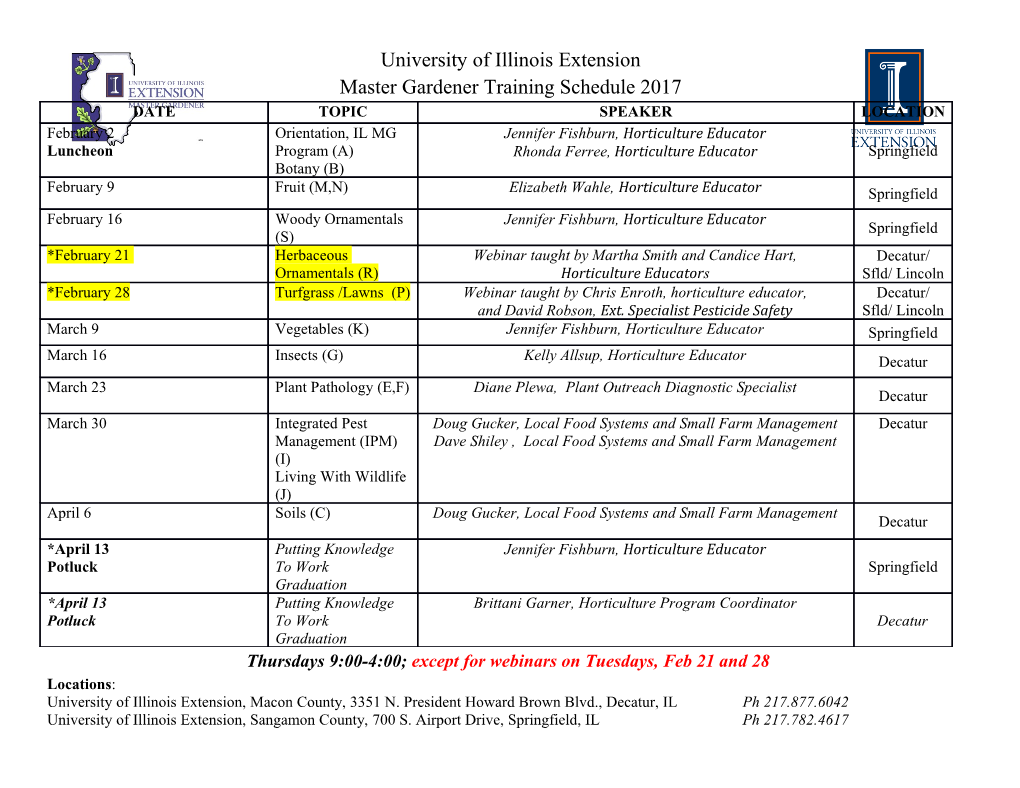
A Probabilistic Constraint-based Approach to Protein Structure Predication 1 Backgrounds and Objectives Protein folding and protein structure predication Protein folding is the process by which a protein structure assumes its functional shape or conforma- tion. It is believed that the dynamical folding of a protein to its native conformation is determined by the amino acid sequence of the protein. Given the usefulness of known protein structures in such valuable tasks as rational drug design, protein structure prediction is a highly active field of research in bioinformatics [3, 10]. During recent decades various methods have been developed along the following two main lines [3]. The first line of methods, called homology modeling, rely on assembling structures of proteins using structural fragments of similar sequences available in sources such as PDB [5]. The second line of methods, called ab initio methods, predict the structure from sequence alone, without relying on similarity at the fold level between the modeled sequence and any of the known structures. The folding of any particular protein is an extremely complex process; and simulation of the folding of even a small protein remains an insurmountable challenge to state-of-the-art computers. Many ab initio methods adopt reduced models [18] such as lattice models which alleviate the com- plexity of the process because of the reduced number of degrees of freedom. Reduced models cannot be expected to consistently generate predications with high accuracy. Nevertheless, the low resolu- tion results obtained can be used to narrow the possible conformations from an exponentially large number to a number small enough that more computationally expensive methods can be applied. The constraint-based approach to protein structure predication Constraint programming [9, 32] is a declarative programming paradigm well suited for describing and solving combinatorial optimization problems. Constraint languages allow for not only high-level modeling but also efficient solving of problems. Recent implementations are amenable to large scale problems thanks to the availability of constraint solving algorithms such as propagation algorithms for finite-domain constraints [22], sophisticated heuristics [14], and advanced compilation techniques [8, 36]. Constraint programming has found its way into many application areas such as design, scheduling, configuration, bioinformatics, and graphical user interface design [6, 12, 15, 13, 33, 35]. Several constraint programming systems are available now. The PI, Neng-Fa Zhou, has designed and implemented an event-handling language, called AR (Action Rules), and developed a state-of- the-art and award-winning1 constraint solver in AR available with B-Prolog [36]. Several attempts have been made to apply constraint programming to protein structure predi- cation [2, 21, 25]. All the systems adopt lattice models for proteins and use some simplified energy functions in the optimization. There are different ways to map lattice models for proteins into CSP (Constraint Satisfaction Problem) models. A straightforward way is to treat amino acids or residues 1The solver was ranked top in two categories in the Second International Solvers Competition (http://www.cril.univ-artois.fr/CPAI06/). 2 as variables and lattice positions as the domains of variables. The only enforced constraints in this model are neighborhood constraints which ensure that every pair of residues are placed in neigh- boring coordinates. Auxiliary constraints, such as constraints for breaking symmetries, are used to enhance the performance. The constraint-based approach has produced some exciting results. For example, Backofen and Will report in [2] that their program outperforms all other approaches for lattice HP models and is able to find optimal structures and prove the optimality for proteins of up to 200 residues. In [26] a goal is set to handle proteins of lengths up to 500. Logic-based probabilistic learning The past few years have witnessed a tremendous interest in logic-based probabilistic learning as testified by an increasing number of formalisms and systems (e.g., BLP [17], CLP(BN) [11], PRM [20], PRISM [29], and SLP [24]). Logic-based probabilistic learning is a multidisciplinary research area that integrates relational or logic formalisms, probabilistic reasoning mechanisms, and machine learning and data mining principles. Logic-based probabilistic learning has found its way into many application areas including bioinformatics, diagnosis and troubleshooting, stochastic language processing, information retrieval, linkage analysis and discovery, and robot control. The PI has involved in the development of PRISM,2 an extension of B-Prolog that integrates logic programming, probabilistic reasoning, and EM learning [29, 30, 38]. PRISM