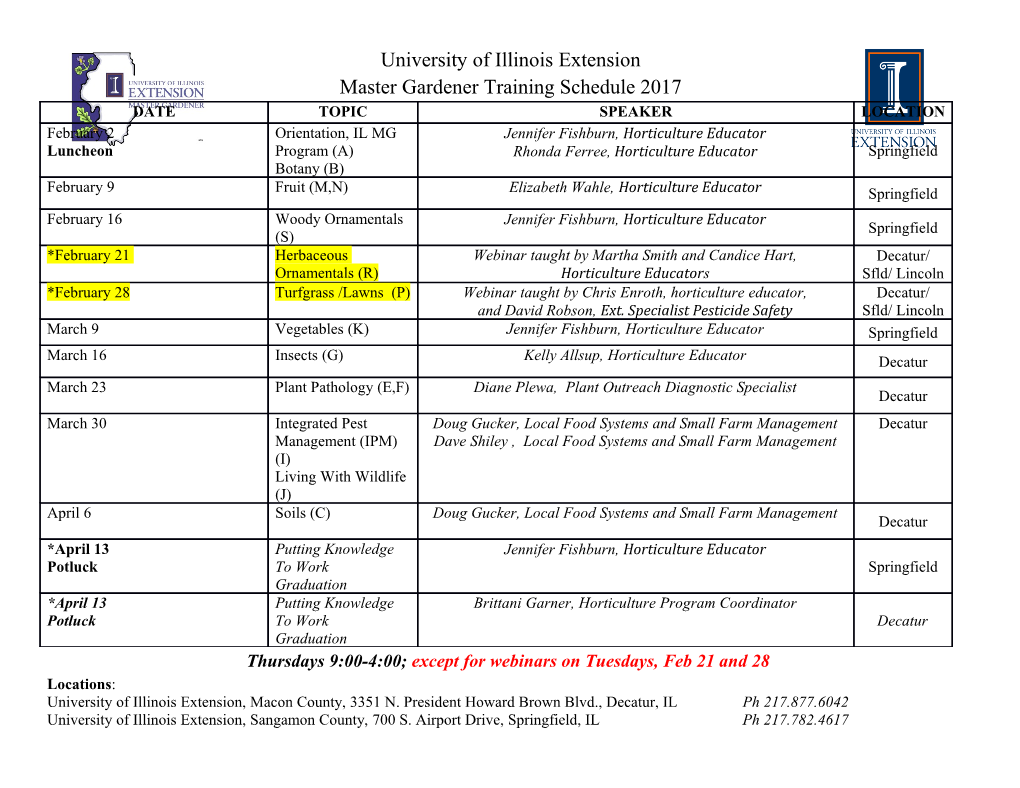
Causal Inference: What If Miguel A. Hernán, James M. Robins October 23, 2019 ii Causal Inference Contents Introduction: Towards less casual causal inferences vii I Causal inference without models 1 1Adefinition of causal effect 3 1.1 Individual causal effects....................... 3 1.2 Average causal effects........................ 4 1.3 Measures of causal effect....................... 7 1.4 Random variability .......................... 8 1.5Causationversusassociation.................... 10 2 Randomized experiments 13 2.1Randomization............................ 13 2.2Conditionalrandomization..................... 17 2.3Standardization............................ 19 2.4 Inverse probability weighting .................... 20 3 Observational studies 25 3.1 Identifiability conditions . ..................... 25 3.2 Exchangeability ............................ 27 3.3Positivity............................... 30 3.4 Consistency: First, definethecounterfactualoutcome...... 31 3.5 Consistency: Second, link counterfactuals to the observed data . 35 3.6Thetargettrial............................ 37 4Effect modification 41 4.1 Definition of effect modification................... 41 4.2 Stratification to identify effect modification............ 43 4.3 Why care about effect modification................. 45 4.4 Stratificationasaformofadjustment............... 47 4.5Matchingasanotherformofadjustment.............. 49 4.6 Effect modificationandadjustmentmethods........... 50 5 Interaction 55 5.1Interactionrequiresajointintervention.............. 55 5.2Identifyinginteraction........................ 56 5.3Counterfactualresponsetypesandinteraction........... 58 5.4 Sufficientcauses........................... 60 5.5 Sufficientcauseinteraction..................... 63 5.6 Counterfactuals or sufficient-componentcauses?.......... 65 iv Causal Inference 6 Graphical representation of causal effects 69 6.1Causaldiagrams........................... 69 6.2Causaldiagramsandmarginalindependence........... 71 6.3Causaldiagramsandconditionalindependence.......... 73 6.4Positivityandconsistencyincausaldiagrams........... 75 6.5 A structural classificationofbias.................. 78 6.6 The structure of effect modification................. 80 7 Confounding 83 7.1Thestructureofconfounding.................... 83 7.2 Confounding and exchangeability .................. 85 7.3 Confounding and the backdoor criterion .............. 87 7.4 Confounding and confounders .................... 90 7.5Single-worldinterventiongraphs.................. 93 7.6 Confounding adjustment . ..................... 94 8 Selection bias 99 8.1Thestructureofselectionbias................... 99 8.2Examplesofselectionbias...................... 101 8.3Selectionbiasandconfounding................... 103 8.4Selectionbiasandcensoring..................... 105 8.5Howtoadjustforselectionbias................... 107 8.6Selectionwithoutbias........................ 110 9 Measurement bias 113 9.1Measurementerror.......................... 113 9.2Thestructureofmeasurementerror................ 114 9.3 Mismeasured confounders . ..................... 116 9.4 Intention-to-treat effect: the effect of a misclassified treatment . 117 9.5 Per-protocol effect.......................... 