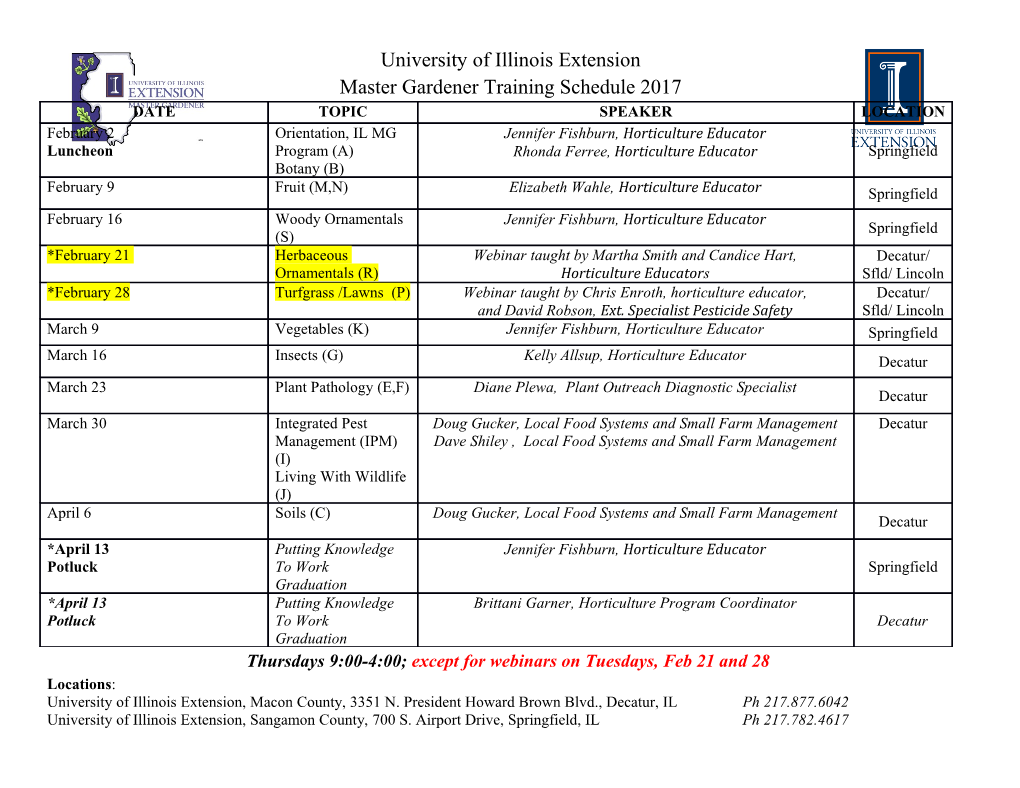
Asymptotic normality in combinatorics Xi Chen School of Mathematical Sciences Dalian University of Technology [email protected] 2017 International Conference on Combinatorics Institute of Mathematics, Academia Sinica, Taipei, Taiwan May 19, 2017 Xi Chen (DUT) Asymptotic normality in combinatorics May 19, 2017 1 / 35 Contents 1 Asymptotic normality 2 Methods and techniques 3 Narayana numbers 4 Problems and conjectures Xi Chen (DUT) Asymptotic normality in combinatorics May 19, 2017 2 / 35 Outline 1 Asymptotic normality 2 Methods and techniques 3 Narayana numbers 4 Problems and conjectures Xi Chen (DUT) Asymptotic normality in combinatorics May 19, 2017 3 / 35 Normal distribution (Gaussian distribution) A random variable X is normally distributed, write X ∼ N (µ, σ2), if Z x 2 1 − (t−µ) Prob(X ≤ x) = p e 2σ2 dt: 2πσ2 −∞ Having achieved fame with his calculation on the orbit of asteroid Ceres (discovered in 1801), Gauss later published a treatise on the subject of celestial orbits. In this work he propounded the method of least squares, and provided theoretical justification by assuming that observational measurements are normally distributed about their mean. probability density function of N (µ, σ2) Carl F. Gauss (1777{1855) Xi Chen (DUT) Asymptotic normality in combinatorics May 19, 2017 4 / 35 De Moivre-Laplace theorem Let Xi ; 1 ≤ i ≤ n, be a sequence of independent 0; 1 random variables Pn with Prob(Xi = 1) = p and Prob(Xi = 0) = q = 1 − p. Let Sn = i=1 Xi . De Moivre-Laplace theorem For any fixed x, Z x 2 p 1 − t Prob(Sn < np + x npq) ! p e 2 dt; 2π −∞ as n ! 1. The mean and variance of Sn are np and npq, respectively. p X n k n−k Prob(Sn < np + x npq) = p q . p k k<np+x npq Xi Chen (DUT) Asymptotic normality in combinatorics May 19, 2017 5 / 35 Generalization of de Moivre-Laplace theorem Let Xi ; 1 ≤ i ≤ n, be a sequence of independent 0; 1 random variables Pn with Prob(Xi = 1) = pi . Let Sn = i=1 Xi with mean and variance n n X 2 X µn = pi ; σn = pi (1 − pi ): i=1 i=1 De Moivre-Laplace theorem: generalization Provided σn ! 1, then for any fixed x, Z x 2 1 − t Prob(Sn < µn + xσn) ! p e 2 dt; 2π −∞ as n ! 1. Xi Chen (DUT) Asymptotic normality in combinatorics May 19, 2017 6 / 35 Berry-Esseen theorem Let Xi ; 1 ≤ i ≤ n, be independent random variables with means µi , 2 3 variances σi , and absolute third central moments ρi = EjXi − µi j . Let Pn P 2 P 2 Sn = i=1 Xi with mean µ = i µi and variance σ = i σi . Berry-Esseen theorem There exist a constant C such that Z x 2 Pn 1 − t C i=1 ρi p 2 Prob(Sn < µ + xσ) − e dt ≤ 3 : 2π −∞ σ A.C. Berry, The accuracy of the Gaussian approximation to the sum of independent variates, Trans. AMS, 1941. C.