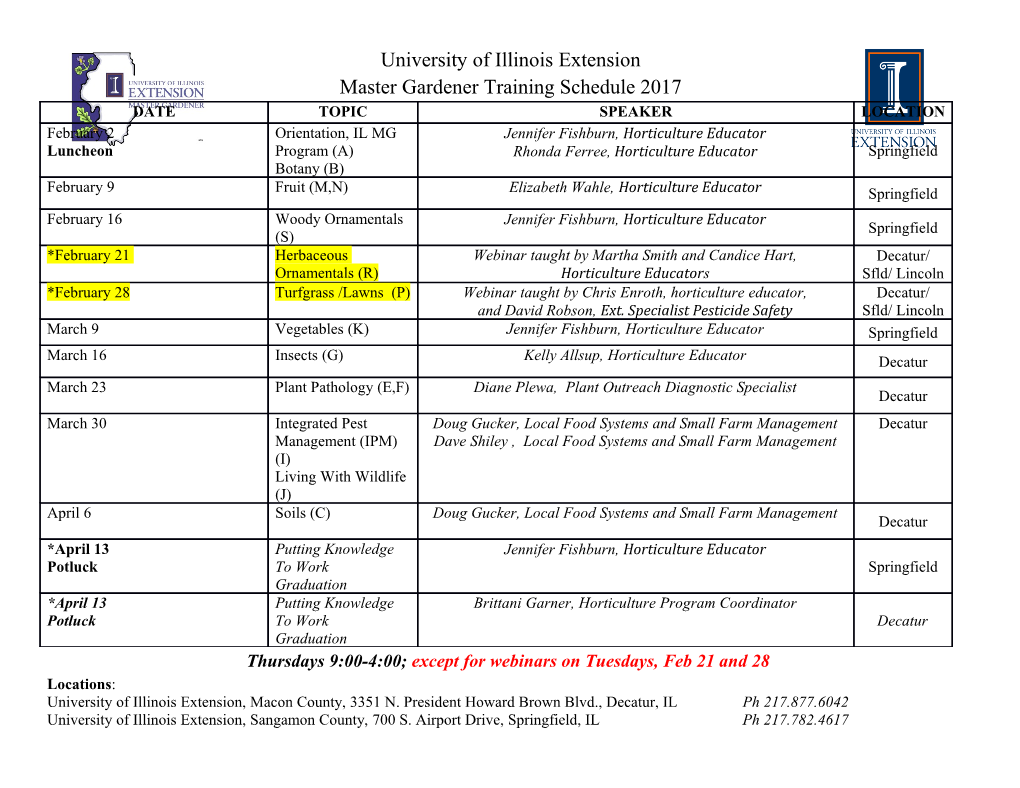
Ehsani et al. BMC Bioinformatics (2016) 17:459 DOI 10.1186/s12859-016-1349-2 RESEARCHARTICLE Open Access Feature-based classification of human transcription factors into hypothetical sub- classes related to regulatory function Rezvan Ehsani1,2†, Shahram Bahrami1,3† and Finn Drabløs1* Abstract Background: Transcription factors are key proteins in the regulation of gene transcription. An important step in this process is the opening of chromatin in order to make genomic regions available for transcription. Data on DNase I hypersensitivity has previously been used to label a subset of transcription factors as Pioneers, Settlers and Migrants to describe their potential role in this process. These labels represent an interesting hypothesis on gene regulation and possibly a useful approach for data analysis, and therefore we wanted to expand the set of labeled transcription factors to include as many known factors as possible. We have used a well-annotated dataset of 1175 transcription factors as input to supervised machine learning methods, using the subset with previously assigned labels as training set. We then used the final classifier to label the additional transcription factors according to their potential role as Pioneers, Settlers and Migrants. The full set of labeled transcription factors was used to investigate associated properties and functions of each class, including an analysis of interaction data for transcription factors based on DNA co-binding and protein-protein interactions. We also used the assigned labels to analyze a previously published set of gene lists associated with a time course experiment on cell differentiation. Results: The analysis showed that the classification of transcription factors with respect to their potential role in chromatin opening largely was determined by how they bind to DNA. Each subclass of transcription factors was enriched for properties that seemed to characterize the subclass relative to its role in gene regulation, with very general functions for Pioneers, whereas Migrants to a larger extent were associated with specific processes. Further analysis showed that the expanded classification is a useful resource for analyzing other datasets on transcription factors with respect to their potential role in gene regulation. The analysis of transcription factor interaction data showed complementary differences between the subclasses, where transcription factors labeled as Pioneers often interact with other transcription factors through DNA co-binding, whereas Migrants to a larger extent use protein-protein interactions. The analysis of time course data on cell differentiation indicated a shift in the regulatory program associated with Pioneer-like transcription factors during differentiation. Conclusions: The expanded classification is an interesting resource for analyzing data on gene regulation, as illustrated here on transcription factor interaction data and data from a time course experiment. The potential regulatory function of transcription factors seems largely to be determined by how they bind DNA, but is also influenced by how they interact with each other through cooperativity and protein-protein interactions. Keywords: Transcription factors, Chromatin opening, Machine learning, Classification * Correspondence: [email protected] †Equal contributors 1Department of Cancer Research and Molecular Medicine, Norwegian University of Science and Technology, PO Box 8905NO-7491 Trondheim, Norway Full list of author information is available at the end of the article © The Author(s). 2016 Open Access This article is distributed under the terms of the Creative Commons Attribution 4.0 International License (http://creativecommons.org/licenses/by/4.0/), which permits unrestricted use, distribution, and reproduction in any medium, provided you give appropriate credit to the original author(s) and the source, provide a link to the Creative Commons license, and indicate if changes were made. The Creative Commons Public Domain Dedication waiver (http://creativecommons.org/publicdomain/zero/1.0/) applies to the data made available in this article, unless otherwise stated. Ehsani et al. BMC Bioinformatics (2016) 17:459 Page 2 of 16 Background TFs can also be classified based on structural proper- Cells recognize and respond to internal and external sig- ties, and the most common classifications are based on nals, often leading to changes in the transcription level of the structure of their DNA-binding domains (DBDs) specific genes. Transcriptional regulatory systems play a [12]. In some instances the structural classification may key role in many biological processes, such as cell cycle also indicate the function of TFs. For example, TFs with progression, maintenance of intracellular metabolism, homeodomain are often associated