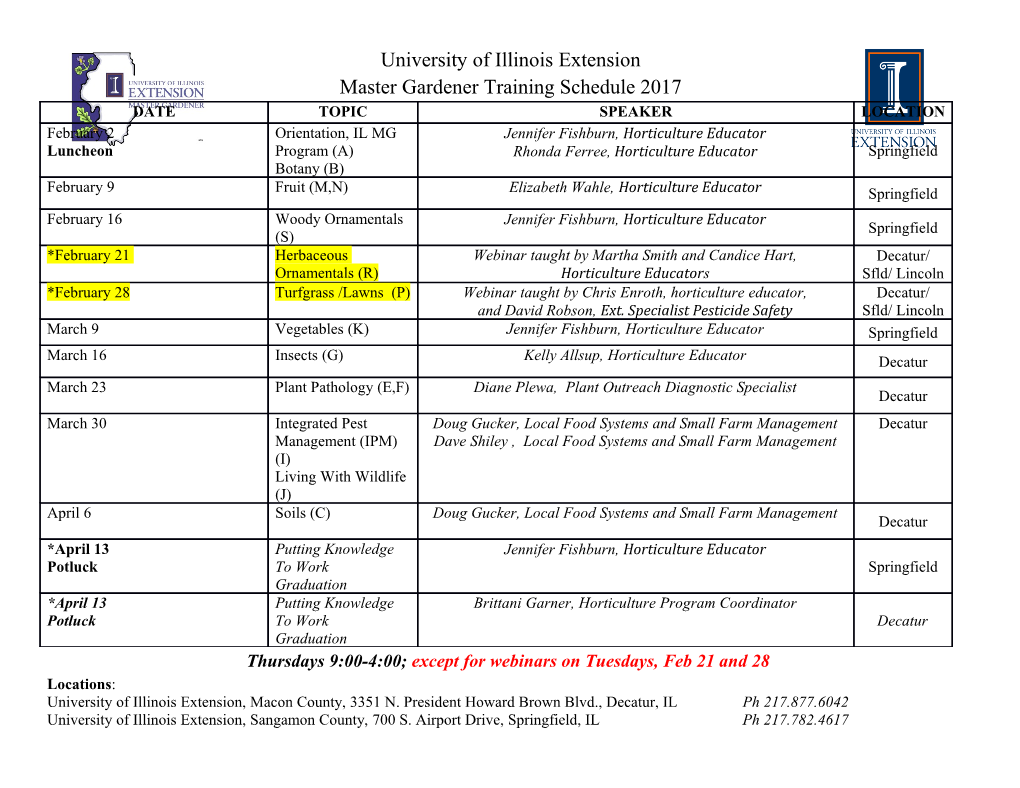
Optimization Basic Results Michel De Lara Cermics, Ecole´ des Ponts ParisTech France Ecole´ des Ponts ParisTech November 22, 2020 Outline of the presentation Magic formulas Convex functions, coercivity Existence and uniqueness of a minimum First-order optimality conditions (the case of equality constraints) Duality gap and saddle-points Elements of Lagrangian duality and Uzawa algorithm More on convexity and duality Outline of the presentation Magic formulas Convex functions, coercivity Existence and uniqueness of a minimum First-order optimality conditions (the case of equality constraints) Duality gap and saddle-points Elements of Lagrangian duality and Uzawa algorithm More on convexity and duality inf h(a; b) = inf inf h(a; b) a2A;b2B a2A b2B inf λf (a) = λ inf f (a) ; 8λ ≥ 0 a2A a2A inf f (a) + g(b) = inf f (a) + inf g(b) a2A;b2B a2A b2B Tower formula For any function h : A × B ! [−∞; +1] we have inf h(a; b) = inf inf h(a; b) a2A;b2B a2A b2B and if B(a) ⊂ B, 8a 2 A, we have inf h(a; b) = inf inf h(a; b) a2A;b2B(a) a2A b2B(a) Independence For any functions f : A !] − 1; +1] ; g : B !] − 1; +1] we have inf f (a) + g(b) = inf f (a) + inf g(b) a2A;b2B a2A b2B and for any finite set S, any functions fs : As !] − 1; +1] and any nonnegative scalars πs ≥ 0, for s 2 S, we have X X inf π f (a )= π inf f (a ) Q s s s s s s fas gs2 2 s2 As as 2As S S s2S s2S Outline of the presentation Magic formulas Convex functions, coercivity Existence and uniqueness of a minimum First-order optimality conditions (the case of equality constraints) Duality gap and saddle-points Elements of Lagrangian duality and Uzawa algorithm More on convexity and duality Convex sets Let N 2 N∗. We consider subsets of the Euclidian space RN N I The subset C ⊂ R is convex if for any x1 2 C, x2 2 C and t 2 [0; 1], we have that tx1 + (1 − t)x2 2 C I An intersection of convex sets is convex I A segment is convex I A hyperplane is convex( H ⊂ RN is a hyperplane if there exists N N y 2 R nf0g and b 2 R such that H = x 2 R hx ; yi + b = 0 ) I An affine subspace (intersection of hyperplanes) is convex Linear and affine functions Consider a function f : RN ! R N N I The function f is linear if, for any x1 2 R , x2 2 R and t1 2 R, t2 2 R, f (t1x1 + t2x2) = t1f (x1) + t2f (x2) N N I The function f is affine if, for any x1 2 R , x2 2 R and t 2 R, f (tx1 + (1 − t)x2) = tf (x1) + (1 − t)f (x2) Exercise. Show that f : RN ! R is affine if and only if g(x) = f (x) − f (0) is linear Convex functions (definitions) Let C ⊂ RN be an nonempty convex subset of RN , where N 2 N∗, and f : C ! R be a function I The function f is affine if, for any x1 2 C, x2 2 C and t 2 [0; 1], f (tx1 + (1 − t)x2) = tf (x1) + (1 − t)f (x2) I The function f is convex if, for any x1 2 C, x2 2 C and t 2 [0; 1], f (tx1 + (1 − t)x2) ≤ tf (x1) + (1 − t)f (x2) I The function f is strictly convex if, for any x1 2 C, x2 2 C, x1 6= x2, and t 2]0; 1[, f (tx1 + (1 − t)x2) < tf (x1) + (1 − t)f (x2) I The function f