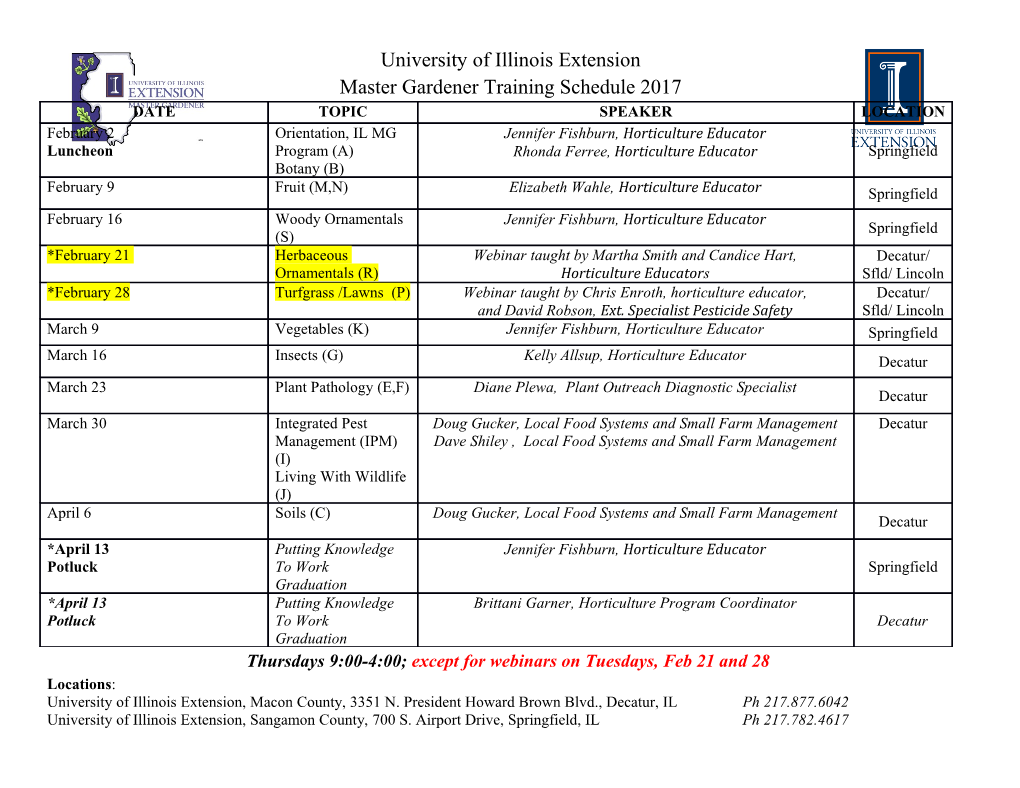
microRNA and smallRNA profiling Anton Enright Group Leader EMBL - European Bioinformatics Institute, Wellcome Trust Genome Campus, Hinxton, Cambridge, CB10 1SD, UK http://www.ebi.ac.uk/enright/ [email protected] High Throughput Sequencing Analysis 2013 Thursday, 24 October 13 microRNA Regulation Adapted from He L. and Hannon G.J; Nature reviews genetics 5:552 (2004) EMBL-EBI 2 High throughput RNA Seq Thursday, 24 October 13 microRNA Regulation Adapted from He L. and Hannon G.J; Nature reviews genetics 5:552 (2004) EMBL-EBI 2 High throughput RNA Seq Thursday, 24 October 13 MicroRNA Processing EMBL-EBI 3 High throughput RNA Seq Thursday, 24 October 13 MicroRNA Processing EMBL-EBI 3 High throughput RNA Seq Thursday, 24 October 13 MicroRNA Processing EMBL-EBI 3 High throughput RNA Seq Thursday, 24 October 13 microRNA Profiling EMBL-EBI 4 High throughput RNA Seq Thursday, 24 October 13 microRNA Profiling • MicroRNA microarray • Statistical issues with number of probes • Cross-hybridisation and biases • Cheap and straightforward • Small RNA Sequencing • Quantitation can be difficult due to biases • Ability to detect novel microRNAs, edits and variation • Reproducible • qRT-PCR Based Approaches • quite accurate • lower throughput • Single Molecule profiling • e.g. Nanostring • New technology EMBL-EBI 5 High throughput RNA Seq Thursday, 24 October 13 Nanostring EMBL-EBI 6 High throughput RNA Seq Thursday, 24 October 13 Nanostring EMBL-EBI 6 High throughput RNA Seq Thursday, 24 October 13 microRNA microarrays • MicroRNA array manufacturers love to talk about how many probes they have on their chips. • In reality there are < 1000 microRNAs for your species and likely <30 expressed in your sample. • A small number of biologically relevant probes = poor reproducibility and background modelling • miRPlus, viral microRNAs, snoRNAs are fairly useless. • In practice significant changes can be observed, but need to validate EMBL-EBI 7 High throughput RNA Seq Thursday, 24 October 13 microRNA arrays EMBL-EBI 8 High throughput RNA Seq Thursday, 24 October 13 microRNA arrays EMBL-EBI 9 High throughput RNA Seq Thursday, 24 October 13 microRNA arrays EMBL-EBI 10 High throughput RNA Seq Thursday, 24 October 13 microRNA Sequencing EMBL-EBI 11 High throughput RNA Seq Thursday, 24 October 13 smallRNA Seq • Illumina/Solexa • Roche 454 • ABI Solid • Ion Torrent • Issues • Amplification biases • Poor quantitation • Mapping Ambiguities • Read Lengths • Size selection • Adaptor Contamination • Read Errors EMBL-EBI 12 High throughput RNA Seq Thursday, 24 October 13 Solexa TruSeq smallRNA prep EMBL-EBI 13 High throughput RNA Seq Thursday, 24 October 13 smallRNA Seq • For mRNA sequencing each molecule is sampled across its length by 30-150nt reads • Biases tend to average out across the length of the transcript • For small RNA sequencing molecules such as microRNAs are shorter than a typical read • No way to average out biases EMBL-EBI 14 High throughput RNA Seq Thursday, 24 October 13 microRNA Sequencing • Biases • GC Bias • Barcode ligation bias • Adapter ligation bias • PCR Amplification bias EMBL-EBI 15 High throughput RNA Seq Thursday, 24 October 13 Downloaded from rnajournal.cshlp.org on December 