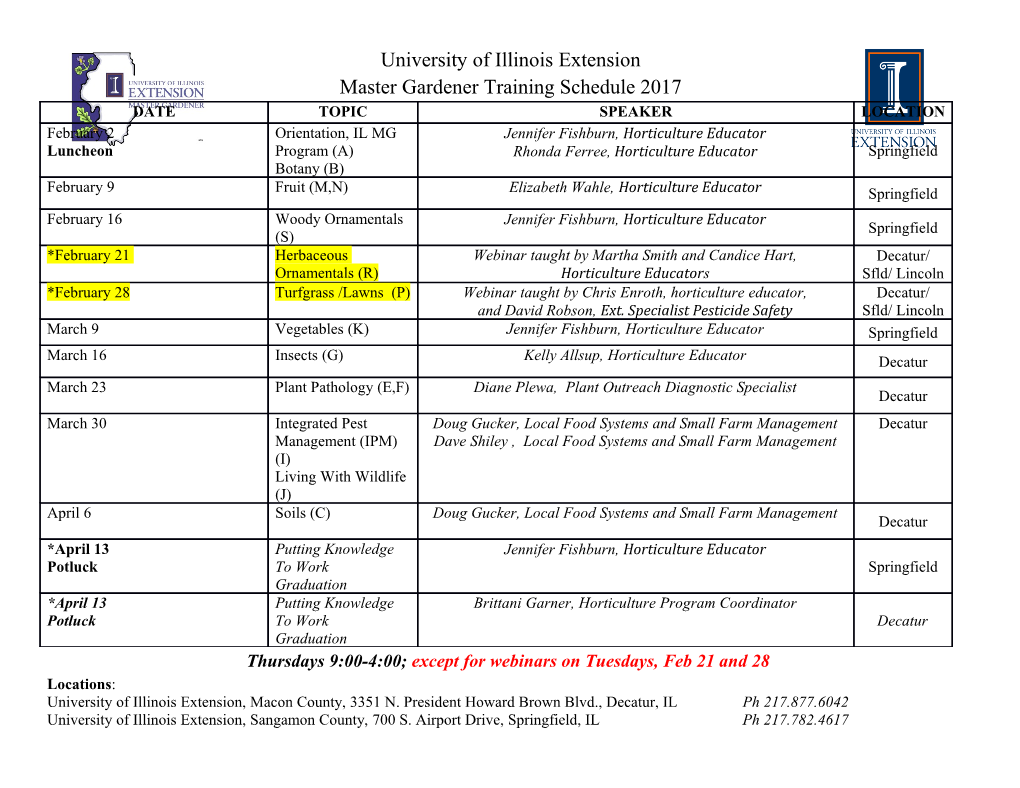
UNIVERSITE´ DE MONTREAL´ DEMAND FORECASTING IN REVENUE MANAGEMENT SYSTEMS SHADI SHARIF AZADEH DEPARTEMENT´ DE MATHEMATIQUES´ ET DE GENIE´ INDUSTREL ECOLE´ POLYTECHNIQUE DE MONTREAL´ THESE` PRESENT´ EE´ EN VUE DE L'OBTENTION DU DIPLOME^ DE PHILOSOPHIÆ DOCTOR (MATHEMATIQUES´ DE L'INGENIEUR)´ AOUT^ 2013 c Shadi Sharif Azadeh, 2013. UNIVERSITE´ DE MONTREAL´ ECOLE´ POLYTECHNIQUE DE MONTREAL´ Cette th`eseintitul´ee: DEMAND FORECASTING IN REVENUE MANAGEMENT SYSTEMS pr´esent´ee par : SHARIF AZADEH, Shadi en vue de l'obtention du dipl^ome de : Philosophiæ Doctor a ´et´ed^ument accept´eepar le jury d'examen constitu´ede : M. ADJENGUE Luc, Ph.D., pr´esident M. SAVARD Gilles, Ph.D., membre et directeur de recherche M. MARCOTTE Patrice, Ph.D., membre et codirecteur de recherche M. LABIB Richard, Ph.D., membre et codirecteur de recherche M. BASTIN Fabian, Doct., membre Mme CIRILLO Cinzia, Ph.D., membre iii To My Heroes, My Lovely Parents, My Dear Saeed , To The Love of My Life, Yousef And To My Beloved Zari Who Always Believed in Me. iv ACKNOWLEDGEMENT I wish to express my sincere appreciation to many individuals and organizations who made this research possible. Special acknowledgment is made to Professor Gilles Savard for directing the thesis with his well understanding, encouragement and guidance during the research. Grateful acknowledgment is made to Professor Patrice Marcotte and Richard Labib for providing invaluable suggestions during my studies. I would like to thank my industrial advisors, Dr. Anne Mercier, Dr. Louis-Philippe Bigras and Dr. Gerardo Berbeglia for their support and guidance. I would like to express my deepest gratitude to my second family at GERAD who made this journey as enjoyable as possible. Special thanks to my dear colleagues and friends : Morad Hosseinalifam, Dr. Ahad Dehghani, Sanaz bararpour, Samin Sadrebazzaz. Finally, I wish to extend my deepest appreciation and gratitude first and foremost to my parents who have shown me how to be passionate and motivated for getting better every day by example, to my best friend, the warmth and the love of my life, Yousef, to my adorable baby brother, Saeed and kind sister, Maryam, and my lovely baba Reza and maman Fariba not only for their encouragement, but also for their help and support in making this research possible. This work has been supported by Natural Sciences and Engineering Research Council of Canada (NSERC)-Fonds Qu´eb´ecois de la Recherche sur la Nature et les Technologies (FQRNT) and ExPretio company in Montreal. v RESUM´ E´ La gestion des revenues est l'art de d´evelopper des mod`eles math´ematiques capables de d´eterminer quel produit offrir `aquel segment de consommateurs `aun moment pr´ecis dans le but de maximiser les profits. La pr´evision de la demande joue un r^ole fondamental dans la gestion des revenues, car un manque de pr´ecision `acet ´egard engendrer une perte de profits. Dans cette th`ese, nous proposons une ´etude syst´ematique et approfondie de diff´erentes m´ethodes qui sont employ´ees pour pr´evoir la demande. Tout d'abord, nous pr´esenterons un nouveau sch´ema de classification d´etaillant les caract´eristiques de ces diff´erentes m´ethodes pour d´eterminer en quoi elles diff`erent les unes des autres. Dans ce but, nous ferons une ana- lyse exhaustive de la litt´erature existant `ace sujet pour ^etre `am^eme de bien