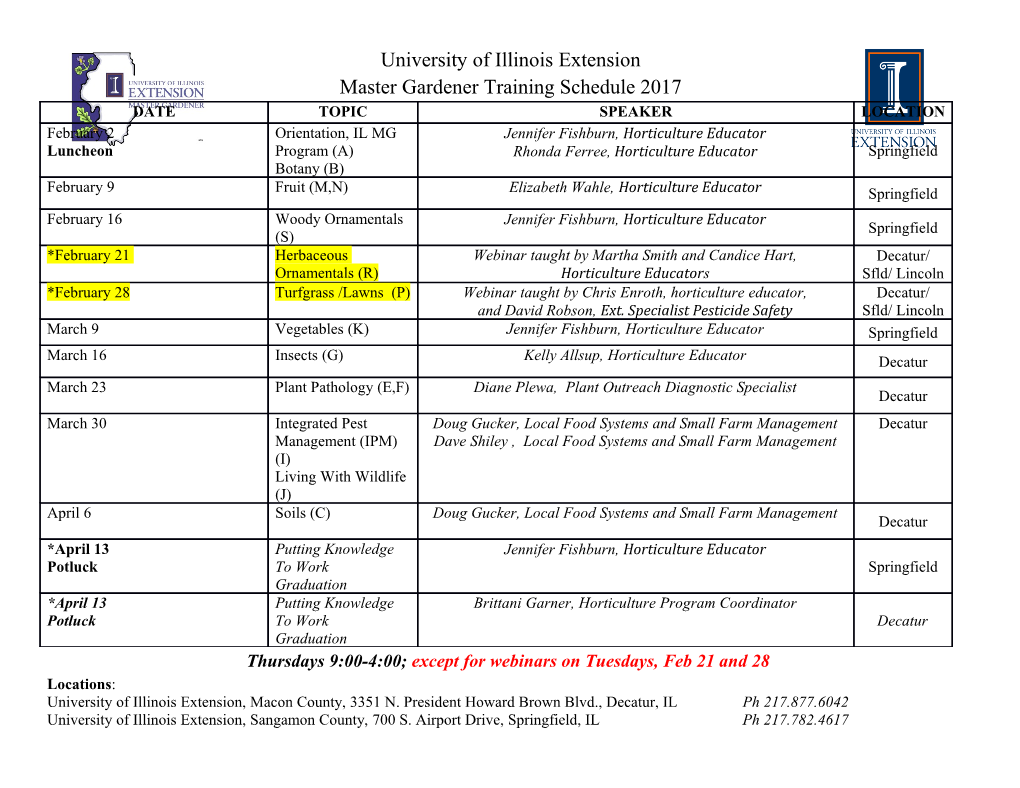
2 ��� Talk.nb Automatic Machine Learning & Symbolic Neural Networks in the Wolfram Language Etienne Bernard - Wolfram Research [email protected] Talk.nb ���3 4 ��� Talk.nb Wolfram Language ◼ 1988: Mathematica �������� Solve[3*x+2⩵ 0, x] 2 �������� x→- 3 �������� DSolve[y'[x] == 10*y[x], y[x], x] 10 x �������� y[x]→ⅇ 1 �������� Plot3D[Sin[x]* y,{x, 1, 10},{y, 1, 2}] �������� ◼ 5000+ functions ◼ http://reference.wolfram.com/language/ Talk.nb ���5 ◼ Coherent ◼ High level � BlurFacesimage_:= FoldblurSingleFace, image, FindFacesimage,"Position", "Image" blurSingleFaceimage_, face_:= ImageComposeimage, Blurface["Image"], 50, face" Position 6 ��� Talk.nb ��������� BlurFaces ��������� ◼ Knowledge based ��������� ����� ��������� ������ ����� Interval Year: 2012 , Day: Thu 14 Nov 2019 Talk.nb ���7 @ ����� �� ��� ���� �� �� ��� ���� ��������� Normal TimeSeries ���� ������� ���� Tue 3 Jan 2012 00:00:00 GMT-8. , $24.54 , Wed 4 Jan 2012 00:00:00 GMT-8. , $25.11 , Thu 5 Jan 2012 00:00:00 GMT-8. , $25.40 , ⋯ 1975⋯ , Mon 11 Nov 2019 00:00:00 GMT-8. , $58.35 , ��������� Tue 12 Nov 2019 00:00:00 GMT-8. , $58.20 , Wed 13 Nov 2019 00:00:00 GMT-8. , $57.89 ����� ������ ���� ���� ���� ���� ���� ��� ��� ���� �������� ����� �� ��� ���� �� �� ��� ���� ��������� DateListPlot TimeSeries ���� ������� ���� 60 50 40 ��������� 30 20 10 0 2012 2014 2016 2018 2020 8 ��� Talk.nb Classify ��������� scrapeImages[string_]:= Thread[WebImageSearch[string, "Thumbnails", MaxItems→ 30]→ string] ��������� classes={"Google logo", "Intel logo", "Apple logo", "Facebook Logo"}; ��������� images= scrapeImages/@ classes; ��������� data= RandomSample[Join@@ images]; training= data[[ ;; 80]]; test= data[[81 ;;]]; ��������� training ��������� logo= Classify[training] ����� ����� ����� ��������� ClassifierFunction �������� ����� ����� �������� ����� ������ ����� ����� ���� ���� ��� �� ��������� ����� ��� � ��������� test[[1]] ��������� → Facebook Logo ��������� logo ��������� Facebook Logo ��������� logo , "Probabilities" ��������� Apple logo→ 0.0053377, Facebook Logo→ 0.991869, Google logo→ 0.0000165805, Intel logo→ 0.00277714 ��������� cm= ClassifierMeasurements[logo, test] ���������� ������������������ ��������� ClassifierMeasurementsObject ������ �� ���� ��������� �� ���� ��� �� ��������� ����� ��� � Talk.nb ���9 ��������� cm["Report"] ��������� ������������ Number of test examples 40 Accuracy (88. ± 5.)