6, 2012 - Published by Cold Spring Harbor Laboratory Press RNA-ligase-dependent biases microRNA Sequencing • Biases • GC Bias • Barcode ligation bias • Adapter ligation bias • PCR Amplification bias FIGURE 3. miRNA representation by sequencing varies by three orders of magnitude and is dependent on the structure of the mature miRNA and miRNA-adapter product. (A) Unsupervised hierarchical clustering of miRNA profiles derived from cDNA libraries generated from the pool of 815 oligoribonucleotides present in equimolar concentrations (pool A, Supplemental Table 1) using Rnl1, Rnl2(1–249), and Rnl2(1– 249)K227Q for the 39-adapter ligation step and sequenced by Solexa next-generation sequencing platform. (B) Pairwise comparison of Spearman rank correlation coefficients of the miRNA profiles from A.(C) Distribution of average sequence read frequencies of the 770 miRNAs present in equimolar concentrations in pool A in cDNA libraries generated using Rnl1, Rnl2(1–249), and Rnl2(1–249)K227Q in the 39-adapter ligation step. The number of biological replicates for each distribution is indicated. miRNA relative frequencies vary by 1000-fold. least efficiently sequenced miRNAs)EMBL-EBI and 64% for miR-567 with equimolar amounts of adapter-ligated material, using 15 High throughput RNA Seq (the most frequently sequenced miRNA). This efficiency a 25-fold excess of radioactively labeled reverse transcrip- Thursday, 24 October 13 range explained well the observed broad sequence read tion primer followed by hydrolysis of the RNA template. frequency distribution as well as the rank of miRNAs. The yields of primer extension products were comparable Furthermore, the up to threefold over-representation of some (data not shown), indicating that reverse transcription was miRNAs relative to the mean read frequency is consistent with not a significant source of sequence-specific biases. the 21% cumulative ligation yield of pool A RNA (Table 2). Lastly, we examined the influence of excessive PCR on We further isolated the products from the 59-adapter small RNA read frequency distribution. A small RNA cDNA ligation for pool A, miR-567, miR-155, miR-10a, miR-16, library generated from pool A using Rnl2(1–249)K227Q for and miR-21 and performed reverse transcription reactions the 39-ligation step followed by Solexa sequencing. This www.rnajournal.org 7 Absolute quantitation can be difficult but differential expression seems more robust EMBL-EBI 16 High throughput RNA Seq Thursday, 24 October 13 Downloaded from pixfunlobdot.59.to on June 23, 2010 - Published by Cold Spring Harbor Laboratory Press Marioni et al. same concentration, only a small proportion of genes show evi- 2004) to the array data, we identified 8113 genes as differentially dence for differences among lanes over those expected from sam- expressed at an FDR of 0.1% (83% with an estimated absolute pling error. For sequences sampled at different concentrations, log2-fold change > 0.5, 43% > 1). Of these, 81% of genes were the differences were more appreciable. Thus, for the remainder of also identified as differentially expressed from the Illumina se- this paper, we consider only the data sequenced at a concentra- quencing data, providing strong evidence that the majority of