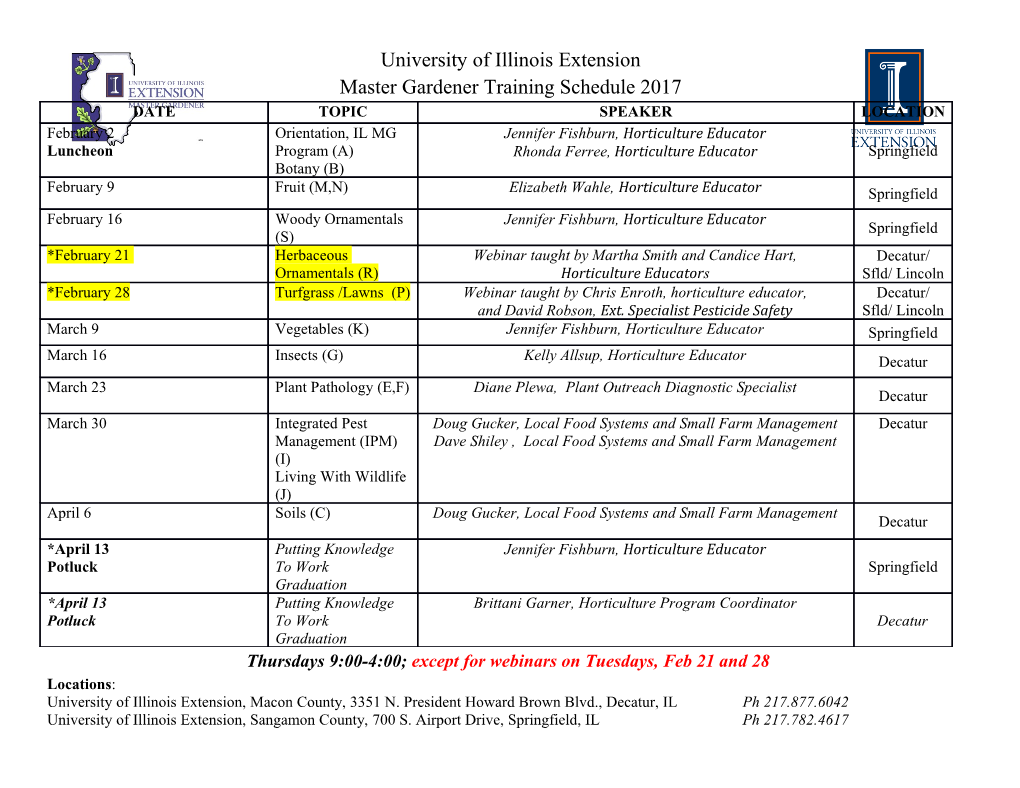
Statistics 149 { Spring 2016 { Assignment 4 Solutions Due Monday April 4, 2016 1. For the Poisson distribution, b(θ) = eθ and thus µ = b′(θ) = eθ. Consequently, θ = ∗ g(µ) = log(µ) and b(θ) = µ. Also, for the saturated model µi = yi. n ∗ ∗ D(µSy) = 2 Q yi(θi − θi) − b(θi ) + b(θi) i=1 n ∗ ∗ = 2 Q yi(log(µi ) − log(µi)) − µi + µi i=1 n yi = 2 Q yi log − (yi − µi) i=1 µi 2. (a) After following instructions for replacing 0 values with NAs, we summarize the data: > summary(mypima2) pregnant glucose diastolic triceps insulin Min. : 0.000 Min. : 44.0 Min. : 24.00 Min. : 7.00 Min. : 14.00 1st Qu.: 1.000 1st Qu.: 99.0 1st Qu.: 64.00 1st Qu.:22.00 1st Qu.: 76.25 Median : 3.000 Median :117.0 Median : 72.00 Median :29.00 Median :125.00 Mean : 3.845 Mean :121.7 Mean : 72.41 Mean :29.15 Mean :155.55 3rd Qu.: 6.000 3rd Qu.:141.0 3rd Qu.: 80.00 3rd Qu.:36.00 3rd Qu.:190.00 Max. :17.000 Max. :199.0 Max. :122.00 Max. :99.00 Max. :846.00 NA's :5 NA's :35 NA's :227 NA's :374 bmi diabetes age test Min. :18.20 Min. :0.0780 Min. :21.00 Min. :0.000 1st Qu.:27.50 1st Qu.:0.2437 1st Qu.:24.00 1st Qu.:0.000 Median :32.30 Median :0.3725 Median :29.00 Median :0.000 Mean :32.46 Mean :0.4719 Mean :33.24 Mean :0.349 3rd Qu.:36.60 3rd Qu.:0.6262 3rd Qu.:41.00 3rd Qu.:1.000 Max. :67.10 Max. :2.4200 Max. :81.00 Max. :1.000 NA's :11 We can see that the 0s have successively been converted to NAs. We still see that test has a mean of 0.349, indicating that 34.9% received the test, and so on, similar to the previous homework. (b) First we use the na.convert.mean() function. Then we again summarize the data: > summary(mypima2.na) pregnant glucose diastolic triceps insulin Min. : 0.000 Min. : 44.00 Min. : 24.00 Min. : 7.00 Min. : 14.0 1st Qu.: 1.000 1st Qu.: 99.75 1st Qu.: 64.00 1st Qu.:25.00 1st Qu.:121.5 1 Median : 3.000 Median :117.00 Median : 72.20 Median :29.15 Median :155.5 Mean : 3.845 Mean :121.69 Mean : 72.41 Mean :29.15 Mean :155.5 3rd Qu.: 6.000 3rd Qu.:140.25 3rd Qu.: 80.00 3rd Qu.:32.00 3rd Qu.:155.5 Max. :17.000 Max. :199.00 Max. :122.00 Max. :99.00 Max. :846.0 bmi diabetes age test glucose.na Min. :18.20 Min. :0.0780 Min. :21.00 Min. :0.000 Min. :0.00000 1st Qu.:27.50 1st Qu.:0.2437 1st Qu.:24.00 1st Qu.:0.000 1st Qu.:0.00000 Median :32.40 Median :0.3725 Median :29.00 Median :0.000 Median :0.00000 Mean :32.46 Mean :0.4719 Mean :33.24 Mean :0.349 Mean :0.00651 3rd Qu.:36.60 3rd Qu.:0.6262 3rd Qu.:41.00 3rd Qu.:1.000 3rd Qu.:0.00000 Max. :67.10 Max. :2.4200 Max. :81.00 Max. :1.000 Max. :1.00000 diastolic.na triceps.na insulin.na bmi.na Min. :0.00000 Min. :0.0000 Min. :0.000 Min. :0.00000 1st Qu.:0.00000 1st Qu.:0.0000 1st Qu.:0.000 1st Qu.:0.00000 Median :0.00000 Median :0.0000 Median :0.000 Median :0.00000 Mean :0.04557 Mean :0.2956 Mean :0.487 Mean :0.01432 3rd Qu.:0.00000 3rd Qu.:1.0000 3rd Qu.:1.000 3rd Qu.:0.00000 Max. :1.00000 Max. :1.0000 Max. :1.000 Max. :1.00000 The fraction of missing values for glucose, diastolic, triceps, insulin, and bmi was 0.007, 0.046, 0.296, 0.487, and 0.014, respectively. The min, max, and quantiles are exactly the same for the observed diastolic and bmi values. The mean is the same for the observed glucose, tricepts and insulin values, but the spread is slightly smaller. When using the na.convert.mean() function, we always expect (1) the mean, min, and max to be the same, and (2) the spread to be smaller, because we imputed the mean of the observed values. (c) > glm.pima2.na = glm(test ~ ., data = mypima2.na, family = binomial) > summary(glm.pima2.na) Call: glm(formula = test ~ ., family = binomial, data = mypima2.na) Deviance Residuals: Min 1Q Median 3Q Max -2.7247 -0.7140 -0.3893 0.7147 2.4596 Coefficients: Estimate Std. Error z value Pr(>|z|) (Intercept) -9.3826661 0.8313109 -11.287 < 2e-16 *** pregnant 0.1244084 0.0325203 3.826 0.00013 *** glucose 0.0378306 0.0039461 9.587 < 2e-16 *** diastolic -0.0104368 0.0087623 -1.191 0.23361 triceps 0.0040094 0.0134387 0.298 0.76544 insulin -0.0006452 0.0011822 -0.546 0.58526 2 bmi 0.0959924 0.0180916 5.306 1.12e-07 *** diabetes 0.9765315 0.3059045 3.192 0.00141 ** age 0.0121485 0.0096162 1.263 0.20647 glucose.na 0.4478017 1.0720840 0.418 0.67617 diastolic.na 1.0150992 0.4814151 2.109 0.03498 * triceps.na -0.0368346 0.2905943 -0.127 0.89913 insulin.na 0.3372729 0.2683721 1.257 0.20885 bmi.na -0.9070556 0.8720426 -1.040 0.29827 --- Signif. codes: 0 `***' 0.001 `**' 0.01 `*' 0.05 `.' 0.1 ` ' 1 (Dispersion parameter for binomial family taken to be 1) Null deviance: 993.48 on 767 degrees of freedom Residual deviance: 704.02 on 754 degrees of freedom AIC: 732.02 Number of Fisher Scoring iterations: 5 The only significant missing-value indicator is that for diastolic.na. This pro- vides evidence of a significant difference in mean response between units that are missing diastolic and those that are not. In particular, those missing diastolic are estimated to be about one unit higher on the log-odds scale, adjusting for all other variables in the model. Furthermore, this also indicates that there is a lack of evidence of a significant difference in the mean response between units that are missing other values and those that are not. Comparing the other coefficients for this model to that in Homework 3, pregnant is now significant, diastolic is more significant (but still insignificant at the 0.05 level), and age is less significant (and still insignificant