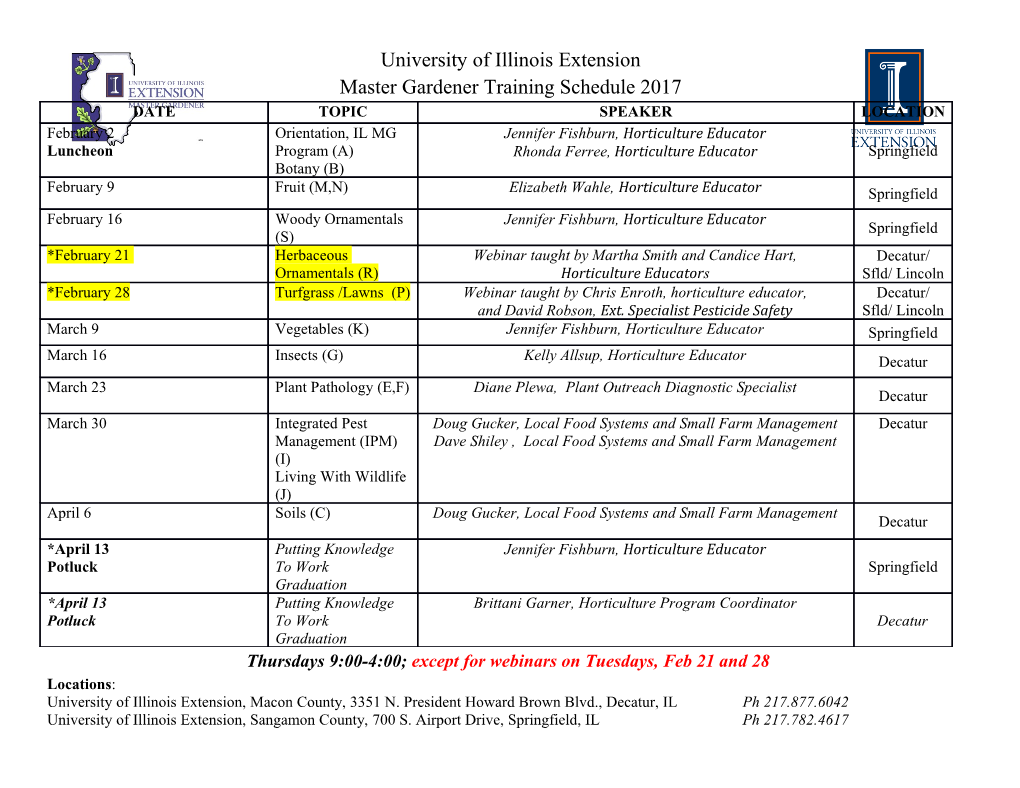
Foundations of Data Science∗ Avrim Blum, John Hopcroft and Ravindran Kannan Thursday 9th June, 2016 ∗Copyright 2015. All rights reserved 1 Contents 1 Introduction 8 2 High-Dimensional Space 11 2.1 Introduction . 11 2.2 The Law of Large Numbers . 11 2.3 The Geometry of High Dimensions . 14 2.4 Properties of the Unit Ball . 15 2.4.1 Volume of the Unit Ball . 15 2.4.2 Most of the Volume is Near the Equator . 17 2.5 Generating Points Uniformly at Random from a Ball . 20 2.6 Gaussians in High Dimension . 21 2.7 Random Projection and Johnson-Lindenstrauss Lemma . 23 2.8 Separating Gaussians . 25 2.9 Fitting a Single Spherical Gaussian to Data . 27 2.10 Bibliographic Notes . 29 2.11 Exercises . 30 3 Best-Fit Subspaces and Singular Value Decomposition (SVD) 38 3.1 Introduction and Overview . 38 3.2 Preliminaries . 39 3.3 Singular Vectors . 41 3.4 Singular Value Decomposition (SVD) . 44 3.5 Best Rank-k Approximations . 45 3.6 Left Singular Vectors . 47 3.7 Power Method for Computing the Singular Value Decomposition . 49 3.7.1 A Faster Method . 50 3.8 Singular Vectors and Eigenvectors . 52 3.9 Applications of Singular Value Decomposition . 52 3.9.1 Centering Data . 52 3.9.2 Principal Component Analysis . 53 3.9.3 Clustering a Mixture of Spherical Gaussians . 54 3.9.4 Ranking Documents and Web Pages . 59 3.9.5 An Application of SVD to a Discrete Optimization Problem . 60 3.10 Bibliographic Notes . 63 3.11 Exercises . 64 4 Random Graphs 71 4.1 The G(n; p)Model ............................... 71 4.1.1 Degree Distribution . 72 4.1.2 Existence of Triangles in G(n; d=n).................. 77 4.2 Phase Transitions . 79 4.3 The Giant Component . 87 2 4.4 Branching Processes . 96 4.5 Cycles and Full Connectivity . 102 4.5.1 Emergence of Cycles . 102 4.5.2 Full Connectivity . 104 4.5.3 Threshold for O(ln n) Diameter . 105 4.6 Phase Transitions for Increasing Properties . 107 4.7 Phase Transitions for CNF-sat . 109 4.8 Nonuniform and Growth Models of Random Graphs . 114 4.8.1 Nonuniform Models . 114 4.8.2 Giant Component in Random Graphs with Given Degree Distribution114 4.9 Growth Models . 116 4.9.1 Growth Model Without Preferential Attachment . 116 4.9.2 Growth Model With Preferential Attachment . 122 4.10 Small World Graphs . 124 4.11 Bibliographic Notes . 129 4.12 Exercises . 130 5 Random Walks and Markov Chains 139 5.1 Stationary Distribution . 143 5.2 Markov Chain Monte Carlo . 145 5.2.1 Metropolis-Hasting Algorithm . 146 5.2.2 Gibbs Sampling . 147 5.3 Areas and Volumes . 150 5.4 Convergence of Random Walks on Undirected Graphs . 151 5.4.1 Using Normalized Conductance to Prove Convergence . 157 5.5 Electrical Networks and Random Walks . 