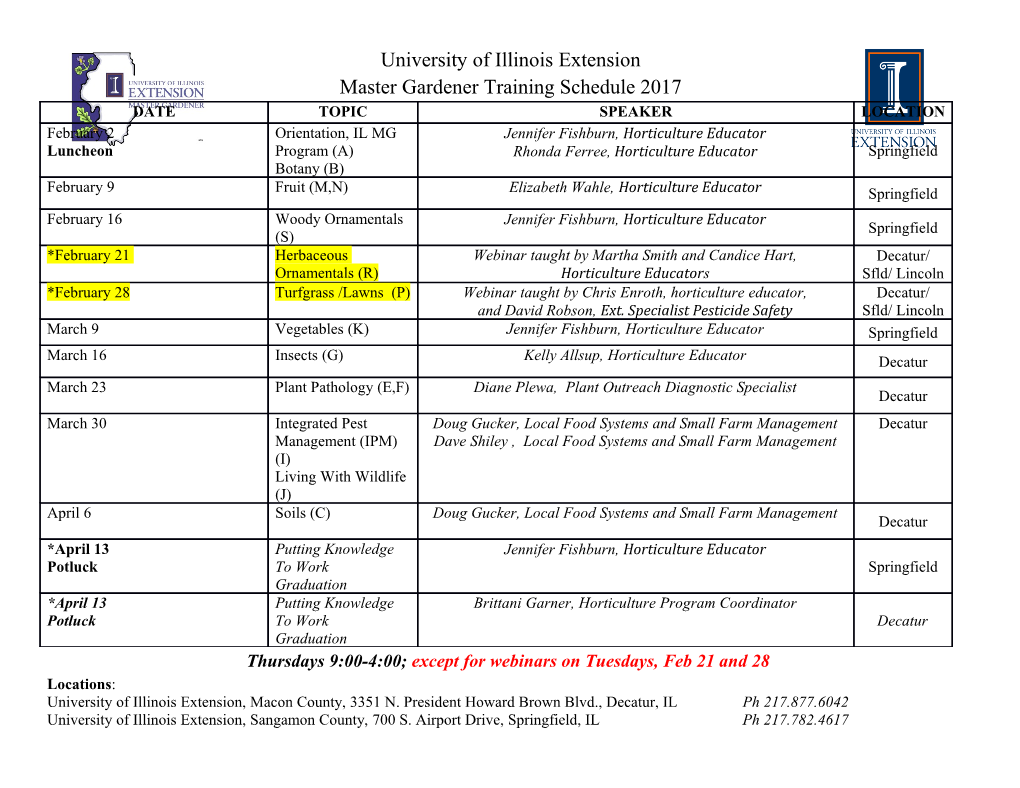
Deep Learning Sensor Fusion for Autonomous Vehicles Perception and Localization: A Review Jamil Fayyad, Mohammad A Jaradat, Dominique Gruyer, Homayoun Najjaran To cite this version: Jamil Fayyad, Mohammad A Jaradat, Dominique Gruyer, Homayoun Najjaran. Deep Learning Sensor Fusion for Autonomous Vehicles Perception and Localization: A Review. Sensors - special issue ”Sen- sor Data Fusion for Autonomous and Connected Driving”, 2020, 20 (15), 35p. 10.3390/s20154220. hal-02942600 HAL Id: hal-02942600 https://hal.archives-ouvertes.fr/hal-02942600 Submitted on 18 Sep 2020 HAL is a multi-disciplinary open access L’archive ouverte pluridisciplinaire HAL, est archive for the deposit and dissemination of sci- destinée au dépôt et à la diffusion de documents entific research documents, whether they are pub- scientifiques de niveau recherche, publiés ou non, lished or not. The documents may come from émanant des établissements d’enseignement et de teaching and research institutions in France or recherche français ou étrangers, des laboratoires abroad, or from public or private research centers. publics ou privés. sensors Review Deep Learning Sensor Fusion for Autonomous Vehicle Perception and Localization: A Review Jamil Fayyad 1, Mohammad A. Jaradat 2,3, Dominique Gruyer 4 and Homayoun Najjaran 1,* 1 School of Engineering, University of British Columbia, Kelowna, BC V1V 1V7, Canada; [email protected] 2 Department of Mechanical Engineering, American University of Sharjah, Sharjah, UAE; [email protected] 3 Department of Mechanical Engineering, Jordan University of Science & Technology, Irbid 22110, Jordan 4 PICS-L, COSYS, University Gustave Eiffel, IFSTTAR, 25 allée des Marronniers, 78000 Versailles, France; dominique.gruyer@univ-eiffel.fr * Correspondence: [email protected] Received: 16 June 2020; Accepted: 24 July 2020; Published: 29 July 2020 Abstract: Autonomous vehicles (AV) are expected to improve, reshape, and revolutionize the future of ground transportation. It is anticipated that ordinary vehicles will one day be replaced with smart vehicles that are able to make decisions and perform driving tasks on their own. In order to achieve this objective, self-driving vehicles are equipped with sensors that are used to sense and perceive both their surroundings and the faraway environment, using further advances in communication technologies, such as 5G. In the meantime, local perception, as with human beings, will continue to be an effective means for controlling the vehicle at short range. In the other hand, extended perception allows for anticipation of distant events and produces smarter behavior to guide the vehicle to its destination while respecting a set of criteria (safety, energy management, traffic optimization, comfort). In spite of the remarkable advancements of sensor technologies in terms of their effectiveness and applicability for AV systems in recent years, sensors can still fail because of noise, ambient conditions, or manufacturing defects, among other factors; hence, it is not advisable to rely on a single sensor for any of the autonomous driving tasks. The practical solution is to incorporate multiple competitive and complementary sensors that work synergistically to overcome their individual shortcomings. This article provides a comprehensive review of the state-of-the-art methods utilized to improve the performance of AV systems in short-range or local vehicle environments. Specifically, it focuses on recent studies that use deep learning sensor fusion algorithms for perception, localization, and mapping. The article concludes by highlighting some of the current trends and possible future research directions. Keywords: autonomous vehicles; self-driving cars; deep learning; sensor fusion; perception; localization and mapping 1. Introduction Autonomous vehicles (AVs) have made impressive technological progress in recent years; these noticeable advancements have brought the concept of self-driving cars into reality. According to a report published by the U.S. Department of Transportation, 94% of vehicle crashes occur due to driver behavior [1]. For this reason, AVs are projected to lower the risk of drastic accidents and increase road safety. Additionally, it is anticipated that AVs will assist in reducing carbon emission levels, and hence protect the environment [2]. Moreover, self-driving cars are expected to smoothen traffic flow, increase productivity, and have enormous economic impacts. Sensors 2020, 20, 4220; doi:10.3390/s20154220 www.mdpi.com/journal/sensors Sensors 2020, 20, 4220 2 of 35 According to the Society of Automobile Engineers (SAE) [3], there are six different levels of automatedSensors 2020 vehicles,, 20, x FOR starting PEER REVIEW from level 0 where the driver is in full control of the vehicle, and2 of ending35 with level 5 where the vehicle is in full control of all driving aspects. These levels are portrayed in Figurewith1 . Currently,level 5 where it canthe vehicle be confidently is in full control stated thatof all levelsdriving 2 andaspects. 