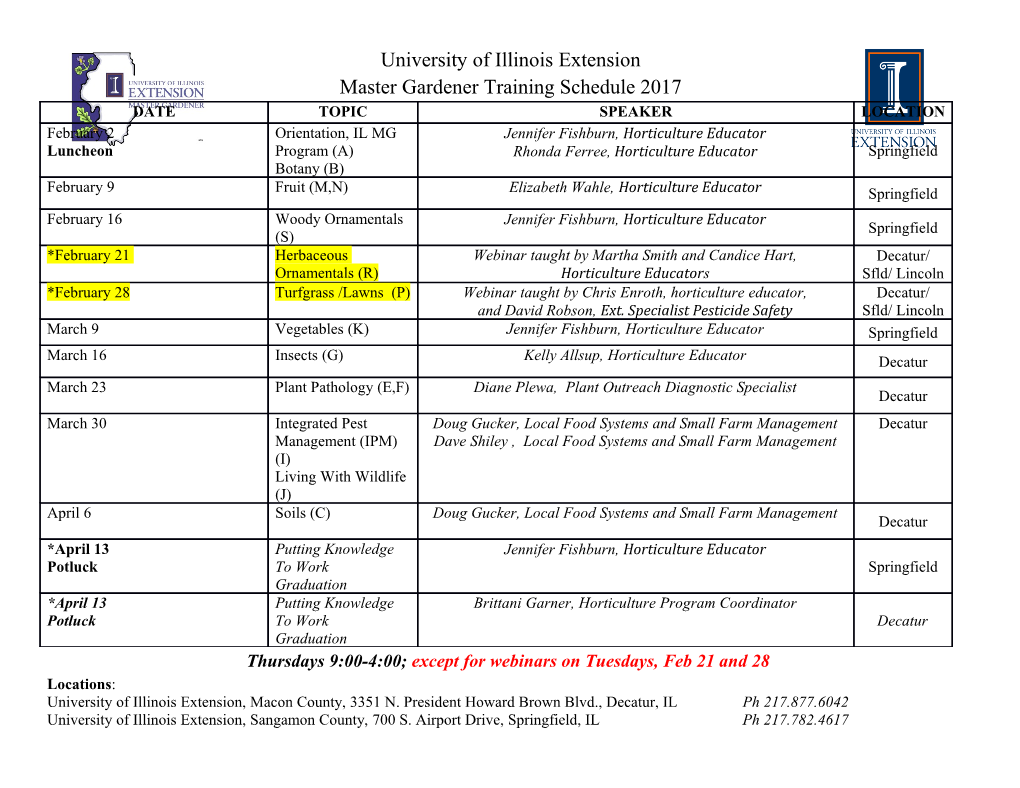
AI in Wargaming Searchlight 7th September 2019 AI/ML niche | Using sparse or qualitative data, and explaining key AI decisions Counter- DUChESS, WARDEn, AORTA C2 for Autonomy, (engagement) maritime Counter-AI Lessons Red Mirror Services, AD, ASuW, learnt (predicting Red AI) politics, ASW market DR SO research (AI swarming) MaLFIE Explainable Manning/ (anomalies) Defence AI/ML downselect SeLeREx (predicting performance) Recruitment, Housing Event/ management, repairs, mission Op Wargaming investment fraud risk RIDES, DIGAR, JSBACH planning (behaviour & workload) What Would Crisis Napoleon Do? management, and Policing Red’s Shoes (predicting ‘Red’) COMMERCIAL IN CONFIDENCE Page 2 AI Red Teaming | What Would Napoleon Do with Red’s Shoes? Actual Predicted likely Predicted worst case Georgy Zhukov 5 5) Walking in Red’s Shoes - 4 3 2 • Allows staffs to understand how the Red Outcomeindex(1 1 1 2 3 4 5 6 7 8 9 10 11 12 13 14 15 16 17 18 19 20 21 22 23 24 25 26 commander’s experience will enable them Georgy Zhukov's learning and experience events Actual Predicted likely Predicted worst case Ruslan Gelayev 5 5) to resist elements of Blue’s plan (or not) - 4 3 2 Outcomeindex(1 1 What would Napoleon do? 1 2 3 4 5 6 7 8 9 10 11 12 Ruslan Gelayev's learning and experience events Actual Predicted likely Predicted worst case Aslan Maskhadov 5 5) • Staffs can profile Red commanders by - 4 finding which past commander may have 3 2 Outcomeindex(1 Decisive conditions Shamil Basayev's most recent experience: Attack and recapture of Grozny in Aug'96. Red Comd Lines of Campaign Campaign DC# Effect… …against… 1 Shamil Opponent's CoG Operation Objective End State SE# Effect… …against… 1 11 2 3 4 5 6 7 8 9 10 11 12Basayev acted in the same way Aslan Maskhadov's learning and experience events Red Comd experiential learning and outcomes Shamil Basayev Algorithm Outcome Actual Predicted likely Predicted worst case performanc interval 5 1 Correlation 5) Likely 85% - 4 Likely vs worst Worst 99% case spread 3 1 Errors Too hi 0 Correct for 2 Too lo 0 Outcomeindex(1 previous Error% 0% 1 1 1 2 3 4 5 6 7 8 9 10 11 12 Shamil Basayev's learning and experience events Source Event Shamil Basayev's learning and experience Effect experienced Response effect (intent) Cultural 1 Kazi Mullah 'nabeg' raid on Kizlyar (taking 200 hostages) in 1831. Defeat Ground manoeuvre Destabilise White Nominative 2 Imam Shamil use of elevated firing positions in forest trees and mountain forts in 1840s. Secure Roads Destroy Ground manoeuvre Combat 3 Defence of Shusha in 'elevated' urban combat vs armour in Nagorno-Karabakh in 1992. Seize Cities Defeat Direct fire Combat 3 Attack on Gagra (in concert with Russia) in Abkhazia in Oct'92. Clear Villages Seize Cities Combat 5 Defence against 42 T72 and 1500 infantry of Chechen opposition in Grozny in Nov'94. Seize Buildings Destroy Ground manoeuvre Combat 6 Defence against 120 T72/T80/BMP/BTRs and 5000 infantry of Russian Group North in Grozny in Dec'94-Jan'95. Seize Buildings Destroy Ground manoeuvre Combat 7 Fighting withdrawal