is strongly convex (of modulus a > 0) if, for any x1 2 C, x2 2 C and t 2 [0; 1], a f (tx + (1 − t)x ) ≤ tf (x ) + (1 − t)f (x ) − t(1 − t)kx − x k2 1 2 1 2 2 1 2 Exercises Let C ⊂ RN be an nonempty subset of RN , where N 2 N∗ I Show that both definitions of an affine function coincide when C = RN I Show that a function f : C ! R is convex if and only if its epigraph is a convex set subset of RN × R I Show that a function f : C ! R is strongly convex of modulus a > 0 a 2 if and only if g(x) = f (x) − 2 kxk is convex I If f : C ! R is convex, show that f is not strictly convex if and only there exists a nonempty convex subset C 0 ⊂ C over which f is affine Convex functions on the real line Proposition Let I ⊂ R be an nonempty interval I A C 1 function f : I ! R is convex if and only if f 0 is increasing on I I A C 2 function f : I ! R is convex if and only if f 00(x) ≥ 0, for all x 2 I I Let a > 0.A C 2 function f : I ! R is a-strongly convex if and only if f 00(x) ≥ a, for all x 2 I I A C 1 function f : I ! R is strictly convex if and only if f is convex and the set fx 2 I j f 00(x) = 0g is either empty or is a singleton Exercise. Study the family of functions fα :]0; +1[! R given by α fα(x) = x . For which values of the parameter α is the function fα convex? For a given a > 0, for which values of the parameter α is the function fα strongly convex of modulus a? Provide an example of a strictly convex function which is not strongly convex. Convexity for multivariate functions 2 N The Hessian matrix Hf (x) of a C function f : R ! R is the N × N symmetric matrix given by @2f Hf (x) = i j (x) @x @x (i;j)2f1;:::;Ng2 Proposition Let C ⊂ RN be an nonempty convex subset of RN , where N 2 N∗ I A C 2 function f : RN ! R is convex on C if and only if the symmetric Hessian matrix Hf (x) is positive for all x 2 C I A C 2 function f : RN ! R is strongly convex of modulus a > 0 on C if and only if the eigenvalues of the symmetric Hessian matrix Hf (x) are uniformly bounded below by a > 0 on C Exercise. Let Q be a N × N symmetric matrix and f (x) = 1=2x 0Qx, where x 0 is the transpose of the vector x. Give conditions on the smallest eigenvalue of Q so that the function f is convex, or strictly convex, or strongly convex of modulus a. Operations on functions preserving convexity Proposition Let (fi )i2I be a family of convex functions Then supi2I fi is a convex function Proposition Let (fi )i=1;:::;n be convex functions Let (αi )i=1;:::;n be nonnegative numbers Pm Then i=1 αi fi is a convex function Proposition Let f : RN ! R be convex Let A be a N × M matrix and b 2 RN Then y 2 RM 7! f (Ay + b) is a convex function Coercivity Definition A function f : RN ! R is coercive if lim f (x) = +1 kxk!