3 are These being levels adopted are portrayed in some in of the commercialFigure 1 cars,. Currently, such asit can GM’s be confidently Cruise [4], stated Tesla’s that Autopilot levels 2 and [5], 3 and are being BMW adopted [6]. Several in some autonomous of the featurescommercial are already cars, being such as performed GM’s Cruise in these[4], Tesla’s cars, suchAutopilot as adaptive [5], and BMW cruise [6]. control, Several automatic autonomous braking, features are already being performed in these cars, such as adaptive cruise control, automatic braking, and lane-keeping aid systems. and lane-keeping aid systems. Figure 1. The six levels of autonomous vehicles as described by the Society of Automobile Engineers (SAE) [3], their definitions, and the features in each level. Figure 1. The six levels of autonomous vehicles as described by the Society of Automobile Engineers Although(SAE) [3], diff theirerent definitions, AV systems and the may features differ in slightlyeach level. from one to another, they all need to present a solution forAlthough the autonomous different AV navigation systems may problem, differ slightly which from is generally one to another, divided they into all fourneedmain to present elements: perception,a solution localization for the autonomous and mapping, navigation path planning, problem, and which control. is generally In perception, divided theinto vehicle four main utilizes a groupelements: of onboard perception, sensors tolocalization detect, understand, and mapping, and path interpret planning, the surrounding and control. environment,In perception, including the staticvehicle and dynamic utilizes a obstacles, group of suchonboard as othersensors moving to detect, vehicles, understand, pedestrians, and interpret road signs,the surrounding traffic signals, and roadenvironment, curbs. Localization including static and and mapping dynamic tasks obstacles, attempt such to locateas other the moving vehicle vehicles, globally pedestrians, with respect to worldroad coordinates. signs, traffic Additionally, signals, and they road are curbs. responsible Localization forbuilding and mapping a map tasks of the attempt vehicle’s to locate surroundings the and continuouslyvehicle globally tracking with respect the vehicle’s to world location coordinates. with Additionally, respect to that they map. are responsible Subsequently, for building path planning a exploitsmap the of outputthe vehicle’s of the surroundings previous two and tasks continuously in order to adopttracking the the optimal vehicle’s and location safestfeasible with respect route to for the that map. Subsequently, path planning exploits the output of the previous two tasks in order to adopt AV to reach its destination, while considering all other possible obstacles on the road [7]. Lastly, based on the optimal and safest feasible route for the AV to reach its destination, while considering all other the selectedpossible path, obstacles the controlon the road element [7]. Lastly, outputs based the on necessary the selected values path, of the acceleration, control element torque, outputs and the steering anglenecessary for the vehicle values of to acceleration, follow that torque, selected and path steering [8]. angle Additionally, for the vehicle multiple to follow studies that selected consider path adding connected[8]. Additionally, vehicle technologies multiple studies [9,10 ],consider such as adding vehicle-to-vehicle connected vehicle (V2V) technologies and vehicle-to-infrastructure [9,10], such as (V2I)vehicle-to-vehicle technologies, where (V2V) essential and informationvehicle-to-infrastructure is shared to(V2I) create technologies, an enhanced where cooperative essential driving environment,information as is shown shared to in create Figure an2 .enhanced This extended cooperative and driving improved environment, cooperative as shown perception in Figure allows 2. vehiclesThis to extended predict and the behaviorimproved ofcooperative the key environmental perception allows components vehicles to (obstacles,predict the roads,behavior ego-vehicles, of the environment,key environmental driver behavior) components efficiently (obstacles, and toroads, anticipate ego-vehicles, any possible environment, hazardous driver events. behavior) efficiently and to anticipate any possible hazardous events. Sensors 2020, 20, 4220 3 of 35 Sensors 2020, 20, x FOR PEER REVIEW 3 of 35 FigureFigure 2. Full 2. Full autonomous autonomous navigation navigationsystem. system. Sensor
Details
-
File Typepdf
-
Upload Time-
-
Content LanguagesEnglish
-
Upload UserAnonymous/Not logged-in
-
File Pages36 Page
-
File Size-