possible interconnections, elementary instructions and programming constructs. Then we introduce algorithmic stored programmability to make it universal and practical. A most successful example is the digital computer, with a core universal machine on integers (Turing machine). 2.1 The basic cellular nonlinear network CNN dynamics In view of the properties of a brain-like computer, the data are topographic (image) flows. We assign one cell processor to one sensory element (pixel, taxel, etc.). In the simplest case: we have a time varying pixel array with each pixel, at each time instant (defining a picture), having a light intensity of gray values between black (say, +1) and white (say, – 1) values. Color pictures are composed of several pictures with different color content. A special caseof a picture is a binary (black-andwhite) mask. Now, let us construct a programmable topographic cellular sensory dynamics, as implementing the protagonist elementary instruction. The recipe is as follows. • Take the simplest dynamical system, a cell (input u, state x and output y are all real valued vector function of the continuous valued time)) • Take the simplest spatial grid for placing the cells with the simplest neighborhood relation (2D sheets) • Introduce the simplest spatial interactions between dynamic cells, being programmable (called cloning template or gene, or simply template) • Add cellular sensors, typically, cell by cellThese steps are leading to a one-layer cellular nonlinear network (CNN) architecture with programmable cloning templates T {A, B, z} shown in Figures 1. The corresponding canonical dynamic differential equations for each cell are shown in Figure 2, and a bus-like sparse set of connections is useful in siome cases, as well. Multi-layer architectures are not considered here. Details can be found in the recent textbook [1]. Observe that : The canonical differential equations shown in Figure 2, called also as standard CNN dynamics are very sparse and very simple, though quite rich in spatial- temporal dynamics. Each equation contains 20 terms only (including the time constant) for a 3x3 neighborhood, independently of the number of cells. These 20 (19 if the time constant is considered as a unit) terms are the parameters of the cloning template T. Many complex wave equations can be described by them, with possible 2-layer architecture. Indeed, the archetype is the Turing morphogenesis equation, a special case of this 2-layer CNN equation. This more general definition of CNN is including many special constructs (including multilayer or complex cell), many dynamic patterns as detected events (stable gray scale or binary patterns, periodic attractors, spatial-temporal chaotic attractors, etc.), and is physical implementation independent. The diverse physical implementations so far include: a mixed signal (analog-binary) circuit array, an optical system, an emulated digital device, a quantum dot array, a molecular array (e.g. bacteriorhodopsine), etc. As a special case, the CNN could be programmed to model locally connected neural networks, e.g. modeling different retinas, etc. 559 Considering the input array flow and the output array flow as the input-output relation, the CNN dynamics is an elementary instruction of an array computer on image flows. The functionality of it is described by the cell dynamics and the cloning template. Notice that this computing array is not necessarily a Single Instruction Multiple Data (SIMD) computing machine, indeed, with a slight extension (already available in operational visual microprocessors) it is also a Multiple Instruction Multiple Data (MIMD) machine, having a space variant (even locally adaptive) template Tij (the simplest case is the space variant threshold or bias, bij ). 2.2 The Universal Machine on Flows and the Cellular Wave Computer [1, 2, 7] Now, we select the CNN dynamics as an elementary instruction of an array computer on image flows. This is a drastic departure in constructing a computer, with the protagonist instruction implemented by a programmable CNN dynamics solving a nonlinear wave equation on data as image flows. The axiomatic foundation or the recipe to form a generic spatial-temporal machine is as follows. • Take the topographic sensory cellular dynamics, axiomatically introduced in Section 2.1 , as the protagonist instruction, with programmable templates; • Construct global operators (functional) on a picture or on an image flow;Construct local memories in each cell to store intermediate results cell by cell;Construct a local communication and control unit in each cell communicating with the global programming unit, called the Global Analog-logic Programming Unit (GAPU);This unit, the GAPU, hosts the global
Details
-
File Typepdf
-
Upload Time-
-
Content LanguagesEnglish
-
Upload UserAnonymous/Not logged-in
-
File Pages8 Page
-
File Size-