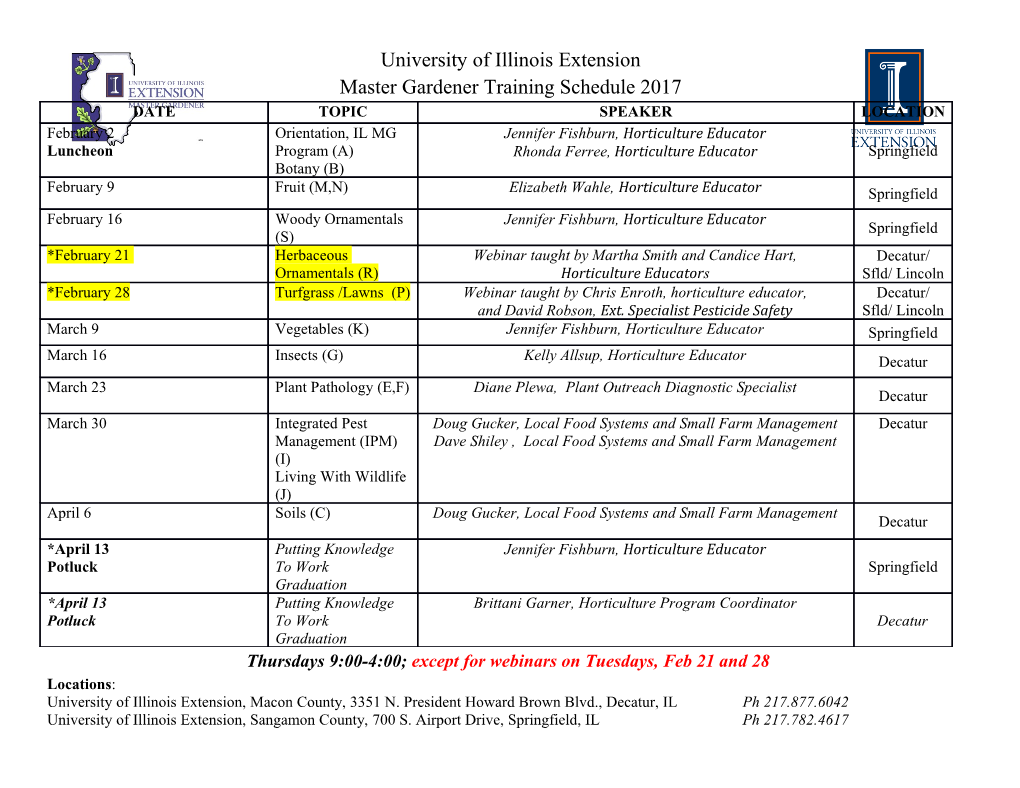
Exponential family STK3100/4100 - 21. august 2014 Plan for 2. lecture: 1. Definition exponential family 2. Examples 3. Expectation and variance 4. Likelihood and estimation Exponential family – p. 1 Definition of GLM Independent responses: Y1,Y2,...,Yn conditioned on explanatory variables Vectors of explanatory variables x1, x2,..., xn where xi =(xi1,xi2,...,xip) are p-dimensional A GLM = Generalized Linear Model is defined by • Y1,Y2,...,Yn comes from the same class of distributions from the exponential family Linear predictors η = β + β x + + β x • i 0 1 i1 ··· p ip • Link function g(): µi = E[Yi] is coupled to the linear 1 predictor by g(µi)= ηi, i.e. µi = g− (ηi) Exponential family – p. 2 Exponential family, de Jong & Heller, Ch. 3 A stochastic variable Y has a distribution belonging to the exponential family if its probability density function (pdf), or probability mass function (pmf) if Y is discrete, can be written as yθ a(θ) − f(y; θ,φ)= c(y,φ)exp( φ ) where • θ - canonical parameter • φ - dispersion parameter • The functions a(θ) and c(y,φ) is specific for each distribution The Gaussian, binomial, Poisson, gamma and other distributions can be written this way Exponential family – p. 3 Exponential distributions with φ =1 Some distributions don’t include the dispersion parameter, i.e. φ =1. Then the pdf or the pmf can be written f(y; θ)= c(y)exp(yθ a(θ)) − This includes • Poisson distribution • Distribution for binary responses, Y =1 or 0, with µ = P(Y = 1) • Binomial distribution 2 • Standard normal distribution with variance σ =1 Exponential family – p. 4 Ex: Poisson distribution, Y Po(λ) ∼ pmf: λy 1 f(y; λ)= exp( λ)= exp(y log(λ) λ), y! − y! − i.e. belonging to the exponential family with • θ = log(λ) • a(θ)= λ = exp(θ) 1 • c(y)= y! Exponential family – p. 5 Ex: Binary variable 1 with probability π Y = 0 with probability 1 π − pmf: y 1 y f(y; π)= π (1 π) − = exp(y log(π)+(1 y) log(1 π)) − − − π = exp(y log( ) + log(1 π)) 1 π − − which is on the form c(y)exp(yθ a(θ)) with − π which gives exp(θ) • θ = log( 1 π ) π = π(θ)= 1+exp(θ) − a(θ)= log(1 π(θ)) = log(1 + exp(θ)) • − − • c(y)=1 Exponential family – p. 6 Ex: Y Bin(n,π) ∼ n y n y pmf: f(y; π)= π (1 π) − y − which can be transformed to c(y)exp(yθ a(θ)) with − π exp(θ) • θ = log( 1 π ) π = 1+exp(θ) − ⇔ a(θ)= n log(1 + exp(θ)) = n log(1 π) • − n • c(y)= y Note that exp(θ) E • a′(θ)= n 1+exp(θ) = nπ = [Y ] exp(θ) a′′(θ)= n 2 = nπ(1 π)= Var[Y ] • (1+exp(θ)) − where a′(θ) and a′′(θ) are the first and second derivatives of a(θ) with respect to θ. These are general expressions