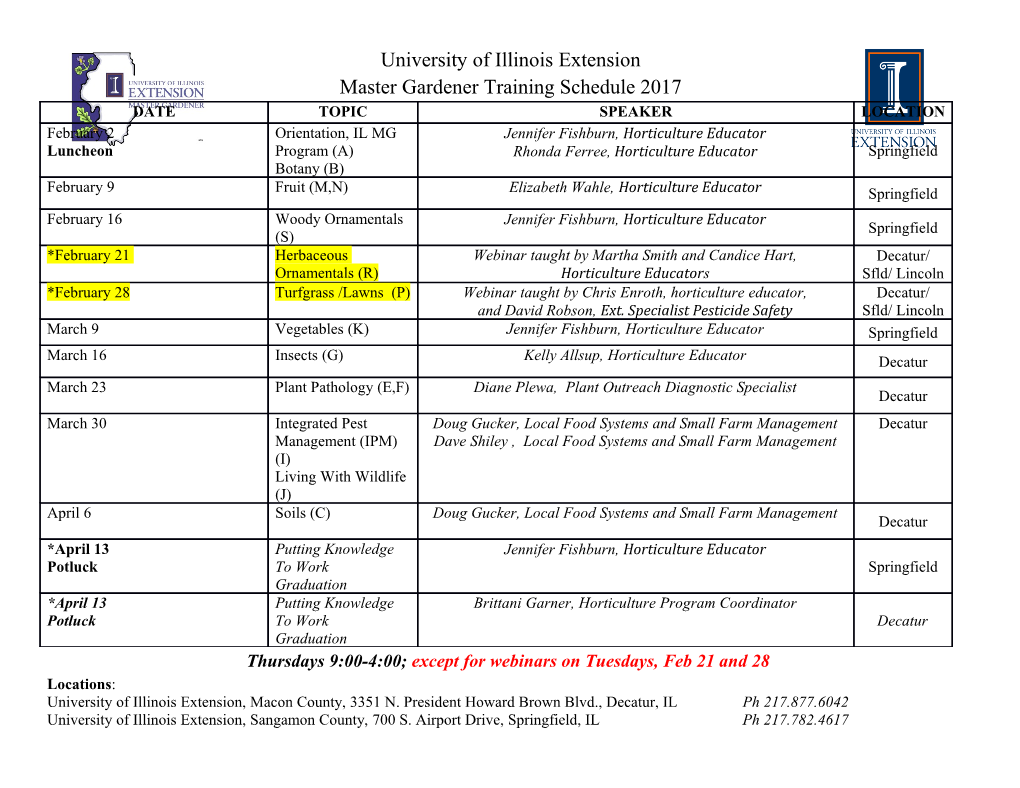
Journal of Arid Environments 178 (2020) 104153 Contents lists available at ScienceDirect Journal of Arid Environments journal homepage: www.elsevier.com/locate/jaridenv Modelling terrestrial reptile species richness, distributions and habitat suitability in Saudi Arabia T ∗ Abdulaziz S. Alatawia,b, , Francis Gilberta, Tom Readera a School of Life Sciences, University of Nottingham, University Park, Nottingham, NG7 2RD, UK b Biology Department, University of Tabuk, Tabuk City, Saudi Arabia ARTICLE INFO ABSTRACT Keywords: Species distribution modelling is a powerful tool that can gives us ecological insights about species distributions, Habitat suitability and potential effects of environmental factors, in poorly known habitats. For the first time the distribution of Maxent terrestrial reptiles in Saudi Arabia was modelled, and environmental factors that affect their current distribution Saudi Arabia and richness investigated. Reptiles are a major vertebrate group in Saudi Arabia and protecting them should be a Species distribution modelling priority for conservation in such an arid environment. Temperature was the most important of eleven predictors. Species richness Maximum species richness of reptiles was predicted in the central plateau, north-western borders, and in coastal Terrestrial reptiles areas of Saudi Arabia. Overall, the predicted and the observed patterns of species richness followed a similar pattern. Our analysis revealed that large scattered parts of Saudi Arabia are considered under-sampled in terms of sampling efforts of terrestrial reptile species. Our results represent the most comprehensive description of terrestrial reptile diversity distributions and habitat suitability in Saudi Arabia to date. 1. Introduction (Yi et al., 2016). The distribution of species richness is influenced by many biotic and abiotic factors; understanding and measuring these How and why species are distributed in space and time is considered factors and how they influence the distribution of species is critical for a fundamental scientific question, especially in ecology (Rushton et al., proper conservation planning (Brown et al., 2007). However, it is not 2004; Guisan and Thuiller, 2005; Brotons, 2014). Humans have ob- an easy task to model the spatial distribution of the richness of species, served the unique relationship that connects living organisms with their especially across large-scale areas (Pineda and Lobo, 2009). The gap in surrounding environment (Guisan and Thuiller, 2005; Elith and current knowledge about the distribution of species richness and the Leathwick, 2009), and for more than a century scientists have tried to suitability of habitats could be fixed with enough biological data ob- come up with theoretical or experimental explanations for spatial and tained from surveys, and accurate evidence of spatial patterns in en- temporal patterns of presence/absence and abundance (Elton, 1927; vironmental variables; these can be combined to produce predictive MacArthur and Wilson, 1967). Today, many important scientific ap- maps of species richness (Lobo et al., 2002; Graham and Hijmans, plications depend on understanding this relationship, such as natural 2006). These descriptive maps of species richness are considered a basic resource management, predicting the impacts of climate change and tool in conservation decision-making (Lobo et al., 2002; Graham and invasive species, and conservation planning more generally (Guisan and Hijmans, 2006). Zimmermann, 2000; Guisan and Thuiller, 2005; Peterson et al., 2011). Techniques for modelling species distributions have developed ra- However, despite considerable progress in methods for understanding pidly in the last two decades (Guisan and Zimmermann, 2000; Guisan spatial and temporal patterns, our knowledge of contemporary levels of and Thuiller, 2005; Elith and Leathwick, 2009). Correlative species biodiversity and the distribution of species richness in many parts of the distribution models (SDMs) (also called ecological niche modelling, world remains poor (Mora et al., 2011; Ficetola et al., 2013). more details in Sillero, 2011) usually work by combining known species Species richness is a key measure of biodiversity and its study can occurrence records with spatial layers of digital environmental data, illuminate important ecological phenomena, such as the species-area and using statistical techniques to estimate geographical distribution relationship, and rules for community assembly (Brown et al., 2007). and habitat suitability for species over an area of interest (Guisan and Species richness is connected with the quality and quantity of available Zimmermann, 2000; Guisan and Thuiller, 2005; Sillero, 2011). The habitat, and habitat degradation can adversely affect species richness main objective is to