at the 0.05 level). Because pregnant is now significant and has increased in magnitude, there is likely a positive relationship between pregnant and receiving the test among units with missing values. Recall that we made an ignorability assumption when we used the na.convert.mean() function (i.e., the missingness only depends on observed values, and not the missing values themselves). This assumption is especially important if one wants to make inference about the relationship between pregnant and test. We cannot use the data to test this assumption, so one should think carefully about this assumption before making conclusions from the above model. 3. (a) We fit the log-linear model that assumes independence of the four factors. 3 > summary(glm(Count ~ M + G + P + E, data = div, family = poisson)) Estimate Std. Error z value Pr(>|z|) (Intercept) 4.75654 0.06525 72.901 <2e-16 *** MMarried 0.09273 0.06220 1.491 0.136 GWomen 0.63009 0.06525 9.657 <2e-16 *** PYes -1.19355 0.07353 -16.231 <2e-16 *** EYes -2.02313 0.09673 -20.915 <2e-16 *** Null deviance: 1333.85 on 15 degrees of freedom Residual deviance: 232.14 on 11 degrees of freedom > anova(mod.no.E, mod.no.P, mod.no.G, mod.no.M, mod.full, test = "Chi") Analysis of Deviance Table Model 1: Count ~ M + G + P Model 2: Count ~ M + G + E Model 3: Count ~ M + P + E Model 4: Count ~ G + P + E Model 5: Count ~ M + G + P + E Resid. Df Resid. Dev Df Deviance Pr(>Chi) 1 12 921.40 2 12 544.43 0 376.98 3 12 330.08 0 214.35 4 12 234.36 0 95.71 5 11 232.14 1 2.22 0.1358 There is some indication that the model does not fit well as the residual deviance over residual degree of freedom is far above 1. Both the p-values and the drop in deviance tests confirm that M is not a significant predictor, while G, P and E are significant predictors. (b) Since we have three significant predictors, G, P and E, we consider models with 2-way and 3-way interactions. Noting the deviances in Figure 1, the best model appears to be the model with the three predictor variables and the 2-way inter- actions G:P and E:P. This corresponds to the factors being pairwise dependent. For instance, GWomen:PYes = -1.25341; this says that women are less likely to have engaged in pre-marital sex than men. 4 Model Deviance df Count ∼ G + P + E 234.36 12 Count ∼ G + P + E + G:P 159.11 11 Count ∼ G + P + E + G:E 221.6 11 Count ∼ G + E + P + E:P 188.35 11 Count ∼ G + E + P + G:P + E:P 113.09 11 Count ∼ G + E + P + G:P + G:E + E:P 110.18 9 Count ∼ G*E*P 110.18 8 Figure 1: Deviances for divorce models (c) Figure 2 show the diagnostic plots.
Details
-
File Typepdf
-
Upload Time-
-
Content LanguagesEnglish
-
Upload UserAnonymous/Not logged-in
-
File Pages10 Page
-
File Size-