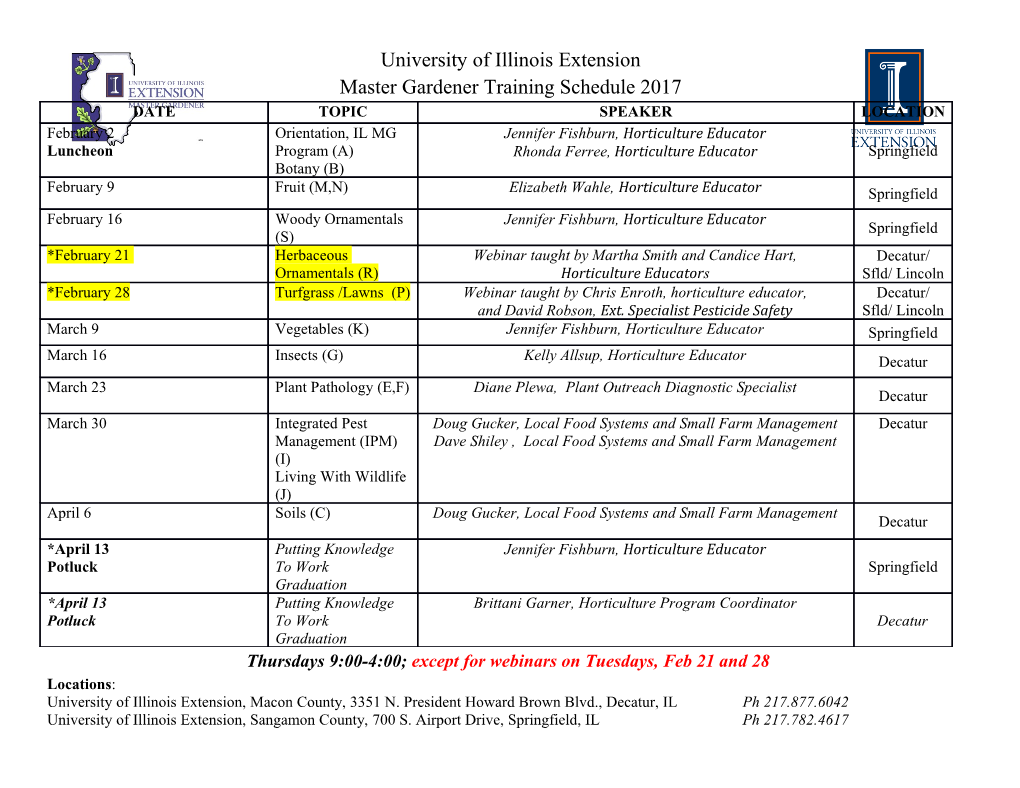
Calculation of Standard Error of the Variance and Standard Deviation October 12, 2009 Consider sampling a distribution N times obtaining the set of measured values Xi. The 0 probability distribution being sampled has a true mean of µ1. Likewise moments and cen- tral moments (moments about the mean) can be computed from this distribution, denoted 0 by µr, µr for the rth moment and central moment respectively. The sample moments 0 mr, mr can be computed from the sampled values Xi via N 1 X m0 = Xr (1) r N i i=1 N 1 X m = (X − m0 )r (2) r N i 1 i=1 If we consider the expectation value of the sample mean we have " N # N 1 X 1 X E[m0 ] = E X = E[X ] (3) 1 N i N i i=1 i=1 Where in the last step the expectation value can be taken inside the summation symbol because the Xi’s are uncorrelated. For a given measurement the expected value of X is the true mean N 1 X E[m0 ] = µ0 = µ0 (4) 1 N 1 1 i=1 This result is true for any moment, but not for any central moment because of the corre- lations between a moment of Xi and the fact that Xi appears in the sample mean. 0 0 E[mr] = µr (5) E[mr] 6= µr (6) 1 However, it can be shown that these quantities differ only by terms O(N −1) which become increasingly negligible as N becomes large. For the case of the second central moment the calculation of these terms is quite tractable. This will be used to compute the variance of the second central moment as an estimate of its uncertainty. We will use a property of central moments to dramatically simplify this calculation. Consider Yi = Xi + α, where α X Y is a constant. If mr and mr are the rth central moments of X and Y then X Y mr = mr (7) X 0 Y 0 This follows from the fact that the first moments are related by (m1 ) = (m1 ) + α which then implies that Y 0 X 0 X 0 Yi − (m1 ) = Xi + α − (m1 ) − α = Xi − (m1 ) (8) If the value of α is chosen to be the true mean of the distribution (for X) then E[Yi] = 0. Making this substitution allows us to eliminate single powers of Xi in computing expecta- tion values. As an example, consider the calculation of the expected value of the second central moment of X " N # " N # 1 X 0 2 1 X 2 0 02 E[m2] = E N (Xi − m1) = N E Xi − 2Xim1 + m1 i i N N N N X 2 X 1 X X = 1 E X2 − X X + X X (9) N i N i j N 2 j k i j j k The expectation value cannot be taken inside the summation since there is a correlation between Xi and Xj if i = j. Therefore we must break each sum into two pieces N N N N N X 2 X 1 X X X E[m ] = 1 E X2 − X X + X + X2 + X X 2 N i N i i j N 2 j j k i j, j6=i j j k, k6=j N N N X 2 1 2 1 X X = 1 1 − + E[X2] + − + E[X X ] N N N i N N i j i i j, j6=i 1 1 2 1 2 = N N(1 − N )E[X ] − N(N − 1) N E[X] N−1 0 02 N−1 = N µ2 − µ1 = N µ2 (10) The aforementioned trick can be used to simplify this calculation. We could have instead calculated the same moment for Y , which is the same as for X as described above. Since E[Y ] = 0 we could drop the second expectation value in the second line of the previous equation. Also E[Y 2] is a central moment by definition which eliminates the last step on 2 the last line. By employing this trick we save the effort in keeping track of the linear terms when expanding the sums and then recombining them at the end to express the answer in terms of a central moment. While this is perhaps overkill for the example, it is certainly 2 useful in the case of E[m2] which we compute next. 2 N N N N 1 X 2 X 1 X X E[m2] = E X2 − X