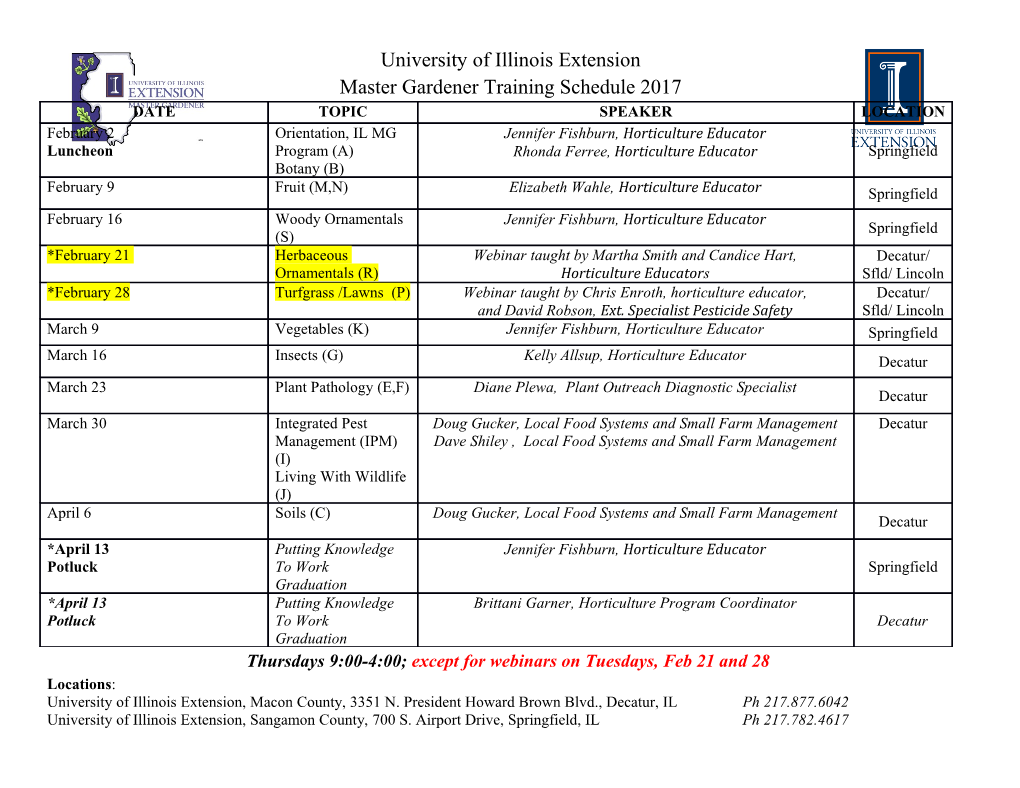
Basics Endogenous regressors Maximum likelihood Limited dependent variables Panel data Microeconometrics Based on the textbooks Verbeek: A Guide to Modern Econometrics and Cameron and Trivedi: Microeconometrics Robert M. Kunst [email protected] University of Vienna and Institute for Advanced Studies Vienna October 21, 2013 Microeconometrics University of Vienna and Institute for Advanced Studies Vienna Basics Endogenous regressors Maximum likelihood Limited dependent variables Panel data Outline Basics Ordinary least squares The linear regression model Goodness of fit Restriction tests on coefficients Asymptotic properties of OLS Endogenous regressors Maximum likelihood Limited dependent variables Panel data Microeconometrics University of Vienna and Institute for Advanced Studies Vienna Basics Endogenous regressors Maximum likelihood Limited dependent variables Panel data What is micro-econometrics? Micro-econometrics is concerned with the statistical analysis of individual (not aggregated) economic data. Some aspects of such data imply special emphasis: ◮ Samples are larger than for economic aggregates, often thousands of observations are available; ◮ Non-linear functional dependence may be identified from data (for aggregates linear or pre-specified functions must suffice); ◮ Qualitative or limited-dependent variables are common; ◮ Individuals can often be regarded as independent, random sampling assumptions can be plausible (no serial correlation); ◮ Individuals are often heterogeneous: heteroskedasticity; ◮ The logical sequences in time are unavailable. Cause and effect are identified from theory, not from the data. Microeconometrics University of Vienna and Institute for Advanced Studies Vienna Basics Endogenous regressors Maximum likelihood Limited dependent variables Panel data Ordinary least squares Linear regression: ordinary least squares There are N observations on a ‘dependent’ variable y and on K 1 ‘explanatory’ variables (regressors) x ,..., xK . The − 2 best-fitting linear combination that minimizes N ′ 2 S(β˜)= (yi x β˜) − i i =1 ′ is the OLS (ordinary least squares) vector b = (b1,..., bK ) . ′ Notation: x 1 but x = (1, x ,..., x K ). 1 ≡ 1 12 1 Microeconometrics University of Vienna and Institute for Advanced Studies Vienna Basics Endogenous regressors Maximum likelihood Limited dependent variables Panel data Ordinary least squares The normal equations of OLS To minimize S(β˜), equate its derivative to 0 (‘normal equations’): N N N ′ ′ 2 xi (yi x b) = 0 ∴ xi x b = xi yi , − − i i i i i =1 =1 =1 ′ or simply, in matrix and vector notation, using y = (y1,..., yN ) ′ and X = (x1,..., xN ) , ′ − ′ b = (X X ) 1X y. ′ Notation: Here, xi = (1, xi2,..., xiK ) . Microeconometrics University of Vienna and Institute for Advanced Studies Vienna Basics Endogenous regressors Maximum likelihood Limited dependent variables Panel data Ordinary least squares yˆ and residuals The (‘systematic’ or ‘predicted’) value ′ yˆi = xi b is the best linear approximation of y from x2,..., xK , the best approximation by linear combinations. The difference between y andy ˆ is called the residual ′ ei = yi yˆi = yi x b. − − i Microeconometrics University of Vienna and Institute for Advanced Studies Vienna Basics Endogenous regressors Maximum likelihood Limited dependent variables Panel data Ordinary least squares Residual sum of squares The function S(.) evaluated at its minimum N N 2 2 S(b)= (yi yˆi ) = e − i i i =1 =1 is the residual sum of squares (RSS). Because of the normal equations, N N ′ xi (yi x b)= xi ei = 0, − i i i =1 =1 such that residuals and regressors are orthogonal. Also, N N ′ ′ ei =0= (yi x b) =y ¯ x¯ b − i − i i =1 =1 ory ¯ =x ¯′b. Sample averages are on the regression hyperplane. Microeconometrics University of Vienna and Institute for Advanced Studies Vienna Basics Endogenous regressors Maximum likelihood Limited dependent variables Panel data Ordinary least squares Simple linear regression The case K = 2 is called the simple linear regression. Geometrically, a straight line is fitted through points xi , yi . Note the property b =y ¯ b x¯ 1 − 2 for the intercept. Starting from (X ′X )−1X ′y, some algebra yields N i=1(xi x¯)(yi y¯) b2 = − − N 2 (xi x¯) i=1 − for the slope. Microeconometrics University of Vienna and Institute for Advanced Studies Vienna Basics Endogenous regressors Maximum likelihood Limited dependent variables Panel data Ordinary least squares A dummy regressor If xi = 1 for some observations and xi = 0 otherwise (man/woman, employed/unemployed, before/after 1989), x is called a dummy variable.x ¯ will be the share of 1’s in the sample. b1 is the average y for the 0 individualsy ¯[0], and b2 is the difference betweeny ¯[1] andy ¯[0]. Microeconometrics University of Vienna and Institute for Advanced Studies Vienna Basics Endogenous regressors Maximum likelihood Limited dependent variables Panel data Ordinary least squares Linear regression and matrix notation Most issues are simpler and more compact in matrix notation: ′ 1 x12 . x1K x1 y1 . X = . = . , y = . . 1 x . x x′ y N2 NK N N The sum of squares to be minimized is now ′ ′ ′ ′ ′ S(β˜) = (y X β˜) (y X β˜)= y y 2y X β˜ + β˜ X X β,˜ − − − and ∂S(β˜)/∂β˜ = 0 yields immediately b = (X ′X )−1X ′y. Microeconometrics University of Vienna and Institute for Advanced Studies Vienna Basics Endogenous regressors Maximum likelihood Limited dependent variables Panel data Ordinary least squares The projection matrix The vector of observations is the sum of its systematic part and the residuals ′ − ′ y = Xb + e = X (X X ) 1X y + e =y ˆ + e. The matrix PX that transforms y into its systematic part ′ −1 ′ yˆ = X (X X ) X y = PX y is called the projection matrix. It is singular, N N of rank K, × and PX PX = PX . Note the orthogonality PX (I PX ) = 0 − to the matrix MX = I PX that transforms y to residuals e. − Microeconometrics University of Vienna and Institute for Advanced Studies Vienna Basics Endogenous regressors Maximum likelihood Limited dependent variables Panel data The linear regression model Linear regression in a statistical model ◮ Without a statistical frame, regression is a mere descriptive device. The OLS coefficients are not estimates. They can be calculated, as long as X ′X is non-singular (no multicollinearity); ◮ The classical didactic regression model assumes stochastic (random) errors and non-stochastic regressors. Current textbooks tend to avoid that model; ◮ The stochastic regression model assumes X and y to be realizations of random variables. Under conditions, operations can be conducted conditional on X , and most classical results can be retrieved. Microeconometrics University of Vienna and Institute for Advanced Studies Vienna Basics Endogenous regressors Maximum likelihood Limited dependent variables Panel data The linear regression model Main ingredients of the statistical regression model In the regression in matrix notation