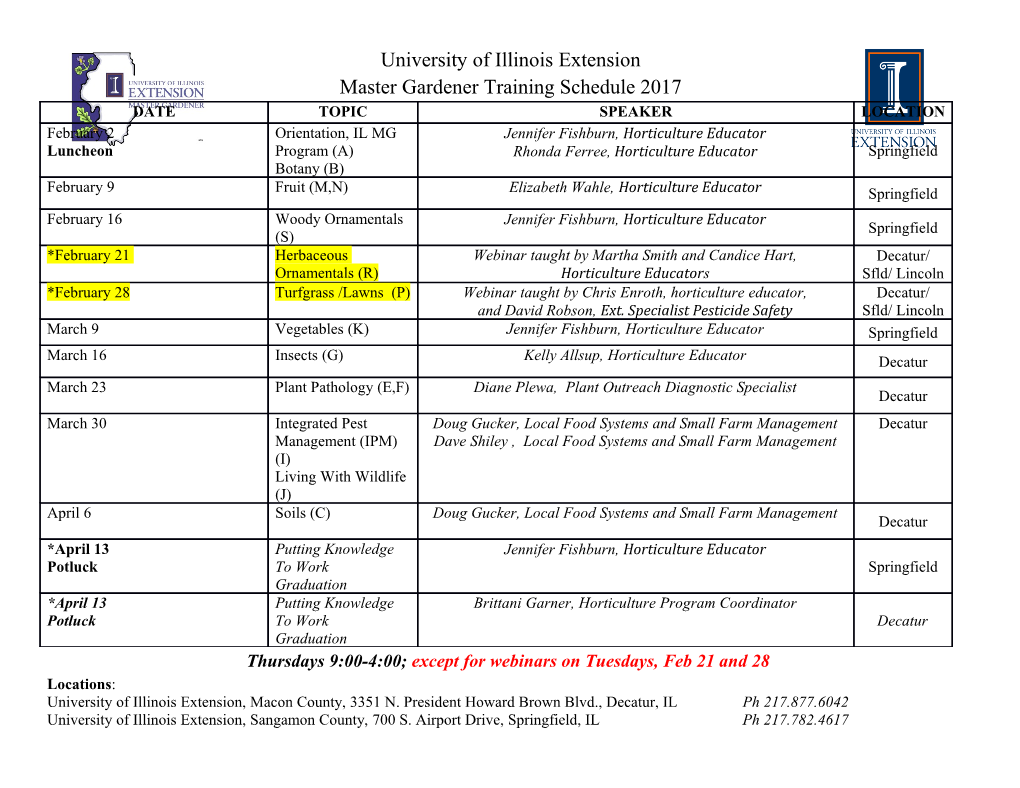
Summary Papers- Applied Multivariate Statistical Modeling- Sampling Distribution -Chapter Three Author: Mohammed R. Dahman. Ph.D. in MIS ED.10.18 License: CC-By Attribution 4.0 International Citation: Dahman, M. R. (2018, October 22). AMSM- Sampling Distribution- Chapter Three. https://doi.org/10.31219/osf.io/h5auc 1. Preface Introduction of differences between population parameters and sample statistics are discussed. Followed with a comprehensive analysis of sampling distribution (i.e. definition, and properties). Then, we have discussed essential examiners (i.e. distribution): Z distribution, Chi square distribution, t distribution and F distribution. At the end, we have introduced the central limit theorem and the common sampling strategies. Please check the dataset from (Dahman, 2018a), chapter one (Dahman, 2018b), and chapter two (Dahman, 2018c). 2. Introduction As a starting point I have to draw your attention to the importance of this unit. The bad news is that I have to use some rigid mathematic and harsh definitions. However, the good news is that I assure you by the end of this chapter you will be able to understand every word, in addition to all the math formulas. From chapter two (Dahman, 2018a), we have understood the essential definitions of population, sample, and distribution. Now I feel very much comfortable to share this example with you. Let’s say I have a population (A). and I’m able to draw from this population any sample (s). Facts are: 1. The size of (A) is (N); the mean is (µ); the variance is (ퟐ); the standard deviation is (); the proportion (횸); correlation coefficient (). 2. The size of (s) is (n); the mean is (풙̅); the variance is (퓼ퟐ); the standard deviation is (풔); the proportion (횸̂); correlation coefficient (풓). Please keep in mind this, (µ, ퟐ, , 횸, ) are the “population parameters”. On the other hand, (풙̅, 퓼ퟐ, 풔, 횸̂, 풓) are the “sample statistics”. Hereafter, any time, in this summary papers, I mention “parameters” that means any values from population. Otherwise, “statistics” that means values from the sample. By the way, I have got a question! What exactly “statistics” are? a measure which describes a fraction of population or estimation of population parameters. In other words, take for example (풙̅) is an estimation of (µ). And (퓼ퟐ) is an estimation of (ퟐ) and so on. 3. Sampling Distribution Let’s assume that I have drawn “k” number of samples from a population “A”. It’s obvious that every sample will give me “statistics” (i.e. 풙̅, 퓼ퟐ, etc.). let’s say that I have listed the output in a dataset as follow: ퟐ From this table you should train yourself how multivariate dimension looks 푠푎푚푝푙 1 풙̅ퟏ 퓼 ퟏ . ퟐ like. Although, we are still in the univariate level. I just wanted you to be 푠푎푚푝푙푒 2 풙̅ퟐ 퓼 ퟐ . ퟐ familiar with such a picture. But now let’s take only one sample from this 푠푎푚푝푙푒 푖 풙̅풊 퓼 풊 . ퟐ dataset. Let’s take “풔풂풎풑풍풆 풊”. See this sample has its list of statistics. 