for the exponential family. Exponential family – p. 7 Ex: Standard normal distribution, Y N(µ, 1) ∼ 1 1 1 µ2 y2 pdf: f(y; µ)= exp( (y µ)2)= exp(yµ ) √2π −2 − √2π − 2 − 2 2 exp( y ) µ2 = − 2 exp(yµ ) √2π − 2 which is on the form c(y)exp(yθ a(θ)) with − • θ = µ θ2 • a(θ)= 2 2 exp( y ) c(y)= − 2 • √2π Again expectation and variance are given from a(θ): • a′(θ)= θ = µ = E[Y ] a′′(θ)=1= Var[Y ] • Exponential family – p. 8 Exponential family with dispersion parameter With general φ not necessarily 1 Includes normal distribution with general σ2 Exponential family – p. 9 Ex: Y N(µ,σ2) ∼ 1 1 pdf: f(y; µ)= exp( (y µ)2) √2πσ −2σ2 − 2 2 2 y 2 1 yµ µ /2 y /2 exp( 2 ) yµ µ /2 = exp( − − )= − 2σ exp( − )) √2πσ σ2 √2πσ2 σ2 which is on the form c(y,φ) exp((yθ a(θ))/φ) with − 2 2 and µ θ • θ = µ a(θ)= 2 = 2 2 • dispersion parameter φ = σ 2 exp( y ) c(y,φ)= − 2φ • √2πφ Note that • E[Y ]= µ = θ = a′(θ) 2 Var[Y ]= σ = φ = φa′′(θ) • Exponential family – p. 10 Expectation and variance in the exponential family • E[Y ]= a′(θ) • Var[Y ]= φa′′(θ) Exponential family – p. 11 Proof for E[Y ]= a′(θ) y a′(θ) − First derivative of f: f ′(y; θ,φ)= φ f(y; θ,φ) Integral of left side ∂ ∂ f ′(y; θ,φ)dy = f(y; θ,φ)dy = (1) = 0 ∂θ ∂θ Z Z Integral of right side 1 E[Y ] a′(θ) ( yf(y; θ,φ)dy a′(θ) f(y; θ,φ)dy)= − , φ − φ Z Z which gives E[Y ]= a′(θ) Assumes that differentiation and integration can be interchanged Exponential family – p. 12 Proof for Var(Y )= φa′′(θ) 2 y a′(θ) a′′(θ) Second derivative: f ′′(y; θ,φ)= − f(y; θ,φ) φ − φ Integral of left side ∂2 ∂2 f ′′(y; θ,φ)dy = f(y; θ,φ)dy = (1) = 0 ∂θ2 ∂θ2 Z Z Integral of right side 2 y a′(θ) a′′(θ) Var(Y ) a′′(θ) − f(y; θ,φ)dy = φ − φ φ2 − φ Z " # which gives Var(Y )= φa′′(θ) Exponential family – p. 13 Ex: Poisson distribution Y Po(λ) ∼ • θ = log(λ): canonical parameter • a(θ) = exp(θ) which gives • E[Y ]= a′(θ) = exp(θ)= λ • Var[Y ]= a′′(θ) = exp(θ)= λ Exponential family – p. 14 Ex: Normal distribution N(µ,σ2) 2 and θ • θ = µ a(θ)= 2 2 • φ = σ which gives • E[Y ]= a′(θ)= θ = µ 2 • Var[Y ]= φa′′(θ)= φ = σ Exponential family – p. 15 Ex. Binomial distribution Y Bin(n,π) ∼ θ = log(π/(1 π) • − • a(θ)= n log(1 + exp(θ)) • φ =1 which gives E n exp(θ) • [Y0]= a′(θ)= 1+exp(θ) = nπ n exp(θ) Var[Y ]= φa′′(θ)= 2 = nπ(1 π)= µ(1 µ/n) • 0 (1+exp(θ)) − − Exponential family – p. 16 Variance function V (µ) Var(Y )= φa′′(θ) It is an 1-1 relationship between µ = E[Y ]= a′(θ) and θ. Therefore can we also express θ = θ(µ) as a function of µ. The variance function is V (µ)= a′′(θ(µ)) such that Var(Y )= φV (µ). For the most common distributions the expression for V (µ) is found