generate new predicted distribution maps that ∗ Corresponding author. School of Life Sciences, University of Nottingham, University Park, Nottingham, NG7 2RD, UK. E-mail addresses: [email protected], [email protected] (A.S. Alatawi). https://doi.org/10.1016/j.jaridenv.2020.104153 Received 30 November 2018; Received in revised form 12 February 2020; Accepted 2 March 2020 0140-1963/ © 2020 Elsevier Ltd. All rights reserved. A.S. Alatawi, et al. Journal of Arid Environments 178 (2020) 104153 capture and explain the species-environment association (Guisan and Thus far, there have only been two attempts (to our knowledge) to Zimmermann, 2000; Guisan and Thuiller, 2005) which, if presence-only estimate the distribution of reptile diversity in Saudi Arabia. Cox et al. models are used, effectively represents habitat suitability for the target (2012) performed a regional assessment for IUCN Red-listing of the species (see Sillero, 2011). These maps show how habitat suitability majority of the reptiles of the Arabian Peninsula. By overlaying simple varies through space and time, by identifying locations in the study area range-distribution polygons for each species, they predicted that high that have environmental conditions similar to those where the species species-richness locations in Saudi Arabia would include the long chain has been found (Pearson, 2008; Sillero, 2011). However, despite con- of south-western mountains, the coast of the Eastern Province and a few siderable progress in the development of these techniques, predicting locations in northern areas. Locations expected to have the lowest species distributions is still a complex and challenging process (Merow species richness were in the Empty Quarter (the Rub’ al Khali), and et al., 2014). central southern areas. A more detailed species distribution model was Many advanced modelling techniques/tools and quantitative developed by Wilms et al. (2011) for just one species in Saudi Arabia - methods have been developed and applied in distribution modelling, Uromastyx aegyptia microlepis - as part of their studies on its thermal including various forms of regression analysis (generalized linear biology and activity patterns. models [GLMs], generalized additive models [GAMs], and machine- Our objective in this paper is to model the distribution of reptiles in learning methods Maximum Entropy [Maxent], and artificial neural general, using presence-only data, and map their potential richness, and networks [ANN]) (Peterson et al., 2011). All of these methods can be their habitat suitability in Saudi Arabia using Maxent. Maxent is cap- used to model potential species richness, but each uses a different able of capturing complex non-linear response curves using a set of process to generate the final distribution map. Some modelling tech- “features” (e.g. linear, quadratic, product, threshold; see Merow et al., niques require presence-absence data (e.g., GLM) while others require 2013; Phillips et al., 2017). The distribution predictions made by only presence data as they can generate background points during Maxent are known to perform well, its regularization procedure ob- modelling (e.g., Maxent) (Phillips et al., 2006). Choosing which method viating over-fitting (Merow et al., 2013). We built models of the spatial to apply depends on the available data and the purpose of the study distribution of individual reptile species as a function of environmental (Elith and Leathwick, 2009). variables in order to understand the magnitude of the impact of these Reptiles are widely distributed around the world and are considered variables on the species concerned. We then combined these models to important components of local ecosystems and biodiversity (AbuZinada produce two maps to describe predicted patterns of diversity across et al., 2004; Carranza et al., 2018). Due to their ectothermy, reptiles are Saudi Arabia. One map shows the summed probability of species oc- sensitive to the thermal characteristics of their environment (Wilms currence, and the other shows the expected species richness assuming a et al., 2011; Carranza et al., 2018), and they demonstrate various threshold rule to convert continuous habitat suitability values into a special adaptations as a result (Metallinou et al., 2015). Because their binary prediction of suitable/not suitable. The predictions that we response to temperature variation is relatively well understood, they produce represents the most comprehensive attempt to understand the can be used as indicators to assess the impact of global warming on the current distribution of terrestrial reptiles in Saudi Arabia to date. environment (Wilms et al., 2011). Today, many reptiles are seriously threatened
Details
-
File Typepdf
-
Upload Time-
-
Content LanguagesEnglish
-
Upload UserAnonymous/Not logged-in
-
File Pages9 Page
-
File Size-