119 10 Random variability 123 10.1 Identificationversusestimation.................. 123 10.2 Estimation of causal effects.................... 126 10.3Themythofthesuper-population................. 128 10.4Theconditionality“principle”................... 129 10.5Thecurseofdimensionality.................... 133 II Causal inference with models 137 11 Why model? 139 11.1Datacannotspeakforthemselves................. 139 11.2Parametricestimatorsoftheconditionalmean.......... 141 11.3Nonparametricestimatorsoftheconditionalmean....... 142 11.4Smoothing.............................. 143 11.5 The bias-variance trade-off ..................... 145 12 IP weighting and marginal structural models 149 12.1Thecausalquestion......................... 149 12.2EstimatingIPweightsviamodeling................ 150 12.3StabilizedIPweights........................ 153 12.4Marginalstructuralmodels..................... 155 12.5 Effect modificationandmarginalstructuralmodels....... 157 CONTENTS v 12.6Censoringandmissingdata.................... 158 13 Standardization and the parametric g-formula 161 13.1StandardizationasanalternativetoIPweighting........ 161 13.2Estimatingthemeanoutcomeviamodeling........... 163 13.3 Standardizing the mean outcome to the confounder distribution 164 13.4IPweightingorstandardization?................. 165 13.5Howseriouslydowetakeourestimates?............. 167 14 G-estimation of structural nested models 171 14.1Thecausalquestionrevisited................... 171 14.2 Exchangeability revisited . ..................... 172 14.3Structuralnestedmeanmodels.................. 173 14.4Rankpreservation.......................... 175 14.5G-estimation............................. 177 14.6Structuralnestedmodelswithtwoormoreparameters..... 179 15 Outcome regression and propensity scores 183 15.1Outcomeregression......................... 183 15.2Propensityscores.......................... 185 15.3 Propensity stratificationandstandardization........... 186 15.4Propensitymatching........................ 188 15.5Propensitymodels,structuralmodels,predictivemodels.... 189 16 Instrumental variable estimation 193 16.1Thethreeinstrumentalconditions................. 193 16.2TheusualIVestimand....................... 196 16.3Afourthidentifyingcondition:homogeneity........... 198 16.4Analternativefourthcondition:monotonicity.......... 200 16.5Thethreeinstrumentalconditionsrevisited........... 204 16.6Instrumentalvariableestimationversusothermethods..... 206 17 Causal survival analysis 209 17.1Hazardsandrisks.......................... 209 17.2Fromhazardstorisks........................ 211 17.3Whycensoringmatters....................... 214 17.4IPweightingofmarginalstructuralmodels............ 216 17.5Theparametricg-formula..................... 217 17.6G-estimationofstructuralnestedmodels............. 219 18 Variable selection for causal inference 223 18.1 The different goals of variable selection .............. 223 18.2Variablesthatinduceoramplifybias............... 225 18.3Causalinferenceandmachinelearning.............. 228 18.4Doublyrobustmachinelearningestimators............ 229 18.5 Variable selection is a difficultproblem.............. 230 III Causal inference from complex longitudinal data 233 19 Time-varying treatments 235 19.1 The causal effectoftime-varyingtreatments........... 