+1 Proposition A strongly convex function is coercive Outline of the presentation Magic formulas Convex functions, coercivity Existence and uniqueness of a minimum First-order optimality conditions (the case of equality constraints) Duality gap and saddle-points Elements of Lagrangian duality and Uzawa algorithm More on convexity and duality Here are the ingredients for a general abstract optimization problem inf J(u) ad u2U I Optimization set U(= RN ) containing optimization variables u 2 U I A criterion J : U ! R [ f+1g I Constraints of the form u 2 Uad ⊂ U Examples of classes of optimization problems inf J(u) ad u2U I Linear programming N I Optimization set U = R I Criterion J is linear (affine) ad I Constraints U defined by a finite number of linear (affine) equalities and inequalities I Convex optimization I Criterion J is a convex function ad I Constraints U define a convex set I Combinatorial optimization N N I Optimization set U is discrete (binary f0; 1g , integer Z , etc.) Minimum Definition We say that u∗ 2 U is a (global) minimum of the optimization problem inf ad J(u) if u2U ∗ ad ∗ ad u 2 U and J(u ) ≤ J(u) ; 8u 2 U In this case, we write J(u∗) = min J(u) ad u2U Existence and uniqueness of a minimum We consider the optimization problem inf J(u) where ad ⊂ = N ad U U R u2U Proposition If the criterion J is continuous and the constraint set Uad is compact (bounded and closed), then there is a minimum Proposition If the constraint set Uad is closed and the criterion J is continuous and coercive, then there is a minimum Proposition If the constraint set Uad is convex, and if the criterion J is strictly convex, a minimum is necessarily unique Exercises We consider the optimization problem inf J(u) ad u2U Give an example I of continuous criterion J and of constraint set Uad for which there is no minimum I of criterion J and of compact constraint set Uad for which there is no minimum I of continuous criterion J and of unbounded and closed constraint set Uad for which there is no minimum I of convex criterion J and of constraint set Uad for which there is more than one minimum I of strictly convex criterion J and of constraint set Uad for which there is more than one minimum Local minimum Definition We say that u∗ 2 U is a local minimum of the optimization problem ∗ ad inf ad J(u) if there exists a neighborhood V of u in such that u2U U ∗ ad ∗ u 2 U and J(u ) ≤ J(u) ; 8u 2 V Proposition If the constraint set Uad is convex, and if the criterion J is convex, a local minimum is a global minimum Outline of the presentation Magic formulas Convex functions, coercivity Existence and uniqueness of a minimum First-order optimality conditions (the case of equality constraints) Duality gap and saddle-points Elements of Lagrangian duality and Uzawa algorithm More on convexity and duality Optimization under equality constraints We consider the optimization problem inf J(u) N u2R under the constraint Θ(u) = 0 where Θ is a function with values in RM N M Θ = (Θ1;:::; ΘM ): R ! R whose components are denoted by Θj , where j runs from 1 to M Sufficient condition for qualification in case of equality constraints Definition Let u∗ 2 RN .
Details
-
File Typepdf
-
Upload Time-
-
Content LanguagesEnglish
-
Upload UserAnonymous/Not logged-in
-
File Pages57 Page
-
File Size-