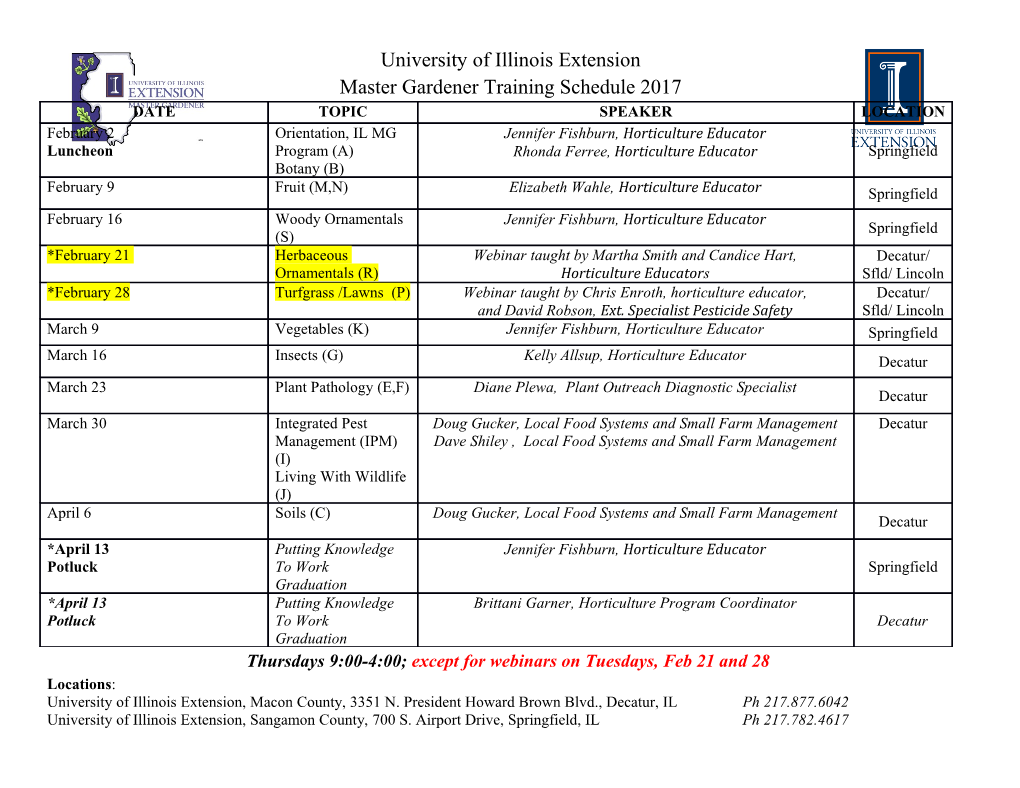
Gradient Play in n-Cluster Games with Zero-Order Information Tatiana Tatarenko, Jan Zimmermann, Jurgen¨ Adamy Abstract— We study a distributed approach for seeking a models, each agent intends to find a strategy to achieve a Nash equilibrium in n-cluster games with strictly monotone Nash equilibrium in the resulting n-cluster game, which is mappings. Each player within each cluster has access to the a stable state that minimizes the cluster’s cost functions in current value of her own smooth local cost function estimated by a zero-order oracle at some query point. We assume the response to the actions of the agents from other clusters. agents to be able to communicate with their neighbors in Continuous time algorithms for the distributed Nash equi- the same cluster over some undirected graph. The goal of libria seeking problem in multi-cluster games were proposed the agents in the cluster is to minimize their collective cost. in [17]–[19]. The paper [17] solves an unconstrained multi- This cost depends, however, on actions of agents from other cluster game by using gradient-based algorithms, whereas the clusters. Thus, a game between the clusters is to be solved. We present a distributed gradient play algorithm for determining works [18] and [19] propose a gradient-free algorithm, based a Nash equilibrium in this game. The algorithm takes into on zero-order information, for seeking Nash and generalized account the communication settings and zero-order information Nash equilibria respectively. In discrete time domain, the under consideration. We prove almost sure convergence of this work [5] presents a leader-follower based algorithm, which algorithm to a Nash equilibrium given appropriate estimations can solve unconstrained multi-cluster games in linear time. of the local cost functions’ gradients. The authors in [20] extend this result to the case of leaderless I. INTRODUCTION architecture. Both papers [5], [20] prove linear convergence in games with strongly monotone mappings and first-order Distributed optimization and game theory provide power- information, meaning that agents can calculate gradients ful frameworks to deal with optimization problems arising of their cost functions and use this information to update in multi-agent systems. In generic distributed optimization their states. In contrast to that, the work [16] deals with problems, the cost functions of agents are distributed across a gradient-free approach to the cluster games. However, the network, meaning that each agent has only partial infor- the gradient estimations are constructed in such a way that mation about the whole optimization problem which is to only convergence to a neighborhood of the equilibrium can be solved. Game theoretic problems arise in such networks be guaranteed. Moreover, these estimations are obtained by when the agents do not cooperate with each other and the using two query points, for which an extra coordination cost functions of these non-cooperative agents are coupled by between the agents is required. the decisions of all agents in the system. The applications Motivated by relevancy of n-cluster game models in of game theoretic and distributed optimization approaches many engineering applications, we present a discrete time include, for example, electricity markets, power systems, distributed procedure to seek Nash equilibria in n-cluster flow control problems and communication networks [6], [11], games with zero-order information. We consider settings, [12]. where agents can communicate with their direct neighbors On the other hand, cooperation and competition coex- within the corresponding cluster over some undirected graph. ists in many practical situations, such as cloud computing, However, in many practical situations the agents do not hierarchical optimization in Smart Grid, and adversarial know the functional form of their objectives and can only networks [3], [4], [8]. A body of recent work has been access the current values of their objective functions at some arXiv:2107.12648v1 [cs.GT] 27 Jul 2021 devoted to analysis of non-cooperative games and distributed query point. Such situations arise, for example, in electricity optimization problems in terms of a single model called n- markets with unknown price functions [15]. In such cases, cluster games [5], [16]–[20]. In such n-cluster games, each the information structure is referred to as zero-order oracle. cluster corresponds to a player whose goal is to minimize Our work focuses on zero-order oracle information settings her own cost function. However, the clusters in this game and, thus, assumes agents to have no access to the analytical are not the actual decision-makers as the optimization of the form of their cost