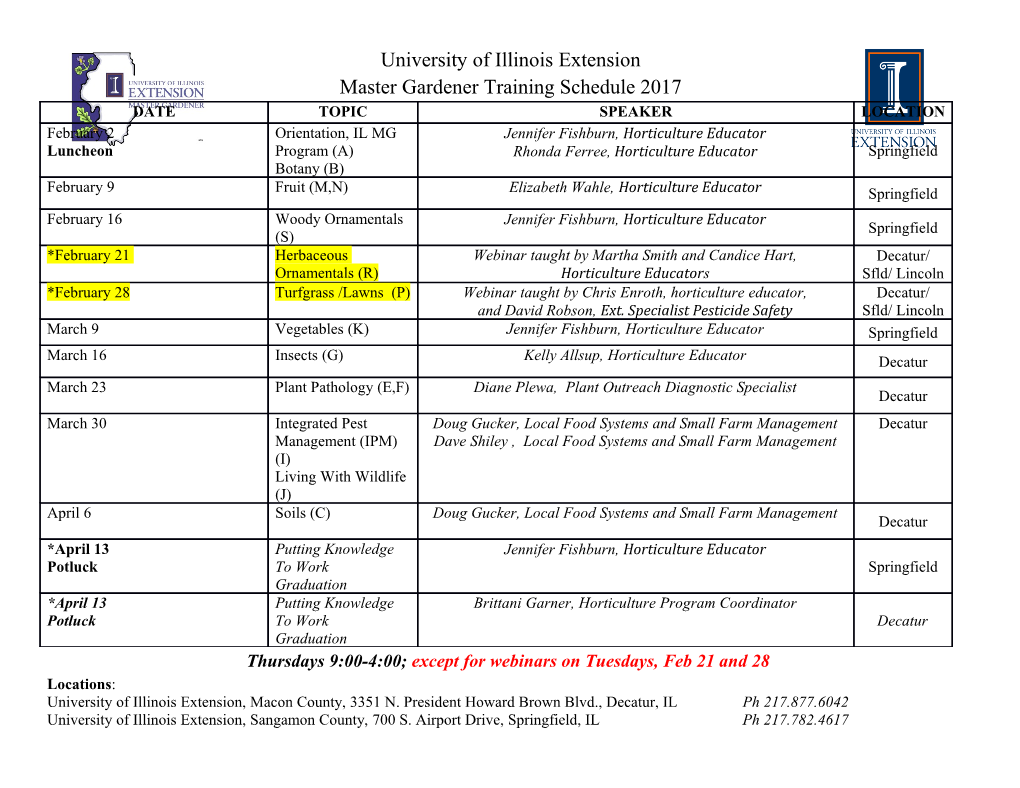
Stat 8112 Lecture Notes The Wilks, Wald, and Rao Tests Charles J. Geyer September 26, 2020 1 Introduction One of the most familiar of results about maximum likelihood is that the likelihood ratio test statistic has an asymptotic chi-square distribution. Those who like eponyms call this the Wilks theorem and the hypothesis test 1 using this test statistic the Wilks test. Let θ^n be the MLE for a model and ∗ θn the MLE for a smooth submodel. The Wilks test statistic is ^ ∗ Tn = 2 ln(θn) − ln(θn) (1) and the Wilks theorem says w Tn −! ChiSq(p − d); where p is the dimension of the parameter space of the model and d is the dimension of the parameter space of the submodel. ^ ∗ It is sometimes easy to calculate one of θn and θn and difficult or impos- sible to calculate the other. This motivates two other procedures that are asymptotically equivalent to the Wilks test. The Rao test statistic is ∗ T ∗ −1 ∗ Rn = rln(θn) Jn(θn) rln(θn); (2) where 2 Jn(θ) = −∇ ln(θ) is the observed Fisher information matrix for sample size n. Under the conditions for the Wilks theorem, the Rao test statistic is asymptotically equivalent to the Wilks test statistic Rn − Tn = op(1): (3) The test using the statistic Rn called the Rao test or the score test or the Lagrange multiplier test. An important point about the Rao test statistic is 1The name is Wilks with an \s" so if one wants a possessive form it would be Wilks's theorem 1 that, unlike the likelihood ratio test statistic, it only depends on the MLE ∗ for the null hypothesis θn. The Wald test statistic is T −1 T −1 Wn = g(θ^n) rg(θ^n)Jn(θ^n) rg(θ^n) g(θ^n); (4) where g is a vector-to-vector constraint function such that the submodel is the set of θ such that g(θ) = 0. Under the conditions for the Wilks theorem, the Wald test statistic is asymptotically equivalent to the Wilks test statistic Wn − Tn = op(1): (5) An important point about the Wald test statistic is that, unlike the like- lihood ratio test statistic, it only depends on the MLE for the alternative hypothesis θ^n. 2 Setup We work under the setup in Geyer (2013). Write −1 qn(δ) = ln(θ0 + τn δ) − ln(θ0); (6) where θ0 is the true unknown parameter value and τn is the rate of conver- gence of the MLE's, that is, we assume τn(θ^n − θ0) = Op(1) ∗ τn(θn − θ0) = Op(1) (our (6) is (14) in Geyer (2013) except that article allows the true unknown parameter to be different for each n). Geyer (2013) says: in \no-n" asymp- totics τn plays no role and we can take τn = 1, so all (6) does is shift the origin to the true unknown parameter value, so the null hypothesis is δ = 0 in the new parameterization. In the \usual asymptotics" of maximum likeli- 1=2 hood (appendix C of that article) we have τn = n , where n is the sample size. In this handout we need τn ! 