cat´egoriser ces m´ethodes dans notre sch´ema. Par la suite, nous investiguerons `apropos des syst`emes de ges- tion des revenue qui utilisent un r´eseau neuronal artificiel modifi´ecombin´e`aun historique des donn´ees pour pr´evoir le nombre de passagers, selon les heures de d´epart, pour une impor- tante entreprise europ´eenne de transport ferroviaire. Apr`es,afin de bien cerner les effets de saisonnalit´eet mod´eliser le comportement des consommateurs, nous proposerons un nouveau mod`ele non param´etrique. La source de notre probl´ematique part d'un mod`ele non-convexe et non lin´eaire compos´e de variables enti`eres. Dans ce mod`ele, les variables repr´esentent l'utilit´ede chaque produit ainsi que la demande potentielle de chaque jour et les variables binaires qui sont utilis´eesafin d'assigner chaque jour `achaque groupe des jours selon ses caract´eristiques. Nous avons li- n´earis´eet rendu convexe ce mod`ele avec succ`esen utilisant des techniques de lin´earisation. Puis, nous avons pr´esent´eles caract´eristiques de la disponibilit´epour un temps donn´eafin d'extraire les corr´elations entre les probabilit´esg´en´er´ees par ces choix. De plus, nous avons d´etermin´epour chaque journ´ee un nombre pr´ed´efini de blocs selon les caract´eristiques sp´eci- fiques de la demande. Ainsi, nous avons pu d´eterminer une solution initiale bas´eesur laquelle on serre l'amplitude des variables. Ensuite, nous avons repr´esent´eun algorithme s´eparation et ´evaluation impliquant des techniques d'optimisation globale pour estimer les utilit´es et la de- mande potentielle `achaque jour. Le pr´etraitement des donn´ees a n´ecessit´el'impl´ementation de plusieurs nœuds avant effectuer le branchement. Ce processus utilise des solveurs lin´eaires et non lin´eaires. Les r´esultats sont repr´esent´espar donn´ees synth´etiques et donn´eesr´eelles. Par ailleurs, ces r´esultats sont compar´es `adeux mod`eles non lin´eaires d'optimisation glo- bale bien connus. Le mod`ele que nous proposons offre une performance nettement sup´erieure. Dans la derni`ere partie de cette dissertation, nous ´etudierons l'impact de ce mod`ele de de- mande sur la performance des revenues g´en´er´ees. Les r´esultats sont repr´esent´es`al'aide des vi donn´ees synth´etiques g´en´er´es par une programmation lin´eaire d´eterministe bas´eesur les mo- d`eles de choix discret. Mots cl´es: Syst`eme de gestion des revenues, Mod`ele de choix discret, R´eseau de neurones artificiel, Optimisation globale vii ABSTRACT A revenue management system is defined as the art of developing mathematical models that are capable of determining which product should be offered to which customer segment at a given time in order to maximize revenue. Demand forecasting plays a crucial role in rev- enue management. The lack of precision in demand models results in the loss of revenue. In this thesis, we provide an in-depth and systematic study of different methods that are applied to demand forecasting. We first introduce a new classification scheme for them and propose the characteristics that differentiate the methods from one another. All existing papers are reviewed and many of them have been categorized based on our classification scheme. After, we investigated a demand prediction model that uses a modified neural network method and historical data to forecast the number of passengers at the departure time for a major