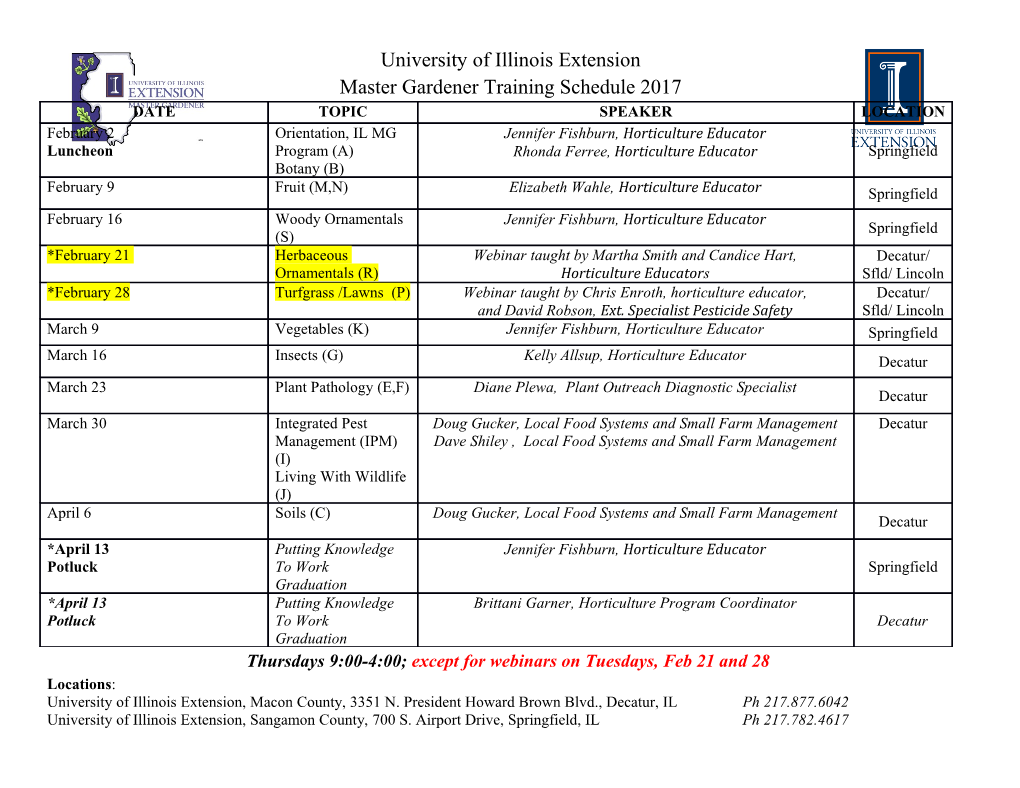
Chapter 2 Static and Dynamic Characteristics of Signals Signals Signals classified as 1. Analog – continuous in time and takes on any magnitude in range of operations 2. Discrete Time – measuring a continuous variable at finite time intervals 3. Digital – discretized magnitude at discrete times Quantization assigns a single number to represent a range of magnitudes of a continuous signal This is done by a Analog to Digital A/D converter 1 Signal Wave Form Static – does not vary with time and can be considered over long periods, like the life of a battery. Dynamic Signal – time dependent y(t) Definitions Deterministic Signal –a signal that varies in time in a predictable manner, such as a sine wave, a step function, or a ramp function. Steady Periodic –a signal whose variation of the magnitude of the signal repeats at regular intervals in time. Definitions cont’d Complex Periodic Waveform – contains multiple frequencies and is represented as a superposition of multiple simple periodic waveforms. Aperiodic Waveforms – deterministic signals that do not repeat at regular intervals (step function). Nondeterministic Signal –a signal with no discernible pattern of repetition. 2 For periodic signal: c = amplitude f = 1/T cycles/sec (hz) T = period ω = 2Πfrad/sec Signal Analysis Assume y=current t 2 t 2 Y = (())/()ytdt dt ∫∫t1 t1 If power is equal to P=I2R, then energy is dissipated in resistor from t1 to t2 Signal Analysis t 2 T 2 E ==Pdt [()]It2 Rdt ∫∫t1 T1 To find a fixed current Ie that produces some energy dissipation in time t1 to t2, 2 2 2 t t 22 t 2 E ==Pdt IRdtIRtee =−=()[()]21 t It Rdt ∫∫t1 t1 ∫t1 2 t 2 ItteRMS=−(/(1 21 )) [()]It dt ⇒ I ∫ t1 3 A time dependent analog signal can be approximated by a discrete set of N numbers over the time period from t1 to t2 y(t) {y(rδt)} r=1,2,N where δt = sampling time Mean Value For discrete time signals, approximate the mean value N yNy= (/1 )∑ i i=1 RMS N 2 yNyrms= (/1 )∑ i i=1 Signal Averaging Period The choice of time period for signal analysis depends on the type of signal For simple periodic, use time period equal to the period of the function. That gives a good representation of long term average value and RMS value. 4 Signal Averaging Period Averaging a simple periodic signal over a time period that is not exactly the period of the function can produce misleading results. However, as the averaging time period becomes long relative to the signal period, the resulting values will accurately represent the signal. Signal Averaging Period Complex wave forms and non- deterministic signals do not have an exact waveform time period because they are made up of signal components with different frequencies. Remember: f=1/T hertz Effect of Time Period Selection In this case select a sampling period that is large with respect to the longest frequency. 