% Accuracy baseline (30. ± 7.)% Geometric mean of probabilities 0.441 ± 0.16 Mean cross entropy 0.820 ± 0.36 Single evaluation time 95.1 ms/example Batch evaluation speed 11.9 examples/s Rejection rate 0% ��������� Apple logo Facebook Logo Google logo Intel logo Apple logo 4 0 2 0 6 Facebook Logo 0 11 0 1 12 actual class Google logo 0 0 10 0 10 Intel logo 0 0 2 10 12 4 11 14 11 predicted class ��������� cm["Examples"→{"Apple logo", "Google logo"}] ��������� → Apple logo, → Apple logo ��������� cm["BestClassifiedExamples"] 10 ��� Talk.nb ��������� → Intel logo, → Google logo, → Google logo, → Google logo, → Facebook Logo, → Facebook Logo, → Facebook Logo, → Apple logo, → Intel logo, → Facebook Logo Talk.nb ���11 form= FormFunction[{"image"→ "Image"}, logo[#image, "TopProbabilities"]&] image ������… FormFunction Submit url= CloudDeploy[form, Permissions→ "Public"] CloudObjecthttps://www.wolframcloud.com/obj/0246a5cd-08b7-47df-9524-d9d74055201b URLShorten[url] https://wolfr.am/I5j1y3V4 12 ��� Talk.nb Neural Networks framework ◼ High level ◼ No performance compromise Talk.nb ���13 A network from scratch ��������� lenet= NetChain[{ ConvolutionLayer[20, 5], Ramp, PoolingLayer[2, 2], ConvolutionLayer[50, 5], Ramp, PoolingLayer[2, 2], FlattenLayer[], LinearLayer[500], Ramp, LinearLayer[10], SoftmaxLayer[] }, "Output"→ NetDecoder[{"Class", Range[0, 9]}], "Input"→ NetEncoder[{"Image",{28, 28}, "Grayscale"}] ] ����� ��������� NetChain uninitialized ����� ����� (����� �× ��× ��) � ���������������� ����� (����� ��× ��× ��) � ���� ����� (����� ��× ��× ��) � ������������ ����� (����� ��× ��× ��) � ���������������� ����� (����� ��×�×�) � ���� ����� (����� ��×�×�) � ������������ ����� (����� ��×�×�) � ������������ ������ (����� ���) � ����������� ������ (����� ���) � ���� ������ (����� ���) �� ����������� ������ (����� ��) �� ������������ ������ (����� ��) ������ ����� �� ���������������� ���������� ������ ��������� �� ������ ����� {�� �} ������� {�� �} ������� ����� {{�� �}�{�� �}} ��������� {�� �} ������� ������� � ��������������� � ������������� ����� ������ �������� ����� (����� ��× ��×�×�) ������� �������� ������ (����� ��) ����� ������ ����� (����� ��× ��× ��) ������� ����� (����� ��×�×�) ��������� training= ResourceData["MNIST"]; test= ResourceData["MNIST", "TestData"]; 14 ��� Talk.nb ��������� RandomSample[training, 10] ��������� → 2, → 1, → 7, → 3, → 2, → 7, → 6, → 3, → 3, →0 ��������� trainednet= NetTrain[lenet, training] ����� ��������� NetChain ����� ����� (����� �× ��× ��) � ���������������� ����� (����� ��× ��× ��) � ���� ����� (����� ��× ��× ��) � ������������ ����� (����� ��× ��× ��) � ���������������� ����� (����� ��×�×�) � ���� ����� (����� ��×�×�) � ������������ ����� (����� ��×�×�) � ������������ ������ (����� ���) � ����������� ������ (����� ���) � ���� ������ (����� ���) �� ����������� ������ (����� ��) �� ������������ ������ (����� ��) ������ ����� �� ���������������� ���������� ������ ��������� �� ������ ����� {�� �} ������� {�� �} ������� ����� {{�� �}�{�� �}} ��������� {�� �} ������� ������� � ��������������� � ������������� ����� ������ �������� ����� (����� ��× ��×�×�) ������� ������ (����� ��) ����� ������ ����� (����� ��× ��× ��) ������� ����� (����� ��×�×�) ��������� RandomSample[test, 10] ��������� → 6, → 7, → 2, → 9, → 6, → 2, → 4, → 3, → 3, →0 ��������� trainednet , "Probabilities" -9 -6 ��������� 0→ 5.3643× 10 , 1→ 1.40952× 10 , 2→ 0.999993, 3→ 4.97541× 10 -6, 4→ 1.29769× 10 -9, 5→ 3.08827× 10 -11, 6→ 9.05024× 10 -12, 7→ 6.28407× 10 -7, 8→ 2.40183× 10 -7, 9→ 2.87436× 10 -10 Talk.nb ���15 ��������� net6= NetTake[trainednet, 6] ����� ��������� NetChain ����� ����� (����� �× ��× ��) � ���������������� ����� (����� ��× ��× ��) � ���� ����� (����� ��× ��× ��) � ������������ ����� (����� ��× ��× ��) � ���������������� ����� (����� ��×�×�) � ���� ����� (����� ��×�×�) � ������������ ����� (����� ��×�×�) ������ ����� (����� ��×�×�) ��������� CurrentImage[] ��������� ��������� Dynamic[Image/@ net6[CurrentImage[]]] 16 ��� Talk.nb Network repository ◼ https://resources.wolframcloud.com/NeuralNetRepository ◼ ~ 100 networks, growing ��������� bert= NetModel["BERT Trained on BookCorpus and English Wikipedia Data"] ������ ��������� NetChain ����� ������ (������×�) �� ������� (������ ����� ���) ��������� �������� (�� �����) ������ (������× ���) ������� �������� (�� �����) ������ (������× ���) ������ ������ (������× ���) �������� �������� ����� ������ (������× ���) � �������� (� �����) ������ (������× ���) � �������� (� �����) ������ (������× ���) � �������� (� �����) ������ (������× ���) � �������� (� �����) ������ (������× ���) � �������� (� �����) ������ (������× ���) � �������� (� �����) ������ (������× ���) � �������� (� �����) ������ (������× ���) � �������� (� �����) ������ (������× ���) � �������� (� �����) ������ (������× ���) �� �������� (� �����) ������ (