1 unless the submodel is flat. We assume the random function qn and the maximum likelihood estima- ^ ∗ tors θn and θn satisfy the conditions of Theorem 3.3 in Geyer (2013), which we call the LAMN conditions. These are implied by the \usual regularity conditions" for maximum likelihood Appendix C of that article. 2 3 Model and Submodel 3.1 Explicit Submodel Parameterization There are two ways of specifying a submodel. The first is to specify a one-to-one vector-to-vector function h whose range is the parameter space of the submodel. Then we can write θ = h(') (7) and consider ' the parameter for the submodel, so the log likelihood for the parameter ' and for the submodel is ∗ ln(') = ln(h(')): As always, the reference distribution used for calculating P -values or α- levels assumes the truth is in the null hypothesis (submodel), that is, the true unknown parameter value for θ is θ0 = h('0); (8) where '0 is the true unknown parameter value for '. The corresponding \q" function for the submodel is ∗ ∗ −1 ∗ qn(") = ln('0 + τn ") − ln('0): (9) ∗ ∗ Maximizing qn gives the MLE "n for the parameter ". Then ∗ −1 ∗ 'n = '0 + τn "n is the MLE for the parameter ', and ∗ ∗ θn = h('n) is the MLE in the submodel for the original parameter θ. 3.2 Lagrange Multipliers Alternatively, the submodel can be given (as in our discussion of the Wald test) by a constraint function g, in which case the submodel param- eter space is the set of θ such that g(θ) = 0. The submodel MLE can be determined without explicit parameterization of the submodel using the method of Lagrange multipliers. The function T Lλ(θ) = ln(θ) − λ g(θ) (10) 3 is called the Lagrangian function for the problem and λ is called the vector of Lagrange multipliers for the problem. The method of Lagrange multipliers seeks to maximize (10) while simultaneously satisfying the constraint, that is, we attempt to solve the simultaneous equations T rln(θ) − λ rg(θ) = 0 (11a) g(θ) = 0 (11b) for the unknowns θ and λ. The dimension of the model is p and the dimen- sion of the submodel is d, so the dimension of θ is p and the dimension of λ is p − d, and (11a) and (11b) constitute 2p − d nonlinear equations in 2p − d ∗ ∗ unknowns. If they have a solution, denote the solution (θn; λn). ∗ If θn is a local maximizer of the Lagrangian, that is, if there is a neigh- ∗ borhood W of θn such that ∗ ∗ ∗ ∗ ln(θ) − λng(θ) ≤ ln(θn) − λng(θn); θ 2 W; ∗ then clearly θn is a local maximizer for the constrained problem, that is, ∗ ln(θ) ≤ ln(θn); θ 2 W and g(θ) = 0 (and the same holds with \local" replaced by \global" in both places if p W = R ). Although either works, the method of Section 3.1 is more straightforward than the method of this section. Hence we will hereafter concentrate on that. Since none of the test statistics we deal with depend on the method ∗ by which θn is found (only on its