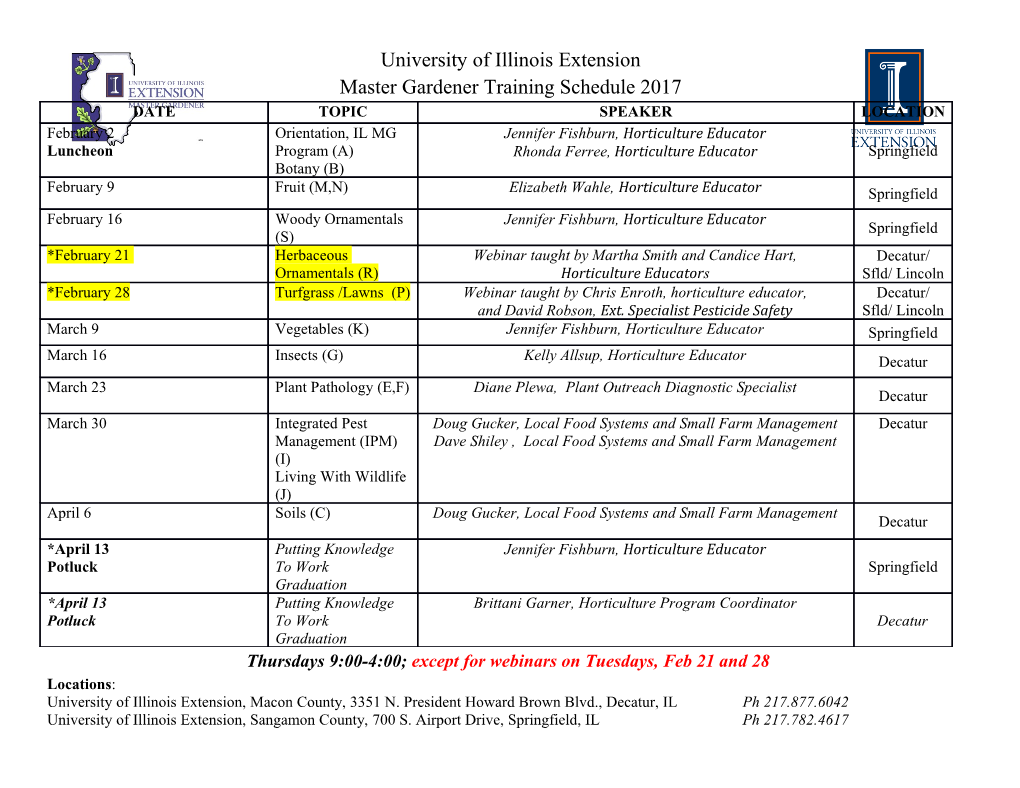
The Data Locality of Work Stealing Umut A. Acar Guy E. Blelloch Robert D. Blumofe School of Computer Science School of Computer Science Department of Computer Sciences Carnegie Mellon University Carnegie Mellon University University of Texas at Austin [email protected] [email protected] [email protected] Abstract Several researches have studied techniques to improve the data locality of multithreaded programs. One class of such techniques This paper studies the data locality of the work-stealing scheduling is based on software-controlled distribution of data among the lo- algorithm on hardware-controlled shared-memory machines. We cal memories of a distributed shared memory system [15, 22, 26]. present lower and upper bounds on the number of cache misses Another class of techniques is based on hints supplied by the pro- using work stealing, and introduce a locality-guided work-stealing grammer so that “similar” tasks might be executed on the same algorithm along with experimental validation. processor [15, 31, 34]. Both these classes of techniques rely on As a lower bound, we show that there is a family of multi- the programmer or compiler to determine the data access patterns G (n) threaded computations n each member of which requires in the program, which may be very difficult when the program has total instructions (work), for which when using work-stealing the complicated data access patterns. Perhaps the earliest class of tech- number of cache misses on one processor is constant, while even on niques was to attempt to execute threads that are close in the com- n) two processors the total number of cache misses is ( . This im- putation graph on the same processor [1, 9, 20, 23, 26, 28]. The plies that for general computations there is no useful bound relating work-stealing algorithm is the most studied of these techniques [9, multiprocessor to uninprocessor cache misses. For nested-parallel 11, 19, 20, 24, 36, 37]. Blumofe et al showed that fully-strict com- computations, however, we show that on P processors the expected putations achieve a provably good data locality [7] when executed additional number of cache misses beyond those on a single proces- with the work-stealing algorithm on a dag-consistent distributed m O (C d m e PT ) sor is bounded by 1 , where is the execution time shared memory systems. In recent work, Narlikar showed that work s C of an instruction incurring a cache miss, s is the steal time, is the stealing improves the performance of space-efficient multithreaded T size of cache, and 1 is the number of nodes on the longest chain applications by increasing the data locality [29]. None of this pre- of dependences. Based on this we give strong bounds on the total vious work, however, has studied upper or lower bounds on the running time of nested-parallel computations using work stealing. data locality of multithreaded computations executed on existing For the second part of our results, we present a locality-guided hardware-controlled shared memory systems. work stealing algorithm that improves the data locality of multi- In this paper, we present theoretical and experimental results threaded computations by allowing a thread to have an affinity for on the data locality of work stealing on hardware-controlled shared a processor. Our initial experiments on iterative data-parallel appli- memory systems (HSMSs). Our first set of results are upper and cations show that the algorithm matches the performance of static- lower bounds on the number of cache misses in multithreaded com- M (C ) partitioning under traditional work loads but improves the perform- putations executed by the work-stealing algorithm. Let 1 de- ance up to 50% over static partitioning under multiprogrammed note the number of cache misses in the uniprocessor execution and M (C ) P work loads. Furthermore, the locality-guided work stealing im- P denote the number of cache misses in a -processor ex- proves the performance of work-stealing up to 80%. ecution of a multithreaded computation by the work stealing algo- rithm on an HSMS with cache size C . Then, for a multithreaded T T 1 computation with 1 work (total number of instructions), criti- 1 Introduction cal path (longest sequence of dependences), we show the following results for the work-stealing algorithm running on a HSMS. Many of today’s parallel applications use sophisticated, adaptive algorithms which are best realized with parallel programming sys- Lower bounds on the number of cache misses for general tems that support dynamic, lightweight threads such as Cilk [8], computations: We show that there is a family of computa- T =(n) M (C )=3C Nesl [5], Hood [10], and many others [3, 16, 17, 21, 32]. The core G 1 1 tions n with such that