functions and gradients. The agents instead cluster’s objective is controlled by the agents belonging to construct their local query points and get the corresponding the corresponding cluster. Each of such agents has her own cost values from the oracle. Based on these values, the local cost function, which is available only to this agent, agents estimate their local gradients to be able to follow but depends on the joint actions of agents in all clusters. the step in the gradient play procedure. We formulate the The cluster’s objective, in turn, is the sum of the local cost sufficient conditions and provide some concrete example on functions of the agents within the cluster. Therefore, in such how to estimate the gradients to guarantee the almost sure convergence of the resulting algorithm to Nash equilibria in The authors are with Control Methods and Robotics Lab at the TU Darmstadt, Germany. n-cluster games with strictly monotone game mappings. To the best of our knowledge, we present the first algorithm solving n-cluster games with zero-order oracle and the clusters. Instead, we consider the following zero-order infor- corresponding one-point gradient estimations. mation structure in the system: No agent has access to the The paper is organized as follows. In SectionII we for- analytical form of any cost function, including its own. Each mulated the n-cluster game with undirected communication agent can only observe the value of its local cost function topology in each cluster and zero-order oracle information. given any joint action of all agents in the system. Formally, Section III introduces the gradient play algorithm which is given a joint action x Ω, each agent j [ni], i [n] j 2 2 2 based on the one-point gradient estimations. The convergence receives the value Ji (x) from a zero-order oracle. Especially, result is presented in Section III as well. SectionIV provides no agent has or receives any information about the gradient. an example of query points and gradient estimations which Let us denote the game between the clusters introduced guarantee convergence of the algorithm discussed in Sec- above by Γ(n; J ; Ω ; ). We make the following f ig f ig fGig tion III. SectionV presents some simulation results. Finally, assumptions regarding the game Γ: SectionVI concludes the paper. Assumption 1. The n-cluster game under consideration is Notations. The set 1; : : : ; n is denoted by [n]. For any strictly convex. Namely, for all , the set is convex, f gn @f(x) i [n] Ωi function f : K R, K R , if(x) = is the 2 ! ⊆ r @xi the cost function Ji(xi; x i) is continuously differentiable in partial derivative taken in respect to the ith coordinate of the for each fixed . Moreover,− the game mapping, which n xi x i vector variable x R . We consider real normed space E, is defined as − which is the space2 of real vectors, i.e. E = n. We use (u; v) R T to denote the inner product in E. We use to denote the F(x) , [ 1J1(x1; x 1);:::; nJn(xn; x n)] (1) k·k r − r − Euclidean norm induced by the standard dot product in E. is strictly monotone on Ω. Any mapping g : E E is said to be strictly monotone on ! j Q E, if (g(u) g(v); u v) > 0 for any u; v Q, where Assumption 2. Each function iJi (xi; x i) is Lipschitz ⊆ − − 2 continuous on Ω. r − u = v. We use Br(p) to denote the ball of the radius r 0 6 ≥ and the center p E and to denote the unit sphere with i S Assumption 3. The action sets Ωj, j [ni], i [n], are the center in 0 E2. We use v to denote the projection 2 2 2 PΩ f g compact. Moreover, for each i there exists a so called safety of v E to a set Ω E. The mathematical expectation 2 2 ⊆ ball Br(p) Ωi with ri > 0 and pi Ωi . of a random value ξ is denoted by E ξ . We use the big-O ⊆ 2 f g The assumptions above are standard in the literature on notation, that is, the function f(x): R R is O(g(x)) as ! f(x) both game-theoretic and zero-order optimization [1]. Finally, x a, f(x) = O(g(x)) as x a, if limx a jg(x)j K for some! positive constant K. ! ! j j ≤ we make the following assumption on the communication graph, which guarantees sufficient information ”mixing” in II. NASH EQUILIBRIUM SEEKING the network within each cluster. A. Problem Formulation Assumption 4. The underlying undirected communication We consider a non-cooperative game between n clusters. graph i([ni]; i) is connected for all i = 1; : : : ; n. The j G A i n n associated non-negative mixing matrix Wi = [wkj] R × Each cluster i [n] itself consists of ni agents. Let Ji 2 j 1 2 defines the weights on the undirected arcs such that wi > 0 and Ωi R denote respectively the cost function and kj ⊆ if and only if (k; j) and Pni wi = 1, k [n ].
Details
-
File Typepdf
-
Upload Time-
-
Content LanguagesEnglish
-
Upload UserAnonymous/Not logged-in
-
File Pages8 Page
-
File Size-