5 Effect of DC Offset If AC component is of primary interest, subtract out DC component, then amplify AC to get more pronounced impact from AC. Signal Amplitude and Frequency Basis: very complex signals, even non- deterministic, can be approximated by a series of sine and cosine functions (fourier analysis) Fourier Analysis is a mathematical equivalent of a prism. Signal Amplitude and Frequency “the representation of complex and non- deterministic waveforms by a simple periodic function allows measurement system response to be reasonably well defined by examining the output resulting from a few specific input waveforms, one of which is the simple periodic.” 6 How do you represent a complex signal by simple function? The fundamental concepts of frequency and amplitude can be understood through the observation and analysis of periodic motions. Sines and cosines can be thought of as mathematical functions that describe specific physical behaviors of a system. Consider the mechanical vibration of a spring-mass system Spring-Mass System Governing Equation: M(d2y/dt2)+ky=0 General form of solution: y=A cos wt+ B sin wt ; ω= km/ T= time of one cycle, called a period f= 1/T hz w= 2Πfrad/sec 7 Frequency Analysis The solution can be rewritten by use of phase angle: y=c cos (wt-ϕ) or y=c sin (wt+ϕ*) Where caB=+22; ϕ=tan-1(B/A); ϕ*=Π/2 - ϕ Frequency Analysis Frequency Analysis: most signals from measurement systems are non-deterministic, having complex wave forms and consequentially can be broken into series of sine and cosine functions. The representation of a signal by an infinite series of sines and cosines is called a fourier series. In theory, all mathematical functions can be represented by a fourier series. Definitions: 1. Y(t) is a periodic function if there is a positive number T y(t+T)=y(t) -If both y1(t), and y2(t) have period T then ay1 + by2 have period T 2. A trigonometric series is given by: Ao + A1 cos t + B1 sin t +A2 cos 2t + B2 sin 2t +…+ 1 where An and Bn are coefficients 8 Example of harmonics of a string plucked at center: Fourier Series and Coefficients A periodic function∞ y(t) with period T=2Π given as yt()=+ Aon∑ ( A cos nt + B n sin nt ) n=1 With y(t) known, determine Ao, An, Bn Integrate y(t) from -Π to Π πππ ∞ π ∫∫∫ydtAodt((t)cossin)= ++∑ An ntdt Bn ∫ntdt −π −π n=1 −−π π Since π π cosntdt = 0 and sinntdt = 0 ∫ − π ∫ − π Euler’s formula: π Aytdto = 12/(π ) ( ) ∫ − π π A = 1/π y(tntdt )cos Follows thatn ∫ −π and π B = 1/()sinπ yntdtt n ∫ −π However,most functions do not have a period of 2Π that will be encountered. 9 Fourier Coefficients for Functions Having Arbitrary Periods Coefficients representing a function of frequency ω are given by: T / 2 ATytdto = 1/() ∫ − T / 2 T / 2 ATytnwtdtn = (/)2 ()cos ∫ − T / 2 T / 2 BTytnwtdtn = (/)2 ()sin ∫ − T / 2 Where n = 1,2,3,…, and T = 2π/ω Fourier Coefficients for Functions Having Arbitrary Periods The trigonometric series that results from these coefficients is a Fourier series and may be written as: ∞ y(t) = A0 + ∑(An cosnωt + Bn sinnωt) n=1 Fourier Coefficients for functions having Arbitrary Periods ∞ The series yt()=+ Aon∑ (An cosnwt + B sin nwt ) can n=1 be rewritten using phase angles as ∞ yt( )=+ AonC cos(nwt −φ ) 22 ∑ n Where CABn = nn n=1 tanϕ nnn= B / A ∞ tanϕ * nnn= AB / + * yt()=+ Aon∑Cn sin(nwt φ ) n=1 10 Even and Odd Functions A function g(t) is even if it is symmetric about the origin for all