while (C )= of these systems is a thread scheduler that balances load among the M even on two processors the number of misses 2 n) processes. In addition to a good load balance, however, good data ( . locality is essential in obtaining high performance from modern parallel systems. Upper bounds on the number of cache misses for nested- parallel computations: For a nested-parallel computation, we M M (C )+2C 1 show that P , where is the number of Permission to make digital or hard copies of part or all of this work or personal or classroom use is granted without fee provided that copies are not steals in the P -processor execution. We then show that the m O (d ePT ) m expected number of steals is 1 , where is the made or distributed for profit or commercial advantage and that copies bear s this notice and the full citation on the first page. To copy otherwise, to time for a cache miss and s is the time for a steal. republish, to post on servers, or to redistribute to lists, requires prior specific Upper bound on the execution time of nested-parallel com- permission and/or a fee. putations: We show that the expected execution time of a SPAA 2000, Bar Harbor, Maine USA © ACM 2000 1-58113-185-2/00/07...$5.00 1 linear work stealing is due to the fact that the data for each run does not 18 work-stealing 2 locality-guided workstealing 2 static partioning fit into the L cache of one processor but fits into the collective L 16 cache of 6 or more processors. For this benchmark the following 14 can be seen from the graph. 12 1. Locality-guided work stealing does significantly better than 10 standard work stealing since on each step the cache is pre- warmed with the data it needs. Speedup 8 6 2. Locality-guided work stealing does approximately as well as static partitioning for up to 14 processes. 4 2 3. When trying to schedule more than 14 processes on 14 processors static partitioning has a serious performance 0 0 5 10 15 20 25 30 35 40 45 50 Number of Processes drop. The initial drop is due to load imbalance caused by the coarse-grained partitioning. The performance then ap- proaches that of work stealing as the partitioning gets more Figure 1: The speedup obtained by three different over-relaxation fine-grained. algorithms. We are interested in the performance of work-stealing computa- tions on hardware-controlled shared memory (HSMSs). We model an HSMS as a group of identical processors each of which has its T (C ) 1 O ( + nested-parallel computation on P processors is P own cache and has a single shared memory. Each cache contains m md e CT +(m + s)T ) T (C ) 1 1 1 , where is the uniprocessor C s blocks and is managed by the memory subsystem automatically. execution time of the computation including cache misses. We allow for a variety of cache organizations and replacement poli- cies, including both direct-mapped and associative caches. We as- As in previous work [6, 9], we represent a multithreaded com- sign a server process with each processor and associate the cache putation as a directed, acyclic graph (dag) of instructions. Each of a processor with process that the processor is assigned. One lim- node in the dag represents a single instruction and the edges repre- itation of our work is that we assume that there is no false sharing. sent ordering constraints. A nested-parallel computation [5, 6] is a race-free computation that can be represented with a series-parallel dag [33]. Nested-parallel computations include computations con- 2 Related Work sisting of parallel loops and fork an joins and any nesting of them. This class includes most computations that can be expressed in As mentioned in Section 1, there are three main classes of tech- Cilk [8], and all computations that can be expressed in Nesl [5]. niques that researchers have suggested to improve the data locality Our results show that nested-parallel computations have much bet- of multithreaded programs. In the first class, the program data is ter locality characteristics under work stealing than do general com- distributed among the nodes of a distributed shared-memory system putations. We also briefly consider another class of computations, by the programmer and a thread in the computation is scheduled on computations with futures [12, 13, 14, 20, 25], and show that they the node that holds the data that the thread accesses [15, 22, 26]. can be as bad as general computations. In the second class, data-locality hints supplied by the programmer The second part of our results are on further improving the data are used in thread scheduling [15, 31, 34]. Techniques from both locality of multithreaded computations with work stealing.
Details
-
File Typepdf
-
Upload Time-
-
Content LanguagesEnglish
-
Upload UserAnonymous/Not logged-in
-
File Pages12 Page
-
File Size-