allows for the description of independent probabilistic choices and their consequences in general logic programs. PRISM supports parameter learning. For a given set of possibly incomplete observed data, PRISM can estimate the probability distributions to best explain the data. PRISM suitable for applications such as learning parameters of stochastic grammars, training stochastic models for gene sequence analysis, user modeling, and obtaining probabilistic information for tuning systems performance. PRISM offers incomparable flexibility compared with specific statistical tools such as Hidden Markov Models (HMMs) [28], Probabilistic Context Free Grammars (PCFGs) [34] and discrete Bayesian networks [7, 27]. Thanks to an efficient tabling system [39] and other optimization techniques, the latest version of PRISM is able to handle large volumes of data. For example, a natural language application written in PRISM is able to train probabilistic models using corpora of tens of thousands of sentences. There is an increasing interest in using machine learning techniques to learn heuristics for search. For CSPs, the heuristics used to order variables and values can have a dramatic effect on the per- formance. Various kinds of strategies have been proposed (e.g., the first-fail principle for ordering variables [22] and the mini-conflict strategy for ordering values [16]). Nevertheless, for many prob- lems users still have to experiment with different heuristics and tune them manually, and this process can be very tedious and painful. Objectives We propose a probabilistic constraint-based approach to protein structure predication. Our approach is based on the reasonable assumption that two similar proteins will have similar structures. In this project we will develop probabilistic models and train them using proteins in the PBD with known structures. These probabilistic models will be used to predicate structures for other proteins. The number of proteins with known three-dimensional conformations in the PDB is the order of 16,000 and is increasing rapidly, while the number of conformation classes has remained about 500 for some 2Available from http://mi.cs.titech.ac.jp/prism/. 3 time is not expected to grow beyond 1000.3 It is expected that a trained probabilistic model can guide the search, leading to a better low bound and thus a dramatic reduction of the search space. This project also aims to improve the constraint-based methods used in existing systems. In concrete, we propose the following: (1) tailor our solver to the protein structure predication problem; (2) design and implement new global constraints and propagation algorithms for the problem; and (3) investigate the effectiveness of other constraint solving techniques such as symmetry-breaking and dual-modeling techniques to this problem. Merits and impacts The main contribution of the project will be a high-performance specialized solver for protein struc- ture predication that takes advantage of probability distributions learned from existing proteins with known structures. The system will be based on our efficient constraint logic programming system B-Prolog and the probabilistic learning system PRISM, and will incorporate the following research results: • Probabilistic models: A naive probabilistic model would facilitate learning but could hardly produce useful results to guide search. On the other hand, a sophisticated model entails expo- nential learning time. An optimal probabilistic model will be developed through experiments that well balances the learning complexity and the quality of results. • Special-purpose constraints: Special-purpose constraints including routing, symmetry-breaking, and dual-modeling constraints will be identified for the problem, and will be implemented using the AR language available in B-Prolog. This is a multidisciplinary research project which will involve both computer scientists and biologists. The research results will disseminated through several avenues and the resulting system from the project will be made available to the public. 2 Protein Structure Predication as a Constraint Satisfaction and Optimization Problem Proteins are amino acid chains, made up from 20 different amino acids, also referred to as residues, that fold into unique three-dimensional protein structures. Let R = r1,...,rn be a sequence of residues. A structure of R is represented by a sequence of points in a three-dimensional space p1,...,pn where pi =<xi,yi,zi>. The following generic formula defines the energy of a structure: E =ΣiΣj>iErirj ∆pipj where Erirj specifies the contact energy of residues ri and rj , and ∆pipj is a value dependent on the distance between pi and pj in the space. A structure is a self-avoiding
Details
-
File Typepdf
-
Upload Time-
-
Content LanguagesEnglish
-
Upload UserAnonymous/Not logged-in
-
File Pages8 Page
-
File Size-