y = X β + ε, y and X collect realizations of observable random variables, β contains K unobserved non-random parameters, and ε collects realizations of an unobserved random variable, the error term. The assumption of random sampling implies that observations are independent across the index i (the rows). A non-stochastic regressor has the same values in a different sample. A deterministic regressor has known values before sampling (constant, trend). Microeconometrics University of Vienna and Institute for Advanced Studies Vienna Basics Endogenous regressors Maximum likelihood Limited dependent variables Panel data The linear regression model Exogenous regressors y is often called the dependent variable, xk , k = 1,..., K, are the regressors or covariates (usage of the word ‘independent variable’ is discouraged). If the exogeneity assumption on the regressors E[εi xi ] = 0 | ′ holds, it follows that E[yi xi ]= x β and also that E[εi ]= 0. With | i non-stochastic regressors, this exogeneity assumption is unnecessary. (There are also other definitions of exogeneity) Microeconometrics University of Vienna and Institute for Advanced Studies Vienna Basics Endogenous regressors Maximum likelihood Limited dependent variables Panel data The linear regression model Estimator and estimate An estimate is a statistic obtained from a sample that is designed to approximate an unknown parameter closely: b is an estimate for β. The unknown but fixed β is called a K–dimensional parameter or a K–vector of scalar parameters (real numbers). In regression, b may be called the coefficient estimates, β may be called the coefficients. An estimator is a rule that says how to obtain the estimate from data. OLS is an estimator. The residual ei approximates the true error term εi but it is not an estimate, as εi is not a parameter. Don’t be sloppy, never confuse errors and residuals. Microeconometrics University of Vienna and Institute for Advanced Studies Vienna Basics Endogenous regressors Maximum likelihood Limited dependent variables Panel data The linear regression model Gauss-Markov conditions The Gauss-Markov conditions imply nice properties for the OLS estimator. In particular, for the linear regression model ′ yi = xi β + εi , assume A1 Eεi = 0, i = 1,..., N; A2 ε ,...,εN and x ,..., xN are independent; { 1 } { 1 } 2 A3 Vεi = σ , i = 1,..., N; A4 cov(εi , εj ) = 0, i, j = 1,..., N, i = j. (A1) identifies the intercept, (A2) is an exogeneity assumption, (A3) is called the homoskedasticity assumption, (A4) assumes the absence of autocorrelation. Microeconometrics University of Vienna and Institute for Advanced Studies Vienna Basics Endogenous regressors Maximum likelihood Limited dependent variables Panel data The linear regression model Some implications of the Gauss-Markov assumptions ◮ (A1), (A3), (A4) imply for the vector random variable ε that 2 Eε = 0 and V(ε)= σ IN , a ‘scalar matrix’; ◮ 2 (A1)–(A4) imply that also E(ε X )=0 and V(ε X )= σ IN , | | other types of exogeneity assumptions; ◮ (A1) and (A2) imply that E(y X )= X β. | Microeconometrics University of Vienna and Institute for Advanced Studies Vienna Basics Endogenous regressors Maximum likelihood Limited dependent variables Panel data The linear regression model Unbiasedness of OLS Under assumptions (A1) and (A2), the OLS estimator is unbiased, i.e.
Details
-
File Typepdf
-
Upload Time-
-
Content LanguagesEnglish
-
Upload UserAnonymous/Not logged-in
-
File Pages37 Page
-
File Size-