tion of 3 pM (five lanes for each sample). genes called from the sequence data are genuinely differentially expressed between the two samples. Furthermore, estimates of Identifying differentially expressed genes the log2-fold changes of gene expression levels between the samples across the two technologies are correlated (Spearman The Poisson model described above provides a natural framework correlation = 0.73) (Fig. 4). The correlation is greater for genes for identifying differentially expressed genes. Indeed, the model that are mapped to by large numbers of sequence reads. For ex- can be cast as a generalized linear model (McCullagh and Nelder ample, for genes mapped to by (on average) more than 32 reads 1989), and standard methods exist to estimate parameters, and to in both tissues (Ն5 on the log scale in Fig. 3), the Spearman compute P-values for each gene testing the null hypothesis that correlation of the fold changes across technologies is 0.79 com- it is not differentially expressed between two groups (see Meth- pared with 0.60 for genes mapped to by at least one but fewer than ods). 32 reads. These comparisons with the array data demonstrate that The results from the goodness-of-fit test above suggest that the Illumina sequencing technology and our analysis approach are a small proportion of genes show deviations from the Poisson performing well. A complete comparison of genewise results from assumption (extra-Poisson variation). To check whether this as- both technologies is available in Supplemental Table 3. pect of the data will lead to false-positive identifications of dif- Considered together, 6538 genes were identified as differen- ferentially expressed genes, we applied the Poisson model to tially expressed using either the sequencing or the array data but identify differentially expressed genes between groups of lanes not by both (Fig. 5). To further examine these discrepancies, we used to sequence the same sample. We observed that even for the used a third technology, quantitative PCR (qPCR), to test for pair of lanes that displayed the strongest evidence of a lane effect, differences in expression between the liver and kidney samples only 14 genes were identified as differentially expressed at a false for five genes called differentially expressed from the sequence discovery rate (FDR) of 0.1% (Supplemental Fig. 7). Similarly, data but not the array (MMP25, SLC5A1, MDK, ZNF570, GPR64) when we applied this model to groups that each contained two and for six genes that were found to be differentially expressed lanes used to sequence the same sample, the worst comparison using the array, but not the sequencing data (C16orf68, CD38, yielded only 24 genes that were incorrectly identified as differ- LSM7, S100P, PEX11A, GLOD5). We designed primers for the entially expressed. We conclude that, in this context, at this qPCR within 1 kb upstream of the annotated 3Ј-end of the genes stringent FDR, deviations from the Poisson model do not lead to (Methods). The qPCR results confirmed as differentially ex- the identification of an appreciable number of false-positive
Details
-
File Typepdf
-
Upload Time-
-
Content LanguagesEnglish
-
Upload UserAnonymous/Not logged-in
-
File Pages97 Page
-
File Size-