value), all of our results apply to either method (assuming the method of Lagrange multipliers does manage to find the correct root of the equations (11a) and (11b)). Our main reason for introducing the method of Lagrange multipliers (other than just to briefly cover them) is to make the name \Lagrange multiplier test" for the Rao test make sense. Obviously, from (11a) we have ∗ ∗ T ∗ rln(θn) = (λn) rg(θn) so the left side, which appears in the Rao test statistic (2) can be computed using the Lagrange multipliers. The name \score test" comes from the name \score function" given to θ 7! rln(θ) by R. A. Fisher. 4 4 The Wilks Test Theorem 1. Under the assumptions of Theorem 3.3 of Geyer (2013) and the setup of the preceding section, assume the function h defining the sub- model parameterization is twice continuously differentiable and H = rh('0) is a full rank matrix, and define ∗ d q (") = q(H");" 2 R ; where, from equation (16) in Geyer (2013), T 1 T p q(δ) = δ Z + 2 δ Kδ; δ 2 R satisfies the LAMN conditions (Z is a random vector, K is a random matrix, K is almost surely positive definite, and the conditional distribution of Z given K is Normal(0;K)). Define δ^n = τn(θ^n − θ0) (12a) ∗ ∗ "n = τn('n − '0) (12b) ^ ∗ where θn is the MLE for the model and 'n is the MLE for the submodel (both produced by one-step-Newton or infinite-step-Newton updates of τn- consistent estimators). Assume τn ! 1 unless the function h is affine (h(') = a + H', where a is a constant vector), in which case τn can be any sequence. Then 0 1 0 1 qn q ∗ ∗ BqnC w B q C B^ C −! B −1 C @δnA @ K Z A ∗ T −1 T "n (H KH) H Z Proof. The asymptotics of qn and δ^n come directly from Theorem 3.3 in Geyer (2013). By the Skorohod theorem there exist random elementsq ~n 2 p andq ~ of C (R ) such thatq ~n and qn have the same laws andq ~ and q have a.s. 2 p the same laws andq ~n −! q~ in C (R ). Clearlyq ~ can have the form T 1 T p q~(δ) = δ Ze + 2 δ Kδ;e δ 2 R ; where the pair (Z;e Ke) has the same law as the pair (Z; K). 5 The definition (6) can be rewritten ln(θ) = ln(θ0) + qn(τn(θ − θ0)) (13) from which we get ∗ −1 qn(") = ln(h('0 + τn ")) − ln(θ0) −1 = qn(τn(h('0 + τn ") − θ0)) ∗ Hence if we defineq ~n by ∗ −1 q~n(") =q ~n(τn(h('0 + τn ") − θ0)) ∗ ∗ thenq ~n will have the same law as qn, and ∗ −1 T −1 rq~n(") = rq~n(τn(h('0 + τn ") − θ0)) rh('0 + τn ") and 2 ∗ r q~n(") = −1 T 2 −1 −1 rh('0 + τn ") r q~n(τn(h('0 + τn ") − θ0)) rh('0 + τn ") −1 −1 2 −1 + τn rq~n(τn(h('0 + τn ") − θ0))r h('0 + τn "): Suppose "n ! ". If we assume τn ! 1, then −1 τn(h('0 + τn "n) − θ0) ! H": (14) If instead of assuming τn ! 1 we assume h is an affine function, then we have equality for all n in (14) and the limit holds trivially.
Details
-
File Typepdf
-
Upload Time-
-
Content LanguagesEnglish
-
Upload UserAnonymous/Not logged-in
-
File Pages16 Page
-
File Size-