푠푎푚푝푙푒 푘 풙̅풌 퓼 풌 . ퟐ It has the estimation of mean “풙̅풊”, the estimation of variance “퓼 풊” and so on. If I take another sample let’s ퟐ say “풔풂풎풑풍풆 풌”. Of course, it has its own estimation of mean “풙̅풌”, the estimation of variance “퓼 풌” and P a g e 1 | 12 Summary Papers- Applied Multivariate Statistical Modeling- Sampling Distribution -Chapter Three Author: Mohammed R. Dahman. Ph.D. in MIS ED.10.18 License: CC-By Attribution 4.0 International Citation: Dahman, M. R. (2018, October 22). AMSM- Sampling Distribution- Chapter Three. https://doi.org/10.31219/osf.io/h5auc so on. The question now, are the values of 풙̅풊 and 풙̅풌 identical? The answer nobody knows! That’s why we consider “statistics” values are actually “random variables”. Because there is no grantee that if you select a sample “1” and measure its “statistics” will be identical to the same “statistics” from other samples of the same population. Having we established this fact, that lead us to another. Let’s say that I’m interested to find out the distribution of “풙̅”. You know now from chapter two that I’m able to find density function, the central tendency, the dispersion, and the shape. See the table where we have: a vector of all the measured “풙̅” from “풔풂풎풑풍풆 ퟏ” till “풔풂풎풑풍풆 풌”. The question 푠푎푚푝푙푒 1 풙ퟏ 푠푎푚푝푙푒 2 풙 is what is the distribution of this vector? The answer is the title of this chapter ퟐ . “sampling distribution”. From what we have learned from chapter two, by now we 푠푎푚푝푙푒 푘 풙 are able to follow the same steps; by finding the distribution of this 풌 list of “random variables”. In other words, “the density function, the central tendency, dispersion, and the shape”. Doing so I will be able to understand the behavior or in other words the distribution of these values (i.e. estimated values of parameters). 4. Examiners I would like to draw your attention to the importance of this section. We have discussed in chapter 2 (Dahman, 2018a), various types of distribution based on the categories (i.e. discrete or continues). If you have missed that part I recommend to you checking that out. Following we are going to discuss whatso called essential distributions. These distributions are very important for you in order to understand the concept of research and data analysis. The name distribution is not the same concept as you have learned from chapter two. Most of the books considering naming them as “distributions”, however I prefer to call them the “examiners”. Kindly, follow the summary papers and you will be able to understand my reason of giving that name. • Z distribution now it’s very clear to you that is one of the distributions of which we have learned, and widely used, is the normal distribution. Normal distribution is a continuous probability distribution. It is also called Gaussian distribution. The normal distribution density function f(z) is called the Bell Curve because it has 푥−휇 2 1 −( ) the shape that resembles a bell. The probability density function is given by: 푓(푥) = 푒 2휎2 . 