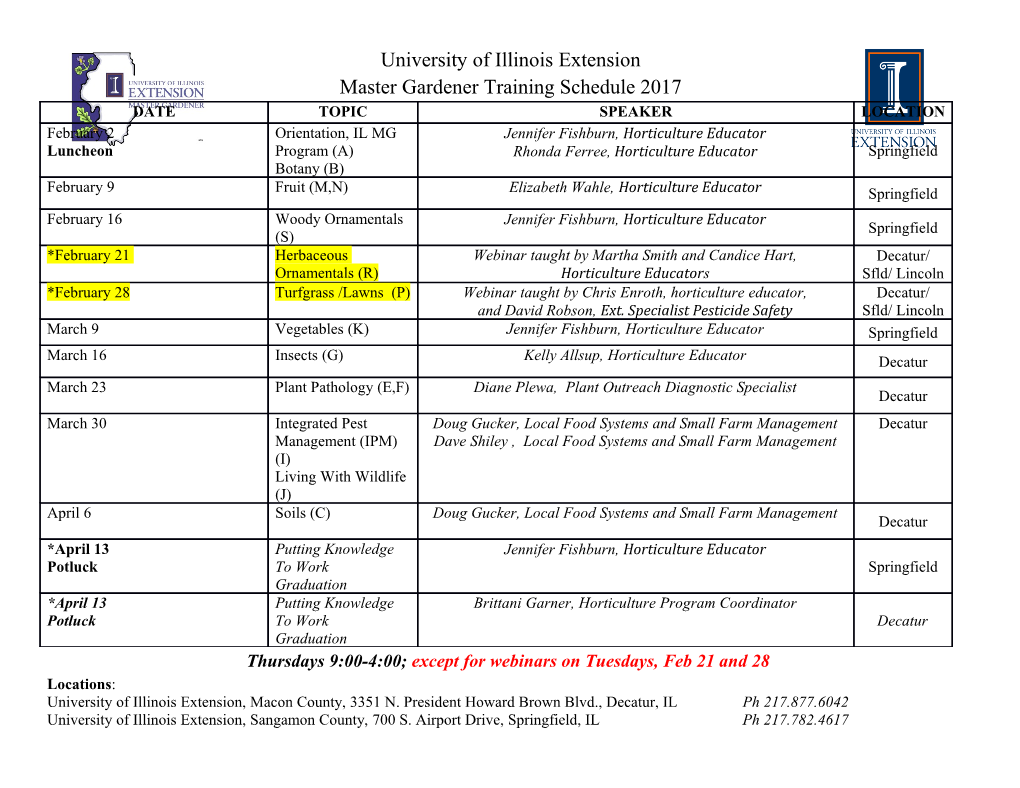
MATH 544 | HOMEWORK V JAKOB STREIPEL Problem 4.1.5 Let A 2 Rn×m be nonzero with rank r. Show that all of the equations ( ( σiui i = 1; 2; : : : ; r T σivi i = 1; 2; : : : ; r Avi = A ui = 0 i = r + 1; r + 2; : : : ; m 0 i = r + 1; r + 2; : : : ; n follow from the singular value decomposition A = UΣV T (and its transpose), where u1; u2; : : : ; un and v1; v2; : : : ; vm are the columns of U and V , respectively. Solution. By construction of A = UΣV T , where V is an orthogonal matrix, meaning V T V = I, we have AV = UΣ: n×m Again by construction Σ = diag(σ1; σ2; : : : ; σr; 0;::: 0) 2 R and σ1 ≥ σ2 ≥ ::: ≥ σr > 0. Hence writing U in terms of its columns U = u1 u2 ··· un we have 2 3 σ1 6 σ2 7 6 7 6 .. 7 6 . 7 UΣ = u1 u2 ··· un 6 7 6 σr 7 6 7 6 0 7 4 . 5 .. = σ1u1 σ2u2 ··· σrur 0ur+1 ··· 0rn = σ1u1 σ2u2 ··· σrur 0 ··· 0 : Writing the left-hand side AV in terms of the columns of V we similarly have AV = Av1 Av2 ··· Avm : Since these are equal, comparing the columns we have precisely ( σiui i = 1; 2; : : : ; r Avi = 0 i = r + 1; r + 2; : : : ; m; as claimed. By transposing A = UΣV T we get AT = (UΣV T ) = (V T )T ΣT U T = V ΣT U T ; Date: April 2, 2018. 1 2 JAKOB STREIPEL whereby, since U T U = I because it's orthogonal, we get AT U = V ΣT : By essentially the same argument as above on this new equation we get T T T T A U = A u1 A u2 ··· A un T = σ1v1 σ2v2 ··· σrvr 0 ··· 0 = V Σ which by comparing columns tells us ( T σivi i = 1; 2; : : : ; r A ui = 0 i = r + 1; r + 2; : : : ; n; as desired. Problem 4.2.3 Recall that the Frobenius matrix norm is defined by n m 1=2 X X 2 kAkF = jaijj : i=1 j=1 2 2 2 1=2 Show that kAkF = (σ1 + σ2 + ::: + σr ) . Solution. Note that as described, A 2 Rn×m. We start by showing that the Frobe- nius norm is equal to q T kAkF = tr(A A) where by tr B we mean the trace of a square matrix B 2 Rm×m, i.e. the sum of the diagonal elements, m X tr B = Bii: i=1 Hence since the diagonal elements of AT A are n n n T X X 2 X 2 (A A)ii = ajiaji = aji = jajij j=1 j=1 j=1 we have m m n T X T X X 2 tr(A A) = (A A)ii = jajij : i=1 i=1 j=1 Switching the order of the sums we get n m T X X 2 tr(A A) = jajij j=1 i=1 and switching the names of the dummy variables i and j we get n m T X X 2 2 tr(A A) = jaijj = kAkF i=1 j=1 by definition. Since these are sums of nonnegative numbers, we can take square roots of both sides. With this expression of the Frobenius norm in hand, suppose B = UC where U is an orthogonal matrix. Then q q q T T T T kBkF = kUCkF = tr((UC) (UC)) = tr(C U UC) = tr(C C) = kCkF MATH 544 | HOMEWORK V 3 since U being orthogonal means