235 19.2Treatmentstrategies........................ 236 19.3Sequentiallyrandomizedexperiments............... 237 vi Causal Inference 19.4Sequentialexchangeability..................... 240 19.5 Identifiability under some but not all treatment strategies . 241 19.6 Time-varying confounding and time-varying confounders .... 245 20 Treatment-confounder feedback 247 20.1Theelementsoftreatment-confounderfeedback......... 247 20.2Thebiasoftraditionalmethods.................. 249 20.3Whytraditionalmethodsfail................... 251 20.4 Why traditional methods cannot be fixed............. 253 20.5Adjustingforpasttreatment.................... 254 21 G-methods for time-varying treatments 257 21.1Theg-formulafortime-varyingtreatments............ 257 21.2IPweightingfortime-varyingtreatments............. 260 21.3Adoublyrobustestimatorfortime-varyingtreatments..... 265 21.4G-estimationfortime-varyingtreatments............. 267 21.5Censoringisatime-varyingtreatment.............. 273 22 Target trial emulation 277 22.1Thetargettrial(revisited)..................... 277 22.2 Causal effectsinrandomizedtrials................ 278 22.3 Causal effects in observational analyses that emulate a target trial281 22.4Timezero.............................. 283 22.5 A unifiedanalysisforcausalinference............... 284 References 288 INTRODUCTION: TOWARDS LESS CASUAL CAUSAL INFERENCES Causal Inference is an admittedly pretentious title for a book. Causal inference is a complex scientific task that relies on triangulating evidence from multiple sources and on the application of a variety of methodological approaches. No book can possibly provide a comprehensive description of methodologies for causal inference across the sciences. The authors of any Causal Inference book will have to choose which aspects of causal inference methodology they want to emphasize. The title of this introduction reflects our own choices: a book that helps scientists–especially health and social scientists–generate and analyze data to make causal inferences that are explicit about both the causal question and the assumptions underlying the data analysis. Unfortunately, the scientific literature is plagued by studies in which the causal question is not explicitly stated and the investigators’ unverifiable assumptions are not declared. This casual attitude towards causal inference has led to a great deal of confusion. For example, it is not uncommon to find studies in which the effect estimates are hard to interpret because the data analysis methods cannot appropriately answer the causal question (were it explicitly stated) under the investigators’ assumptions (were they declared). In this book, we stress the need to take the causal question seriously enough to articulate it, and to delineate the separate roles of data and assumptions for causal inference. Once these foundations are in place, causal inferences become necessarily less casual, which helps prevent confusion. The book describes various data analysis approaches that can be used to estimate the causal effect of interest under a particular
Details
-
File Typepdf
-
Upload Time-
-
Content LanguagesEnglish
-
Upload UserAnonymous/Not logged-in
-
File Pages310 Page
-
File Size-