������× ���) �� �������� (� �����) ������ (������× ���) �� �������� (� �����) ������ (������× ���) ������ ������ (������× ���) �� �������� ����� ������ (������× ���) � �������� (� �����) ������ (������× ���) � �������� (� �����) ������ (������× ���) ������ ������ (������× ���) �� �������� n ⨯ 768 n⨯ 768 n⨯ 768 + N Output 768 ⨯ add norm n n⨯ 768n⨯ 768 D Input attention dropout ���������� �������� 7 n ⨯ 64 768 768 ⨯ ⨯ n n n 768 6 ⨯ ⨯ 64 n 768 ⨯ n n ⨯ 768 64 768 768 ⨯ ⨯ ⨯ n 768 n n 768⨯ 5 n n ⨯ ⨯ 64 n 768 n⨯⨯ 768 n⨯ 64 n⨯ 768 n⨯ 768 n C M Query 64 Output n768 4 ⨯ 13 14 ⨯ ⨯ n n 768 64 ⨯ n n⨯ 768 n ⨯ 768 Input n n⨯ ⨯ 3 64 768 n 768 ⨯ n n ⨯ n ⨯ ⨯ 768 768 768 Talk.nb ���17 768 768 768 n ⨯ 768 64 n ⨯ ⨯ 2 n n 768 n ⨯ ⨯ 768 768 64 ⨯ 12 n 64 ⨯ 11 n 10 1 �� �������� n⨯ 768 n⨯ 64 M Query query elem n ⨯ 64 n⨯ 64 n⨯ 64 M A Output 768 64 ⨯ value⨯ attention n n n⨯ 768 M Input key ���������� �������������� ���������� ������� ���� ��������[…] ����� ���� ����� ���������� ���� ����� ���� ������ (������× ��) ������ ������ (������× ��) ������ ������ (������× ��) ������� ������ (������× ��) 18 ��� Talk.nb Surgery ◼ Surgery functions: ◼ NetExtract, NetRename, NetReplace, NetTake, NetJoin, NetAppend, NetDrop, NetInsert, ... ��������� resnet= NetModel["Inception V3 Trained on ImageNet Competition Data"] ����� ��������� NetChain ����� ����� (����� �× ���× ���) ����_������ ���������������� ����� (����� ��× ���× ���) ����_��������� ����������������������� ����� (����� ��× ���× ���) ����_���� ���� ����� (����� ��× ���× ���) ����_�_������ ���������������� ����� (����� ��× ���× ���) ����_�_��������� ����������������������� ����� (����� ��× ���× ���) ����_�_���� ���� ����� (����� ��× ���× ���) ����_�_������ ���������������� ����� (����� ��× ���× ���) ����_�_��������� ����������������������� ����� (����� ��× ���× ���) ����_�_���� ���� ����� (����� ��× ���× ���) ���� ������������ ����� (����� ��× ��× ��) ����_�_������ ���������������� ����� (����� ��× ��× ��) ����_�_��������� ����������������������� ����� (����� ��× ��× ��) ����_�_���� ���� ����� (����� ��× ��× ��) ����_�_������ ���������������� ����� (����� ���× ��× ��) ����_�_��������� ����������������������� ����� (����� ���× ��× ��) ����_�_���� ���� ����� (����� ���× ��× ��) ����� ������������ ����� (����� ���× ��× ��) ���������� �������� (�� �����) �����
Details
-
File Typepdf
-
Upload Time-
-
Content LanguagesEnglish
-
Upload UserAnonymous/Not logged-in
-
File Pages29 Page
-
File Size-