U T U = I. Similarly multiplication by an orthog- onal matrix from the right does not change the Frobenius norm since q q T T kCUkF = tr((CU) (CU)) = tr((CU)(CU) ) q q q T T T T = tr(CUU C ) = tr(CC ) = tr(C C) = kCkF where we have twice invoked the fact that tr(AB) = tr(BA) for any two (com- patible) matrices, which follows by the same reversal and relabelling of the sums defining the trace as we used above. T Hence since A = UΣV where Σ = diag(σ1; σ2; : : : ; σr; 0;:::; 0) and U and V are orthogonal, we have that AV = UΣ, we have q T 2 2 2 kAkF = kUΣV kF = kUΣkF = kΣkF = σ1 + σ2 + ::: + σr : Problem 4.2.8 MATLAB's command cond computes the condition number κ2(A). This works for both square and nonsquare matrices. Generate a random 3×3 matrix (A = randn(3)) and use MATLAB to compute κ2(A) three different ways: (i) using cond, (ii) taking the ratio of largest to smallest singular value, and −1 (iii) computing kAk2kA k2 (norm(A)* norm(inv(A))). Solution. Running A = randn(3) we acquire the matrix 2 0:2323 −0:2365 2:22943 A = 4 0:4264 2:0237 0:33765 −0:3728 −2:2584 1:0001 (rounded to four decimals, at any rate) on which we will perform the subsequent computations. (i) Executing cond(A) returns κ2(A) = 76:530628562477673. The reason we print the above to so many decimal places will be apparent in part (iii). (ii) The MATLAB command s = svd(A) returns a list of the singular values of A in descending order. Hence s(1) / s(end) computes κ2 = σ1/σr = 76:530628562477673; which is precisely what we got above. This is not very surprising since help cond specifies that cond computes κ2 precisely as the ratio of the largest and smallest singular values as given by svd. (iii) Finally norm(A)* norm(inv(A)) returns −1 κ2 = kAk2kA k2 = 76:530628562477744; which differs from the previous two in the thirteenth decimal. Note for the record that on out computer, as measured by tic and toc, the former two are also slightly faster than the last option on the matrix at hand. Theorem 4.2.11 Let A 2 Rn×m, n ≥ m, rank(A) = m, with singular values σ1 ≥ σ2 ≥ ::: ≥ σm > 0. Then T −1 −2 T −1 T −1 k(A A) k2 = σm ; k(A A) A k2 = σm ; T −1 −1 T −1 T kA(A A) k2 = σm ; and kA(A A) A k2 = 1: 4 JAKOB STREIPEL Problem 4.2.12 Let A be as in Theorem 4.2.11 with singular value decomposi- tion A = UΣV T . (a) Determine the singular value decompositions of the matrices (AT A)−1; (AT A)−1AT ;A(AT A)−1; and A(AT A)−1AT in terms of the singular value decomposition of A. Use the orthogonality of U and V whenever possible. Pay attention to the dimensions of the various matrices. (b) Use the results of part (a) to prove Theorem 