X + X X (11) 2 N 2 i N i j N 2 j k i j j k The expression in parentheses has 6 terms N !2 N !4 N !4 X 4 X 1 X X2 + X + X i N 2 i N 2 i i i i 2 2 N N N N N !4 4 X X 2 X X 4 X − X2 X + X2 X − X N i j N i j N 2 i i j i j i 2 N !2 N N N !4 X 2 X X 1 X = X2 − X2 X + X (12) i N i j N 2 i i i j i Now we can expand all of the sums in eqn. 12 N !2 N N N X 2 X 4 X X 2 2 Xi = Xi + Xi Xj (13) i i i j, j6=i 2 N N N N N N X 2 X X 2 X 2 X X Xi Xj = Xi Xj + XjXk i j i j j k, k6=j N N N N N X 4 X X 2 2 X X 3 = Xi + Xi Xj + Xi Xj i i j, j6=i i j, j6=i N N N X X X 2 + Xi XjXk (14) i j,j6=i k, k6=i,j N !4 N N N N N X X 4 X X 3 X X 2 2 Xi = Xi + 4 Xi Xj + 3 Xi Xj i i i j, j6=i i j, j6=i N N N N N N N X X X 2 X X X X +12 Xi XjXk + 24 XiXjXkXl (15) i j, j6=i k, k6=i,j i j, j6=i k, k6=i,j l, l6=i,j,k 3 We can use the same trick we used in calculating mr here. Thus we can drop all terms containing a single power of Xi. Plugging this in to eqn. 12 we get N N N 2 1 X 2 3 X X 1 − + X4 + 1 − + X2X2 (16) N N 2 i N N 2 i j i i j, j6=i N N N 1 X X X E[m2] = E (N − 1)2 X4 + (N 2 − 2N + 3) X2X2 (17) 2 N 4 i i j i i j, j6=i N N N 1 X X X E[m2] = (N − 1)2 E[X4] + (N 2 − 2N + 3) E[X2]E[X2] (18) 2 N 4 i i j i i j, j6=i In the second term expectation value of the product factorizes since the restricted sum enforces the condition that the two variables are independent. There are N possible values for i and N − 1 values for j 2 1 2 2 2 E[m2] = N 4 (N − 1) Nµ4 + (N − 2N + 3)N(N − 1)µ2 (19) (N − 1)2 µ N − 1 V ar[m ] = E[m2] − E[m ]2 = 4 + µ2 N 2 − 2N + 3 − N(N − 1) 2 2 2 N 2 N N 3 2 (N − 1)2 µ N − 1 = 4 + µ2 (2 − (N − 1)) N 2 N N 3 2 (N − 1)2 µ − µ2 2(N − 1) = 4 2 + µ2 (20) N 2 N N 3 2 This form shows the behavior at large N explicitly. In this case µ − µ2 V ar[m ] ' 4 2 (21) 2 N This is true for N 1. If N is large enough for the Central Limit Theorem to apply then 4 µ4 = 3σ for the Normal Distribution and we have 2σ4 V ar[m ] ' (22) 2 N Now we typically estimate the uncertainty on a quantity computed from statistical sampling by the square root of its variance. For the case of interest we wish to find the uncertainty on the standard deviation. We know the uncertainty in its square to be the square root of the variance of the second central moment (sample variance) which was the result of eqn. 20. √ 2 √ √ δ[( m2 ) ] = 2 m2δ[ m2 ] (23) s √ 2 2 µ4 √ δ[( m2 ) ] N − 1 σ2 − 1 N − 1 δ[ m2 ] = √ = − 3 (24) 2 m2 N 4N 2N 4 1 Application to Weighted Samples In the case of the jet analysis, for each ET bin we have sampled the distribution for ∆ET values for a given jet sample J; his distribution is P (i|J), where i denotes the ith ∆ET bin and we have suppressed the ET index. We want to use these samplings to determine a sample mean and standard deviation (with appropriate statistical errors) for the total ∆E distribution T X P (i) = P (i|J)P (J) (25) J Where P (J) = σJ /σ is the known ratio of the cross sections. To make statements about the distribution P (i) one makes samples the distribution and computes statistical moments. The inference is that the values of the sample moments are estimates of the true moments in the fashion of eqn. 5. However we can alternatively make statements about the true moments (and consequently the true distribution) by sampling the conditional probabilities and computing their moments.
Details
-
File Typepdf
-
Upload Time-
-
Content LanguagesEnglish
-
Upload UserAnonymous/Not logged-in
-
File Pages10 Page
-
File Size-