푥 is 휎√2휋 the random variable; μ is the mean value; σ is the standard deviation (std) value; e = 2.7182818... constant; π = 3.1415926... constant. How to use this formula! Let’s assume that I have two datasets from two different subjects as follow: Eng. DS:: 86.00, 84.00, 86.00, 85.00, 83.00, 81.00, 81.00, 80.00, 90.00, 81.00, 80.00, 90.00, 79.00 MSA DS:: 49.00, 52.00, 59.00, 42.00, 65.00, 49.00, 57.00, 52.00, 48.00, 52.00, 53.00, 50.00, 66.00 The descriptive statistics as well as the “pdf” as following: P a g e 2 | 12 Summary Papers- Applied Multivariate Statistical Modeling- Sampling Distribution -Chapter Three Author: Mohammed R. Dahman. Ph.D. in MIS ED.10.18 License: CC-By Attribution 4.0 International Citation: Dahman, M. R. (2018, October 22). AMSM- Sampling Distribution- Chapter Three. https://doi.org/10.31219/osf.io/h5auc It’s known that, in Eng. Dataset the largest score is “90”. On the other hand, in MSA dataset the largest score is “66”. Now, if we are asked to ANSWER TWO QUESTIONS: 1. From Eng. Class: if I pick a random test without looking at the score and you want to know the probability the score was below “80”? What you can do is take the probability density function; and calculate the probability P(X<80) integrating on this function from -∞ to 80, replacing your SD and mean on the function. But it is a tedious integration at best. 2. tell who performed better with respect to others, the student with score “90” in Eng. Class or student with score “66” in MSA class? Looking from the cover, it’s easy to say of course the student who scored “90”. However, you have dismissed a very important measurement which is the variance. Here is the point where the “Z distribution” comes into the picture. What is it? It is a probability density function and especially a normal distribution that has a mean equal to zero and a standard deviation equal to one and that is used especially in testing hypotheses about means or proportions of samples drawn from populations whose population standard deviations are known. 풙−흁 Let’s create a standardized value 풛 = . And you have to know that the expected value of “z” is zero; 푬(풛) = 흁 = ퟎ and the expected variance of “z” is 1; 푽풂풓(풛) = ퟐ = ퟏ. Note: to keep things easy I didn’t want to go over the prove of this. If you are interested to find more about the mathematical proof you can see (Dean W. & Wichern, 2007). Following that I’m able to create a standardized “pdf”, however you will see that the mean center is “0” and standard deviation is “1”. Let’s go back and answer the first and the second question using “Z distribution”: 1. P(X<80) = P(X< (80−85.53)/3.68) = P(X< -0.951). To get this value you can integrate on the SND density function or (much preferably) you can search on the normal distribution table (or z-table) which resumes the results of many possible evaluations, so you don’t have to integrate. In this case, P a g e 3 | 12 Summary Papers- Applied Multivariate Statistical Modeling- Sampling Distribution -Chapter Three Author: Mohammed R.
Details
-
File Typepdf
-
Upload Time-
-
Content LanguagesEnglish
-
Upload UserAnonymous/Not logged-in
-
File Pages12 Page
-
File Size-