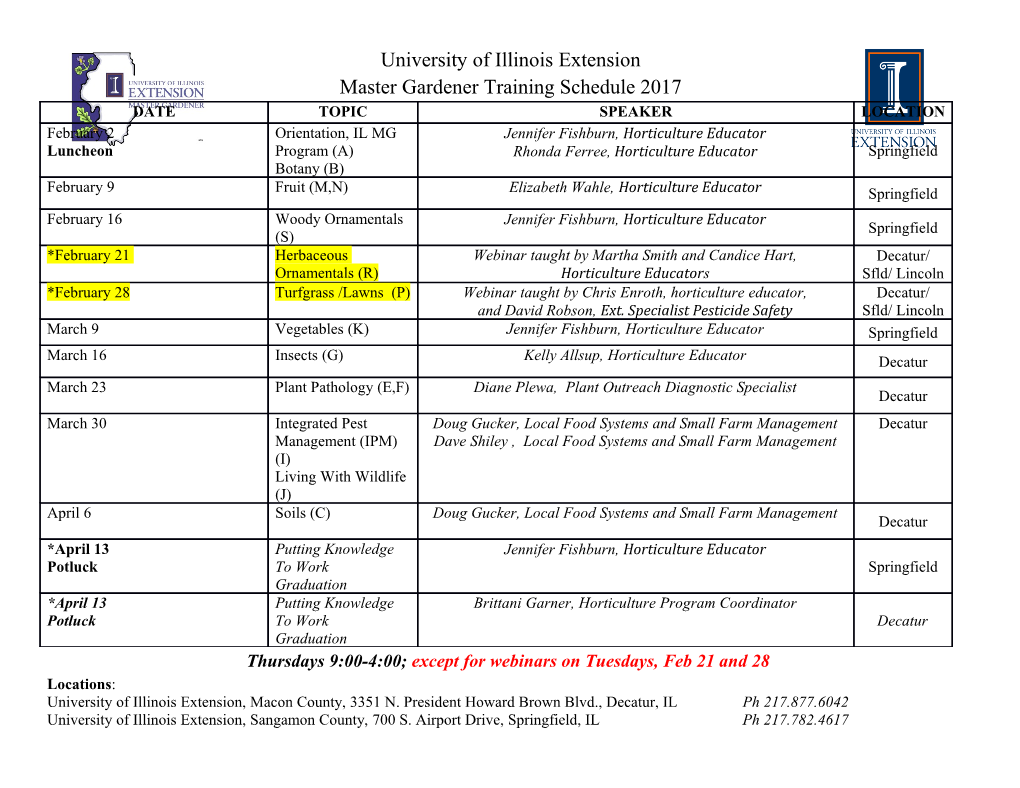
Do relationships change after a crisis?The case of a housing market♦ Charles Ka Yui Leung and Patrick Wai Yin Cheung♣ This version: June 2007 Abstract This paper studies the co-movements of different real estate developments following the approach of Forbes and Rigobon (2002). The mean correlation of price, trading volume as well, among estates increases before a financial crisis. The distribution of the correlations is also skewed. After the crisis, the correlations drop dramatically and stay low. We also adopt the approach by Campbell et al. (2001) to decompose the price variance. The share of the idiosyncratic component increases from about 60% to 90% after the crisis. These findings are consistent to a family of theories which emphasize on “regime switch” in expectation. JEL Classification Number: G10, R30 Keywords: financial crisis, hedonic pricing, structural break, evolution of valuation, rolling regression ♦ Acknowledgement: The authors are very grateful to Nan-Kuang Chen, Robert Edelstein, Eric Hanushek, Sunny Kwong, John Quigley, Timothy Riddiough, Jacob Sagi, seminar participants of Chinese University of Hong Kong, City University of Hong Kong, Hong Kong Economics Association Meeting, International Conference on Real Estates and the Macroeconomy for conversations and comments; City University of Hong Kong for financial support. The usual disclaimer applies. ♣ Correspondence: Leung and Cheung: Department of Economics and Finance, City University of Hong Kong, Kowloon Tong, Hong Kong; (Phone) (852) 2788 9604; (Fax) (852) 2788 8806; (Email) [email protected]. … In the ruin of all collapsed booms is to be found the work of men who bought property at prices they knew perfectly well were fictitious, but who were willing to pay such prices simply because they knew that some still greater fool could be depended on to take the property off their hands and leave them with profit. From One Hundred Years of Land Values in Chicago, by Homer Hoyt … Usually the process starts with a trend that is not yet recognized… The trend becomes increasingly dependent on the bias and the bias becomes increasingly exaggerated. During this period, both the bias and the trend may be repeatedly tested by external shocks. If they survive the tests, they emerge strengthened until they become seemingly unshakable…. A point comes when the divergence between belief and reality becomes so great that the participants' bias comes to be recognized…. Eventually, the loss of belief is bound to cause a reversal in the trend…; this trend reversal is the crossover point.... When the process is complete, neither the trend nor the bias remains the same. The process does not repeat itself. There is a regime change.... From Soros on Soros: Staying Ahead of the Curve, by George Soros 1. Introduction Financial crises, and the possible negative consequences to the general economy, have always been a concern for academic researchers and policy makers alike. As the quotations above suggest, people may be aware of the “irrational component” of the asset pricing during the “boom”, yet participate anyway. Thus, it seems natural to conjecture that after a “crash” or a “crisis” in the asset market, a “structural change” would occur, and even affecting the relationship between the asset market and the 1 aggregate economy. 1 Studying this issue, however, is not straightforward. Corporations may change their management, the focus of their business, or even merge with other firms after a financial crisis, or even experience such changes during or just before the crisis. It makes the comparison across time periods non-trivial. On the other hand, housing units are typically indivisible, and display relatively less variations over time and hence housing research can contribute to the studies of financial crises. As a result, an emerging literature is developed to study the relationship between the financial crises and the housing market. Previous efforts typically focus on two major questions. First, is the real estate market a cause of a financial crisis? Second, what are the consequences of a financial crisis to the real estate market? To answer these questions, the literature either uses aggregate data (city or even national level) or very micro data (transaction or mortgage loan contract level).2 To complement the literature, this paper would rather focus on whether (and how) the interactions, price and trading volume correlations in particular, among different real estate developments, or simply estates, change after a financial crisis.3 Clearly, this question is relevant to several strands of the literature. First, it is related to the pricing of real estate.4 It is long recognized that the “stable factors” such as the reputation of the real estate developer and the specific location characteristics matter for the real estate pricing. It is especially true for residential housing as the school districts are proved to be an important empirical determinant for housing prices.5 The previous literature seems to focus on real estate market in more advanced countries where severe economy-wide crises are relatively rare. In contrast, this paper studies a real estate market which experiences a dramatic crisis and the interactions among different real estate developments potentially change. Since the “stable factors” do not 1 Clearly, it is beyond the scope of this paper to review this literature. See Eichengreen (2002), and the reference therein. 