t, g(-t) = g(t) A function h(t) is odd if, for all t, h(-t) = -h(t) Fourier Coefficients for functions having Arbitrary Periods If the signal is even, Bn=O and the series becomes: ∞ ∞ yt()= ∑ An cosnωt yt()= ∑ An cos(( n2π t )/ T ) n=1 n=1 If the signal is odd, An=0 and series becomes: ∞ ∞ yt()= ∑ Bn sinnωt yt()= ∑ Bn sin(( n2π t )/ T ) n=1 n=1 Example 2.5: Original: E(t)=120 sin 120Πt T=1/60sec Rectified: E(t)=⏐120 sin 120Πt⏐ T /2 1/ 120 ATytdto ==2[( 1 / ) ( ) 2 / ( 1 / 60 ) 120 sin 120π tdt = 76 . 4 ∫∫0 0 11 T / 2 ATytntTdtn = (/)42 ()cos((π )/) ∫ 0 1/ 120 = 4/( 1 / 60 ) 120 sin 120ππtntdt cos 120 ∫ 0 For values of n that are odd, the coefficient An is identically zero. For values of n that are even, the result is 120 − 2 2 An = ( + ) π n − 1 n + 1 The Fourier series for the function |120 sin 120πt| is 76.4 – 50.93 cos 240πt – 10.10 cos 480πt – 4.37 cos 720πt The frequency content of a signal is found by examining the amplitude of various frequency components, and by expanding the fourier series and plotting amplitudes of sine and cosine terms. 12 Fourier Transform In Fourier Series analysis we worked with arbitrary but known signals. The coefficients of the FS specified the amplitude of the sine and cosines having a specific frequency. In practical applications, we may not know anything about the form or frequency of the signal. We need a method to decompose signals in terms of their frequency components. Fourier Transform From earlier work: ∞ yt()=+ Aon∑ ( A cosnωt +B n sinnωt ) n=1 T / 2 ATytn = (/)2 ()cosnωtdt ∫ −T / 2 T / 2 BTytn = (/)2 ()sinnωtdt ∫ −T / 2 ω = 2πf Assumption: We remove the constraint that the signal be a periodic function, and let the period go to infinity. The coefficients become continuous functions of frequency. ∞ ω = ωtdt A()∫ −∞yt ()cos ∞ B()ω = yt ()sinωtdt ∫ −∞ 13 Assumption The fourier coefficients of A(ω) and B(ω) are components of fourier transforms of y(t) Υ()ω =−Aw () iB (ω) Then substituting ∞ Υ(ω )=−yt ( )(cosωt i sinωt )dt ∫ −∞ − iθ Using identity ei= cosθ − sinθ ∞ leads to Υ()ω = yte ()− iωt dt (Eq 2.29) ∫ −∞ Substituting for cyclic frequency F=w/2π=1/T (Eq 2.30) ∞ Υ ()fytedt= ()− ift2π ∫ −∞ These are the two-sided fourier transforms of y(t) If we know the amplitude-frequency properties of a signal (fourier transform), the inverse-FT will reconstruct y(t). ∞ ift2π yt()= (12 /π ) Yf ( ) e dt ∫ −∞ Y(f) describes the signal as a continuous function of frequency. If y(t) is known or measured, then its fourier transform provides the amplitude-frequency properties of the signal that are not apparent from the time-based signal. 14 The fourier transform is a complex number ΥΥ()fAfiBf=− () () = () feifθ () The magnitude is given by 2 2 ΥΥΙΥ()fff=+ Re[()]M [()] Phase shift − 1 θ ()fff= tan(([()])/(Re[()]))ΙΥM Υ *Amplitude spectrum: Cf()=+ Af ()2 Bf ()2 *Phase shift: θ ()fBfAf= tan(())/(())− 1 Power spectrum: (())/Cf2 2 The Fourier transform has provided a method to decompose a measured signal y(t) into its amplitude-frequency components.
Details
-
File Typepdf
-
Upload Time-
-
Content LanguagesEnglish
-
Upload UserAnonymous/Not logged-in
-
File Pages17 Page
-
File Size-