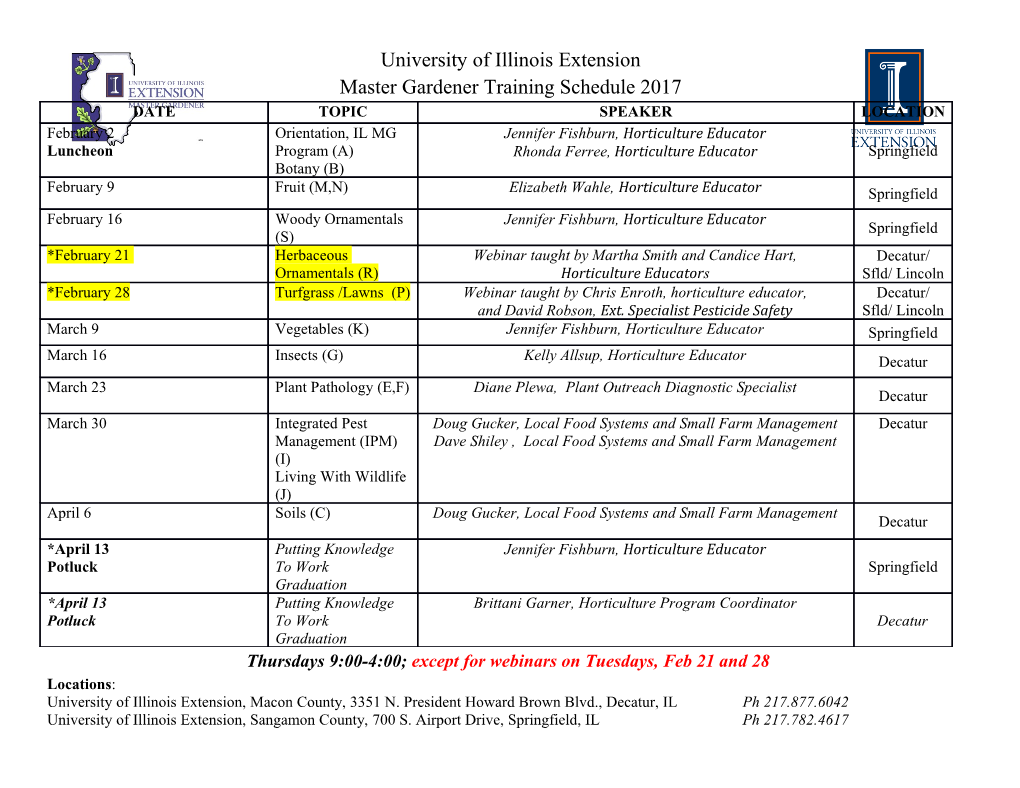
bioRxiv preprint doi: https://doi.org/10.1101/089391; this version posted December 20, 2017. The copyright holder for this preprint (which was not certified by peer review) is the author/funder. All rights reserved. No reuse allowed without permission. Running Head: PREDICTING CELL TYPE BALANCE INFERENCE OF CELL TYPE COMPOSITION FROM HUMAN BRAIN TRANSCRIPTOMIC DATASETS ILLUMINATES THE EFFECTS OF AGE, MANNER OF DEATH, DISSECTION, AND PSYCHIATRIC DIAGNOSIS *Megan Hastings Hagenauer, Ph.D.1, Anton Schulmann, M.D.2, Jun Z. Li, Ph.D.3, Marquis P. Vawter, Ph.D.4, David M. Walsh, Psy.D.4, Robert C. Thompson, Ph.D.1, Cortney A. Turner, Ph.D.1, William E. Bunney, M.D.4, Richard M. Myers, Ph.D.5, Jack D. Barchas, M.D.6, Alan F. Schatzberg, M.D.7, Stanley J. Watson, M.D., Ph.D.1, Huda Akil, Ph.D.1 1Mol. Behavioral Neurosci. Inst., Univ. of Michigan, Ann Arbor, MI, USA; 2 Janelia Research Campus, Howard Hughes Medical Institute, Ashburn, VA, USA, 3Genet., Univ. of Michigan, Ann Arbor, MI, USA; 4Univ. of California, Irvine, CA; 5HudsonAlpha Inst. for Biotech., Huntsville, AL, USA; 6Stanford, Palo Alto, CA, 7Cornell, New York, NY, USA *Corresponding Author: Megan Hastings Hagenauer, Ph.D. e-mail: [email protected] Molecular Behavioral Neuroscience Institute (MBNI) 205 Zina Pitcher Pl. Ann Arbor, MI 48109 1 bioRxiv preprint doi: https://doi.org/10.1101/089391; this version posted December 20, 2017. The copyright holder for this preprint (which was not certified by peer review) is the author/funder. All rights reserved. No reuse allowed without permission. Running Head: PREDICTING CELL TYPE BALANCE ABSTRACT 4 1. INTRODUCTION 5 2. METHODS & VALIDATION 7 2.1 Compiling a Database of Cell Type Specific Transcripts 7 2.2 “BrainInABlender”: Employing the Database of Cell Type Specific Transcripts to Predict Relative Cell Type Balance in Heterogenous Brain Samples 10 2.3 Validation of Relative Cell Content Predictions Using Datasets Derived from Purified or Cultured Cells 11 2.4 Validation of Relative Cell Content Predictions Using a Dataset Derived from Human Post- Mortem Tissue 15 2.5 Using Cell Type Specific Transcripts to Predict Relative Cell Content in Transcriptomic Data from Macro-Dissected Human Cortical Tissue from Psychiatric Subjects 16 2.6 Does the Reference Dataset Matter? Cell Type Specific Transcripts Identified by Different Publications Produce Similar Predictions of Relative Cell Type Balance 18 3. RESULTS 19 3.1 Inferred Cell Type Composition Explains a Large Percentage of the Sample-Sample Variability in Microarray Data from Macro-Dissected Human Cortical Tissue 19 3.2 Cell Content Predictions Derived from Microarray Data Match Known Relationships Between Clinical/Biological Variables and Brain Tissue Cell Content 22 3.3 Cell Type Balance Changes in Response to Psychiatric Diagnosis 29 3.4 Discriminating Between Changes in Cell Type Balance and Cell-Type Specific Function 30 3.5 Including Cell Content Predictions in the Analysis of Microarray Data Improves Model Fit And Enhances the Detection of Diagnosis-Related Genes in Some Datasets 32 4. DISCUSSION 39 5. ACKNOWLEDGEMENTS 44 6. REFERENCES 45 7. SUPPORTING INFORMATION 55 7.1 Detailed Methods and Results: Using