2 Clearly, it is beyond the scope of this paper to review this literature. See Gan (2006), Mera and Renuad (2000), Quigley (1999, 2001), Chen and Wang (2006), Leung (2004) and the reference therein. 3 An “estate” in Hong Kong is similar to a “housing development” in the United States. In Hong Kong, an estate is usually constituted by several high-rise buildings built by the same developers on a particular location, with similar if not identical designs and materials, and managed by the same company. This naturally produces a high degree of homogeneity among units which facilitates scientific studies. Size of some of the estates can make them form a distinct community., 4 As surveyed by Malpezzi (2002), the previous literature typically focuses on the functional form and the argument of the hedonic pricing equation. They usually pool all the data as one period and focus on the cross-sectional variations. 5 Again, it is beyond the scope of this paper to review the vast literature. See Weimer and Wolkoff (2001), Hanushek and Welch (2006) and the reference therein. 2 change in the crisis, any changes we can measure can be attributed to the other factors, such as the wealth effect on housing demand, or some expectation factors. This project is also related to the literature on the interaction between the banking sector and the real estate sector (for instance, see Chen and Wang, 2006; Gan, 2004; Zhu, 2002). They have very different focuses and did not explore the co- movement of different real estate developments. The strategic interactions among new real estate developments have been explored theoretically, using a real option approach (for instance, see Wang and Zhou, 2000, 2006; Wang et al, 2000). They did not employ micro-data to test their theory though. Perhaps more importantly, this paper is built on the “financial contagion” literature. Contagion can be defined in many ways (for instance, see Pericoli and Sbracia, 2003; Rigobon, 2003a, b), and the methodology of the empirical analysis is often chosen accordingly. Roughly speaking, the contagion literature investigates the situation where one asset (or market) receives an unfavorable shock and how other assets (or markets) are affected. Accordingly to Forbes and Rigobon (2002), contagion is ‘a significant increase in cross-market linkages after a shock to one country (or group of countries)’. Thus, the empirical tests based on correlation coefficients will measure the change (or increase) in the correlation in returns after a shock. Strictly speaking, since this paper studies the “inter-estate” interactions, it is not a situation of “financial contagion”. Nevertheless, the tools in that literature will prove to be applicable. Earlier literature tends to adopt the “sampling splitting approach”, which rely on the researchers to divide the full sample into “crisis” and “non-crisis” sub-samples, and then compare the estimated correlation coefficient for each sub- sample.6 Unfortunately, as shown by Forbes and Rigobon (2002, p.2229), “the tests for contagion based on correlation coefficient are biased and inaccurate due to heteroskedasticity in market returns”. An alternative approach is to convert correlation coefficients for different periods into a time series. There are a number of advantages for the researchers to adopt this approach, including a visualization of the fluctuations of correlation coefficient over time, and the ability to apply the time-series econometric techniques in investigating the impact of trend, structural breaks and long memory processes. A popular approach 6 Among others, see Solnik, Bourcrelle and Le Fur (1996), Forbes and Rigobon (2002) for a survey of that literature. 3 is to use the Dynamic Conditional Correlation (DCC) model, presented by Engle (2002) and Engle and Sheppard (2001), which is an extension of Bollerslev’s (1990) Constant Conditional Correlation (CCC) model. 7 However, DCC model may be difficult to implement with a large number of time series. In our context, we would need to estimate several hundred parameters for 36 estates with only 168 periods (14 years of monthly data), which is almost infeasible, and definitely not desirable. Thus, we follow Forbes and Rigobon (2002), which is more flexible with sample size, with some modifications. Specifically, we follow the “rolling regression approach” and estimate correlation coefficients within each ‘‘moving window’’, each with 24 monthly observations.8 This enables us to detect changes in the correlation estimates which are affected by abnormal events, or the “financial crisis shocks” we attempt to identify, given the limiting sample size. Notice that dataset is especially suitable to address our research questions.
Details
-
File Typepdf
-
Upload Time-
-
Content LanguagesEnglish
-
Upload UserAnonymous/Not logged-in
-
File Pages48 Page
-
File Size-