directly. Exponential family – p. 17 Variance function for some distributions Normal distribution: a′′(θ)=1, which gives the variance function V (µ)=1 (the constant function) Poisson distribution: a′′(θ) = exp(θ)= µ, i.e. V (µ)= µ (the identity function) Binomial distribution: neθ a′′(θ)= θ 2 = nπ(1 π)= µ(1 µ/n), i.e. (1+e ) − − V (µ)= µ(1 µ/n) − Exponential family – p. 18 Other members in the exponential family • Gamma distribution, with the exponential distribution as a special case • Inverse Gaussian distribution • Negative binomial distribution, with the geometric distribution as a special case Exponential family – p. 19 Gamma distribution −1 pdf: f(y; µ,ν)= y ( yν )ν exp( yν/µ) for y > 0 Γ(ν) µ − Belongs to the exponential family with θ = 1/µ and a(θ)= log( θ) • − − − • φ =1/ν which gives • E[Y ]= a′(θ)= 1/θ = µ − 2 Var 1 µ • [Y ]= φa′′(θ)= νθ2 = ν 2 • V (µ)= µ ν =1 gives the exponential distribution pdf: f(y; µ)= 1 exp( y/µ)= λ exp( λy), where λ =1/µ µ − − Exponential family – p. 20 Inverse Gaussian distribution: Y has density, for y > 0, 1 1 (y µ)2 f(y; µ,σ2)= exp( − ) 2µy3σ2 −2y µ2σ2 where µ = E[Y ] and Varp(Y )= σ2µ3. This belongs to the exponential family with θ = µ, φ = σ2 and V (µ)= µ3. mu=5, sigma2=0.01 mu=5, sigma2=0.05 mu=5, sigma2=0.1 f(y) f(y) f(y) 0.0 0.1 0.2 0.3 0.00 0.05 0.10 0.15 0.20 0.00 0.05 0.10 0.15 0.20 0 10 20 30 40 50 60 0 10 20 30 40 50 60 0 10 20 30 40 50 60 y y y mu=20, sigma2=0.01 mu=20, sigma2=0.05 mu=20, sigma2=0.1 f(y) f(y) f(y) 0.00 0.01 0.02 0.03 0.04 0.05 0.00 0.01 0.02 0.03 0.04 0.05 0.00 0.02 0.04 0.06 Exponential family – p. 21 0 10 20 30 40 50 60 0 10 20 30 40 50 60 0 10 20 30 40 50 60 Negative binomial distribution A useful distribution for over-dispersed counts Γ(y + r) pmf: P(Y = y)= (1 p)rpy for y =0, 1, 2,... y!Γ(r) − With κ =1/r assumed known this belongs to the exponential family with µ = E[Y ]= rp/(1 p), • − • without any dispersion parameter • V (µ)= µ(1 + κµ). This distribution may arise for instance if Y λ Po(λ), | ∼ where λ is a stochastic gamma distributed variable with expectation µ and shape parameter r =1/κ. Exponential family – p. 22 Overview of some distributions in the exponential family Distrib. θ a(θ) φ E[Y ] V (µ) Bin(n,π) log( π ) log(1+ eθ) 1 µ = nπ nπ(1 π)= µ(1 µ/n) 1−π − − Po(µ) log(µ) exp(θ) 1 µ µ 2 2 θ 2 N(µ,σ ) µ 2 σ µ 1 Gamma(µ,ν) 1 log( µ) 1 µ µ2 − µ − − ν 2 1 2 3 IG(µ,σ ) 2 √ 2θ σ µ µ − 2µ − − − NB(µ,κ) log( κµ ) 1 log(1 κeθ) 1 µ µ(1 + κµ) 1+κµ κ − Exponential family – p.
Details
-
File Typepdf
-
Upload Time-
-
Content LanguagesEnglish
-
Upload UserAnonymous/Not logged-in
-
File Pages26 Page
-
File Size-