Cell Type Specific Transcripts to Predict Relative Cell Content in Datasets from Purified Cells and Artificial Cell Mixtures 56 2 bioRxiv preprint doi: https://doi.org/10.1101/089391; this version posted December 20, 2017. The copyright holder for this preprint (which was not certified by peer review) is the author/funder. All rights reserved. No reuse allowed without permission. Running Head: PREDICTING CELL TYPE BALANCE 7.2 Comparison of Our Method vs. PSEA: Predicting Cell Identity in a Human Single-Cell RNA-Seq Dataset 68 7.3 Additional Detailed Preprocessing Methods for the Macro-Dissected Microarray Datasets 76 7.3.1 Pritzker Dorsolateral Prefrontal Cortex Microarray Dataset (GSE92538) 76 7.3.2 Allen Brain Atlas Cross-Regional Microarray Dataset 78 7.3.3 Human Cortical Microarray Dataset GSE53987 (submitted to GEO by Lanz et al. (36)) 79 7.3.4 Human Cortical Microarray Dataset GSE21138 (submitted to GEO by Narayan et al. (38)) 79 7.3.5 Human Cortical Microarray Dataset GSE21935 (submitted to GEO by Barnes et al. (37)) 80 7.3.6 CommonMind Consortium Human Cortical RNA-Seq Dataset 81 7.4 Additional figures and results: Does the Reference Dataset Matter? There is a Strong Convergence of Cell Content Predictions Derived from Cell Type Specific Transcripts Identified by Different Publications 81 7.5 Additional figures and results: Cell Type Indices Predict Other Genes Known to Be Cell Type Enriched 91 7.6 Additional figures and results: Inferred Cell Type Composition Explains a Large Percentage of the Sample-Sample Variability in Microarray Data from Macro-Dissected Human Cortical Tissue 94 7.7 Additional figures and results: Discriminating Between Changes in Cell Type Balance and Cell- Type Specific Function 98 7.8 The Top Diagnosis-Related Genes Identified by Models that Include Cell Content Predictions Pinpoint Known Risk Candidates 99 8. SUPPLEMENTARY TABLES 105 3 bioRxiv preprint doi: https://doi.org/10.1101/089391; this version posted December 20, 2017. The copyright holder for this preprint (which was not certified by peer review) is the author/funder. All rights reserved. No reuse allowed without permission. Running Head: PREDICTING CELL TYPE BALANCE Abstract Psychiatric illness is unlikely to arise from pathology occurring uniformly across all cell types in affected brain regions. Despite this, transcriptomic analyses of the human brain have typically been conducted using macro-dissected tissue due to the difficulty of performing single-cell type analyses with donated post-mortem brains. To address this issue statistically, we compiled a database of several thousand transcripts that were specifically-enriched in one of 10 primary cortical cell types, as identified in previous publications. Using this database, we predicted the relative cell type composition for 833 human cortical samples using microarray or RNA-Seq data from the Pritzker Consortium (GSE92538) or publicly-available databases (GSE53987, GSE21935, GSE21138, CommonMind Consortium). These predictions were generated by averaging normalized expression levels across transcripts specific to each cell type using our R-package BrainInABlender (validated and publicly-released: https://github.com/hagenaue/BrainInABlender). Using this