-G. Esseen, On the liapunoff limit of error in the theory of probability, Arkiv for Matematik, Astronomi och Fysik, 1942. Xi Chen (DUT) Asymptotic normality in combinatorics May 19, 2017 7 / 35 Asymptotic normality Let a(n; k) be a double-indexed sequence of nonnegative numbers and Pn p(n; k) = a(n; k)= j=0 a(n; j) the normalized probabilities. We say that a(n; k) is asymptotically normal by a central limit theorem with mean µn 2 and variance σn if Z x X 1 −t2=2 lim sup p(n; k) − p e dt = 0: (1) n!1 x2R 2π −∞ k≤µn+xσn Say that a(n; k) is asymptotically normal by a local limit theorem on S if 1 −x2=2 lim sup σnp(n; bµn + xσnc) − p e = 0: (2) n!1 x2S 2π When S = R, the validity of (2) implies that of (1) and e−x2=2 Pn a(n; j) a(n; k) ∼ j=0 ; n ! 1; p 2 2πσn where k = µn + xσn for some fixed x. Xi Chen (DUT) Asymptotic normality in combinatorics May 19, 2017 8 / 35 Outline 1 Asymptotic normality 2 Methods and techniques 3 Narayana numbers 4 Problems and conjectures Xi Chen (DUT) Asymptotic normality in combinatorics May 19, 2017 9 / 35 Method 1: direct approach Sometimes the enumeration problem, suitably normalized, can be viewed as the distribution of a sum of independent 0; 1 variables. Then the generalization of de Moivre-Laplace theorem is applicable. Example number of cycles in a permutation; number of left-to-right minima in a permutation; number of inversions in a permutation; number of distinct prime divisors (Erd}os-Kactheorem). W. Feller, The fundamental limit theorems in probability, Bull. AMS, 1945. W. Feller, An Introduction to Probability Theory and Its Applications, volume I, 1957. P. Erd}os,M. Kac, The Gaussian law of errors in the theory of additive number theoretic functions, American Journal of Mathematics, 1940. M. Kac, Enigmas of Chance: An Autobiography, 1985. Xi Chen (DUT) Asymptotic normality in combinatorics May 19, 2017 10 / 35 Method 2: RZness Let [a(n; k)]n;k≥1 be an infinite lower triangular matrix with nonnegative Pn k a(n;k) entries. Let An(x) = a(n; k)x and p(n; k) = P . Then k=0 j a(n;j) 0 X An(1) µn = kp(n; k) = An(1) k and 00 2 X 2 2 An(1) 2 σn = k p(n; k) − µn = + µn − µn: An(1) k Qn In particular, if An(x) = i=1(x + rn;i ) have only negative zeros, then n n X 1 2 X rn;i µn = ; σn = 2 : 1 + rn;i (1 + rn;i ) i=1 i=1 2 It follows that σn ≤ µn; 8n. Xi Chen (DUT) Asymptotic normality in combinatorics May 19, 2017 11 / 35 Central limit theorem Theorem P k 2 Let An(x) = k a(n; k)x have only real zeros, and let µn; σn be the asso- 2 ciated means and variances. If σn ! +1, then a(n; k) are asymptotically normal by a central limit theorem. P. L´evy, Sur une propri´et´ede la loi de poisson relative aux petites probabilit´es, Soc. Math. de France, Comptes rendus des sc´eances de l'ann´ee,1936. L.H. Harper, Stirling behavior is asymptotically normal, Ann. Math. Statist., 1967. Xi Chen (DUT) Asymptotic normality in combinatorics May 19, 2017 12 / 35 Local limit theorem Theorem (Bender, 1973) 2 Suppose that a(n; k) satisfy a central limit theorem, and σn ! +1. 