160 5.6 Random Walks on Undirected Graphs with Unit Edge Weights . 164 5.7 Random Walks in Euclidean Space . 171 5.8 The Web as a Markov Chain . 175 5.9 Bibliographic Notes . 179 5.10 Exercises . 180 6 Machine Learning 190 6.1 Introduction . 190 6.2 Overfitting and Uniform Convergence . 192 6.3 Illustrative Examples and Occam's Razor . 194 6.3.1 Learning disjunctions . 194 6.3.2 Occam's razor . 195 6.3.3 Application: learning decision trees . 196 6.4 Regularization: penalizing complexity . 197 6.5 Online learning and the Perceptron algorithm . 198 6.5.1 An example: learning disjunctions . 198 6.5.2 The Halving algorithm . 199 3 6.5.3 The Perceptron algorithm . 199 6.5.4 Extensions: inseparable data and hinge-loss . 201 6.6 Kernel functions . 202 6.7 Online to Batch Conversion . 204 6.8 Support-Vector Machines . 205 6.9 VC-Dimension . 206 6.9.1 Definitions and Key Theorems . 207 6.9.2 Examples: VC-Dimension and Growth Function . 209 6.9.3 Proof of Main Theorems . 211 6.9.4 VC-dimension of combinations of concepts . 214 6.9.5 Other measures of complexity . 214 6.10 Strong and Weak Learning - Boosting . 215 6.11 Stochastic Gradient Descent . 218 6.12 Combining (Sleeping) Expert Advice . 220 6.13 Deep learning . 222 6.14 Further Current directions . 228 6.14.1 Semi-supervised learning . 228 6.14.2 Active learning . 231 6.14.3 Multi-task learning . 231 6.15 Bibliographic Notes . 232 6.16 Exercises . 233 7 Algorithms for Massive Data Problems: Streaming, Sketching, and Sampling 237 7.1 Introduction . 237 7.2 Frequency Moments of Data Streams . 238 7.2.1 Number of Distinct Elements in a Data Stream . 239 7.2.2 Counting the Number of Occurrences of a Given Element. 242 7.2.3 Counting Frequent Elements . 243 7.2.4 The Second Moment . 244 7.3 Matrix Algorithms using Sampling . 247 7.3.1 Matrix Multiplication Using Sampling . 249 7.3.2 Implementing Length Squared Sampling in two passes . 252 7.3.3 Sketch of a Large Matrix . 253 7.4 Sketches of Documents . 256 7.5 Bibliography . 258 7.6 Exercises . 259 8 Clustering 264 8.1 Introduction . 264 8.1.1 Two general assumptions on the form of clusters . 265 8.2 k-means Clustering . 267 8.2.1 A maximum-likelihood motivation for k-means . 267 4 8.2.2 Structural properties of the k-means objective . 268 8.2.3 Lloyd's k-means clustering algorithm . 268 8.2.4 Ward's algorithm . 270 8.2.5 k-means clustering on the line . 271 8.3 k-Center Clustering . 271 8.4 Finding Low-Error Clusterings . 272 8.5 Approximation Stability . 272 8.6 Spectral Clustering . 275 8.6.1 Stochastic Block Model . 276 8.6.2 Gaussian Mixture Model . ..
Details
-
File Typepdf
-
Upload Time-
-
Content LanguagesEnglish
-
Upload UserAnonymous/Not logged-in
-
File Pages439 Page
-
File Size-