method, we found that the principal components of variation in the datasets were largely explained by the neuron to glia ratio of the samples. This variability was not simply due to dissection – the relative balance of brain cell types was influenced by a variety of demographic, pre- and post-mortem variables. Prolonged hypoxia around the time of death predicted increased astrocytic and endothelial content in the tissue, illustrating vascular upregulation. Aging was associated with decreased neuronal content. Red blood cell content was reduced in individuals who died following systemic blood loss. Subjects with Major Depressive Disorder had decreased astrocytic content, mirroring previous morphometric observations. Subjects with Schizophrenia had reduced red blood cell content, resembling the hypofrontality detected in fMRI experiments. Finally, in datasets containing samples with especially variable cell content, we found that controlling for predicted sample cell content while evaluating differential expression improved the detection of previously- identified psychiatric effects. We conclude that accounting for cell type can greatly improve the interpretability of microarray data. 4 bioRxiv preprint doi: https://doi.org/10.1101/089391; this version posted December 20, 2017. The copyright holder for this preprint (which was not certified by peer review) is the author/funder. All rights reserved. No reuse allowed without permission. Running Head: PREDICTING CELL TYPE BALANCE 1 1. Introduction 2 The human brain is a remarkable mosaic of diverse cell types stratified into rolling cortical layers, 3 arching white matter highways, and interlocking deep nuclei. In the past decade, we have come to 4 recognize the importance of this cellular diversity in even the most basic neural circuits. At the same time, 5 we have developed the capability to comprehensively measure the thousands of molecules essential for 6 cell function. These insights have provided conflicting priorities within the study of psychiatric illness: do 7 we carefully examine individual molecules within their cellular and anatomical context or do we extract 8 transcript or protein en masse to perform large-scale unbiased transcriptomic or proteomic analyses? In 9 rodent models, researchers have escaped this dilemma by a boon of new technology: single cell laser 10 capture, cell culture, and cell-sorting techniques that can provide sufficient extract for transcriptomic and 11 proteomic analyses. However, single cell analyses of the human brain are far more challenging (1–3) – 12 live tissue is only available in the rarest of circumstances (such as temporal lobe resection) and intact 13 single cells are difficult to dissociate from post-mortem tissue without intensive procedures like laser 14 capture microscopy. 15 Therefore, to date, the vast majority of unbiased transcriptomic analyses of the human brain have 16 been conducted using macro-dissected, cell-type heterogeneous tissue. On Gene Expression Omnibus 17 alone, there are at least 63* publicly-available macro-dissected post-mortem human brain tissue datasets,
Details
-
File Typepdf
-
Upload Time-
-
Content LanguagesEnglish
-
Upload UserAnonymous/Not logged-in
-
File Pages107 Page
-
File Size-