4.2.11. Solution. Note that by assumption A is full rank, whereby AT A is indeed invertible, making the expressions we're after well-defined. (a) Let us first of all understand AT A. Since A = UΣV T , with U and V by definition being orthogonal, we have AT = (UΣV T )T = (V T )T ΣT U T = V ΣT U T as the singular value decomposition of AT , with ΣT looking very much like Σ, except that the extra block of zeros is to the right of the diagonal block, rather than below it. This importantly means that T 2 2 2 m×m Σ Σ = diag(σ1; σ2; : : : ; σm) 2 R which means that AT A = V ΣT U T UΣV T = V (ΣT Σ)V T is the singular value decomposition of AT A. Now since ΣT Σ is a diagonal square matrix with nonzero diagonal elements, it's invertible. In particular T −1 −2 −2 −2 m×m (Σ Σ) = diag(σ1 ; σ2 ; : : : ; σm ) 2 R Hence (AT A)−1 = (V ΣT ΣV T ) = (V T )−1(ΣT Σ)−1V −1 = V (ΣT Σ)−1V T : Now this is not quite the singular value decomposition of (AT A)−1 since the singular values are currently in ascending order, as opposed to descending. We therefore let P be the m × m matrix with 1s on the anti-diagonal and 0s elsewhere|which is clearly orthogonal; P T = P and P T P = P 2 = I|in the following way (AT A)−1 = VPP (ΣT Σ)−1PPV T = (VP )(P (ΣT Σ)−1P )(VP )T where T −1 −2 −2 −2 P (Σ Σ) P = diag(σm ; σm−1; : : : ; σ1 ) and VP is orthogonal since both V and P are orthogonal. This, therefore, is the de- sired singular value decomposition, since the singular values are now in descending order. Multiplying this by AT from the right we get (AT A)−1AT = (V (ΣT Σ)−1V T )(V ΣT U T ) = V (ΣT Σ)−1ΣT U T ; where T −1 T −1 −1 −1 m×n (Σ Σ) Σ = diag(σ1 ; σ2 ; : : : ; σm ) 2 R : MATH 544 | HOMEWORK V 5 Hence we again need to adjust the order of the diagonal elements by the same multiplication by P above, i.e. (AT A)−1AT = (VP )(P (ΣT Σ)−1ΣT P )(UP )T is the singular value decomposition of (AT A)−1AT . Similarly if we multiply (AT A)−1 from the left by A we get A(AT A)−1 = (UΣV T )(V (ΣT Σ)−1V T ) = UΣ(ΣT Σ)−1V T where T −1 −1 −1 −1 n×m Σ(Σ Σ) = diag(σ1 ; σ2 ; : : : ; σm ) 2 R and so once more we adjust the order of the singular values and get A(AT A)−1 = (UP )(P Σ(ΣT Σ)−1P )(VP )T as the singular value decomposition of A(AT A)−1. Finally for A(AT A)−1AT we have A(AT A)−1AT = (UΣ(ΣT Σ)−1V T )(V (ΣT Σ)V T ) = UΣ(ΣT Σ)−1ΣT V T where T −1 T −1 −1 −1 n×n Σ(Σ Σ) Σ = diag(σ1 ; σ2 ; : : : ; σm ) diag(σ1; σ2; : : : ; σm) = I 2 R : Hence A(AT A)−1AT = UIV T is the singular value decomposition of A(AT A)−1AT .
Details
-
File Typepdf
-
Upload Time-
-
Content LanguagesEnglish
-
Upload UserAnonymous/Not logged-in
-
File Pages9 Page
-
File Size-