with developmental physiological balance, and cellular differentiation in devel- processes, and those with a “winged” helix-turn-helix opmental time courses [1, 2]. The regulatory system for (HTH) motif are frequently associated with the inter- transcription involves several proteins, and in particular feron regulatory factor family and triggering of immune transcription factors (TFs) can coordinate a diversity of responses against viral infections [12]. Wingender et al. regulatory processes. Many diseases arise from errors in (2013) have made a comprehensive classification of 1558 the regulatory system for transcription; TFs are overrepre- human TFs based on a hierarchy of general topology sented among oncogenes [3], and a third of human devel- (Superclass), similar structures of the DBD (Class), opmental disorders have been related to dysfunctional sequence and functional similarities (Family), sequence- TFs [4]. However, alterations in the activity and regulatory based subgroupings (Subfamily), TF gene (Genus), and pathway of TFs are also likely to be a source for pheno- TF polypeptide (Factor ‘species’), and this classification typic diversity and evolutionary adaptation [5–7]. is known as TFClass. TFs are classified according to this Most TFs bind to DNA by recognizing specific DNA six-level classification scheme, where four levels are ab- sub-sequences known as transcription factor binding stractions according to different criteria, while the fifth sites (TFBSs), and thereby they control the transcription level shows the TF genes, and the sixth level individual of nearby genes through their promoters, or more dis- gene products. They collected and curated 71 animal TF tant genes through enhancers. However, it has been real- families. Altogether, ten superclasses have been identi- ized that binding of TFs to TFBSs is not enough to fully fied, comprising of 40 classes and 111 families [13]. explain the regulatory program of gene expression [8]. In a previous paper we presented a comprehensive list The set of cis-regulatory regions (promoters, enhancers) of properties for 1978 human TFs. We identified 1225 is identical at the DNA sequence level in all cell types of DNA binding TFs, based on existing annotation of Pfam a given species. Therefore the transcriptional program domains and identification of additional Pfam DBDs. For specific to each cell type must be the result of the set of the remaining 753 TFs we could not identify a clear DBD. TFs expressed in that cell type, and how genes are Annotated properties included DBDs, protein–protein in- selected for transcriptional activation or repression. The teractions, and post-translational modifications. The paper same TFs can be expressed at the same rate in different demonstrated how such a resource can be used to identify cell types, but may have separate binding sites, as TF properties that are enriched in a subset of TFs [14]. How- function and regulatory pathways also depend upon ever, it is an interesting question whether TF properties chromatin structure and epigenetic modifications [9]. also can be used to predict the regulatory function of TFs, TFs can be classified according to regulatory function. for example as defined by Sherwood et al. This is particu- Sherwood et al. (2014) introduced PIQ (protein interaction larly relevant as Sherwood et al. could assign regulatory quantitation), a computational method for modeling the function only to a subset of the known TFs. magnitudeandshapeofgenome-wideDNaseIhypersensi- There are several different types of classifiers from ma- tivity profiles used for identification of transcription factor chine learning that may be used for this type of function binding sites [10]. They identified binding sites for more prediction, as for example Random Forest (RF), Support than 700 transcription factors from an experiment using Vector Classifier (SVC) with different kernels (e.g. linear, DNase I hypersensitivity analysis followed by sequencing, Radial Basis Function (RBF), polynomial), k Nearest and used the data for a hypothetical classification of tran- Neighbours (kNN), and Gaussian naïve Bayes (GNB) scription factors into three groups; Pioneers, Settlers and classifiers. There are also several approaches for improv- Migrants. Pioneer TFs are assumed to be distinguished by ing classifier performance, like boosting, where an their ability to bind to DNA target sites, even in inaccess- ensemble of possibly weak classifiers as combined into a ible regions, and were found bound to chromatin before stronger classifier, and AdaBoost [15] is an important activation of enhancers and gene expression modulation. implementation of boosting. The binding of Settler TFs seems to
Details
-
File Typepdf
-
Upload Time-
-
Content LanguagesEnglish
-
Upload UserAnonymous/Not logged-in
-
File Pages16 Page
-
File Size-