from Grozny to Vedeno in Feb-May'95. Clear Capital Destroy Ground manoeuvre Decisive conditions Shamil Basayev's most recent experience: Attack and recapture of Grozny in Aug'96. Red Comd LinesCombat of 8 Defence of Vedeno in early Jun'95. ManyCampaign members of his immediate family killed in air raids. Had to flee Defeat Ground manoeuvre Destabilise White Campaign DC# Effect… …against… Shamil Opponent's CoG OperationCombat 9 Attack on Budyonnovsk in mid Jun'95. Objective Destroy Ground manoeuvre Divert Fires End State Combat 10SE# AttackEffect… and recapture…against… of Grozny in Aug'96. 11 Defeat Ground manoeuvre BasayevDefeat Ground manoeuvre Situation to test Red's response in Value Weight Value Weight Value Weight Value Weight Terrain Plains 1 Features River 1 Climate Cold 1 Disposition Salient 1 Value Weight Value Weight Value Weight Value Weight Blue #Inf 100000 1 Blue #Armour 100 1 Blue #Arty 500 1 Blue #Aircraft 20 1 Value Weight Value Weight Value Weight Value Weight Red #Inf 20000 1 Red #Armour 10 1 Red #Arty 50 1 Red #Aircraft 2 1 Value Weight Value Weight Value Weight Value Weight Red/Blue Inf 0.2 1 Red/Blue Armour 0.1 1 Red/Blue Arty 0.1 1 Red/Blue Aircraft 0.1 1 Event Closest event from Shamil Basayev's learning and experience Mission Scheme Outcome Effect experienced Response effect (intent) 6 Defence against 120 T72/T80/BMP/BTRs and 5000 infantry of Russian Group North in Grozny in Dec'94-Jan'95.Counter-strike Defend in depth Loss Seize Buildings Destroy Ground manoeuvre Situation experienced Measures of Effectiveness Terrain Dense urban Features Cities Climate Cold Disposition Surrounding En Inf losses 6096.00 Blue #Inf 38000 Blue #Armour 120 Blue #Arty Blue #Aircraft LER Inf 0.22 Red #Inf 5000 Red #Armour 4 Red #Arty Red #Aircraft % Inf 0.27 Red/Blue Inf 0.131578947 Red/Blue Armour 0.033333333 Red/Blue Arty Red/Blue Aircraft RC ex % Inf loss 0.11 COMMERCIAL IN CONFIDENCE Page 3 3 WARDEn | Wargamer’s Automatically Recorded Decision Engine • Designed to capture the key parts of the discussions between players in the wargaming environment before providing automated insights into the drivers of the decisions that have been made. • Uses AI and NLP techniques to extract key information from the narratives – Decision drivers – Whom players have held conversations with, therefore what information their decisions are based on, – Wider context behind the decisions that were made. Extracted decisions and drivers Transcription and NLP analysis COMMERCIAL IN CONFIDENCE Page 4 4 Red AI | DR SO • As part of our Red Mirror ‘counter AI’ project – Countering ‘course of action’ AI proved relatively easy – Countering multiple agents with many course of action options is much harder – Lots of ‘swarming’ algorithms for navigation and flocking – No AI representing hostile swarming e.g. multi-axis swarming or surrounding • Deep Reinforcement Swarming Optimisation – Moderately trained – Heavily trained COMMERCIAL IN CONFIDENCE Page 5.
Details
-
File Typepdf
-
Upload Time-
-
Content LanguagesEnglish
-
Upload UserAnonymous/Not logged-in
-
File Pages5 Page
-
File Size-