1 If a(n; k) is unimodal in k for each n, then a(n; k) satisfy a local limit theorem on S = fx : jxj ≥ "g; 8" > 0; 2 if a(n; k) is log-concave in k for each n, then a(n; k) satisfy a local limit theorem on R. E.A. Bender, Central and local limit theorems applied to asymptotic enumer- ation, JCTA, 1973. Xi Chen (DUT) Asymptotic normality in combinatorics May 19, 2017 13 / 35 Strongly asymptotic normality Newton's inequality Pn k If k=0 ak x has only real zeros, then 1 1 a2 ≥ a a 1 + 1 + ; k k−1 k+1 k n − k and the sequence a0; a1;:::; an is therefore log-concave and unimodal. Definition P k 2 If An(x) = k a(n; k)x have only real zeros and σn ! +1, then we say that a(n; k) are strongly asymptotically normal. In this case, a(n; k) satisfy both central and local limit theorems. Xi Chen (DUT) Asymptotic normality in combinatorics May 19, 2017 14 / 35 Polynomials with only real zeros Theorem (Wang-Yeh, 2005) Pn k Suppose that An(x) = k=0 a(n; k)x and a(n; k) satisfy a(n; k) = (rn + sk + t)a(n − 1; k − 1) + (an + bk + c)a(n − 1; k): If rb ≥ as and (r + s + t)b ≥ (a + c)s, then An(x) have only real zeros. Theorem (Liu-Wang, 2007) Suppose that An(x) satisfy deg An−1(x) ≤ deg An(x) ≤ deg An−1(x) + 1 and 0 An(x) = an(x)An−1(x) + bn(x)An−1(x) + cn(x)An−2(x): if bn(x); cn(x) ≤ 0 for x ≤ 0, then An(x) have only real zeros. Wang, Yeh, Polynomials with real zeros and P´olya frequency sequences, JCTA, 2005. Liu, Wang, A unified approach to polynomial sequences with only real zeros, AAM, 2007. Xi Chen (DUT) Asymptotic normality in combinatorics May 19, 2017 15 / 35 Examples of strongly asymptotically normal numbers Example n binomial coefficients k signless Stirling numbers of the first kind c(n; k) Stirling numbers of the second kind S(n; k) Eulerian numbers A(n; k) matching numbers of a graph Laplacian coefficients of a graph W. Feller, The fundamental limit theorems in probability, Bull. AMS, 1945. L.H. Harper, Stirling behavior is asymptotically normal, Ann. Math. Statist., 1967. L. Carlitz, D.C. Kurtz, R. Scoville, O.P. Stackelberg, Asymptotic properties of Eulerian numbers, Z. Wahrscheinlichkeitstheorie und Verw. Gebiete, 1972. C.D. Godsil, Matching behaviour is asymptotically normal, Combinatorica, 1981. Y. Wang, H.-X. Zhang, B.-X. Zhu, Asymptotic normality of Laplacian coefficients of graphs, J. Math. Anal. Appl., accepted. Xi Chen (DUT) Asymptotic normality in combinatorics May 19, 2017 16 / 35 Method 3: moments For a random variable X , the associated moment generating function is defined by tX MX (t) := Ee : t2=2 If X ∼ N (0; 1), then MX (t) = e . Theorem (Curtiss, 1942) Suppose Xn is a sequence of random variables with distribution functions 2 t2=2 1 R x − t F (x).
Details
-
File Typepdf
-
Upload Time-
-
Content LanguagesEnglish
-
Upload UserAnonymous/Not logged-in
-
File Pages35 Page
-
File Size-