Euro- pean railway company. Afterwards, in order to capture seasonal effects and taking customer behavior into account, we proposed a new, non-parametric mathematical model. The original problem is a nonconvex nonlinear model with integer variables. The variables in this model are the product utilities, the daily demand flow and binary assignment variables. We success- fully linearized and convexified the model by using linearization techniques. Then, we used the characteristics of product availabilities for a given time to extract logical relations between choice probabilities. Moreover, we have classified each day to one of the predefined numbers of clusters based on their related daily demand flow. We represent a branch and bound al- gorithm, which uses global optimization techniques to find the estimated utilities and daily potential demand. Several node preprocessing techniques are implemented before branching. Both linear and nonlinear solvers are used in the branching process. The computational re- sults are represented by using synthetic data. Also, they are compared to two well-known nonlinear and global optimizers and our proposed model outperforms both solvers. In the final part of this dissertation, we investigate the impact of the suggested demand model on revenue performance. The numerical results are presented using synthetic data produced by a modified Deterministic Choice-Based Linear Programming approach. Keywords: Revenue Management, Choice-Based Demand Modeling, Uncensoring Meth- ods, Neural Networks, Global Optimization Approach viii TABLE OF CONTENTS DEDICATION . iii ACKNOWLEDGEMENT . iv RESUM´ E...........................................´ v ABSTRACT ......................................... vii TABLE OF CONTENTS . viii LIST OF TABLES . xi LIST OF FIGURES . xii INTRODUCTION . 1 CHAPTER 1 ARTICLE 1 : A TAXONOMY OF DEMAND UNCENSORING ME- THOD IN REVENUE MANAGEMENT . 4 1.1 Introduction.....................................4 1.2 Features of demand models in RM systems . .6 1.2.1 Supply-side features . .7 1.2.2 Demand characteristics . .8 1.3 Estimation of unconstrained demand . 10 1.3.1 Basic methods . 11 1.3.2 Statistical methods . 12 1.3.3 Choice-based models . 15 1.3.4 Optimization methods . 16 1.4 Ataxonomy..................................... 20 1.5 Conclusion...................................... 21 CHAPTER 2 ARTICLE 2 : RAILWAY DEMAND FORECASTING IN REVENUE MA- NAGEMENT USING NEURAL NETWORKS . 24 2.1 Introduction..................................... 24 2.2 Problemdefinition ................................. 26 2.3 Pre-processing . 28 ix 2.4 Model ........................................ 30 2.4.1 Architecture of the neural network . 30 2.4.2 Learning algorithm and parameter adjustments . 31 2.4.3 Model improvements . 32 2.4.4 Validation.................................. 33 2.5 Results........................................ 34 2.6 Conclusion...................................... 39 CHAPTER 3 ARTICLE 3 : A NON-PARAMETRIC APPROACH TO DEMAND FO- RECASTING IN REVENUE MANAGEMENT . 44 3.1 Introduction..................................... 44 3.2 Problem formulation . 46 3.3 Algorithmic framework . 49 3.3.1 Linearization . 49 3.3.2 Relaxation and convexification . 51 3.4 Solutionalgorithm ................................. 52 3.4.1 Preprocessing . 52 3.4.2 Branch-and-bound .
Details
-
File Typepdf
-
Upload Time-
-
Content LanguagesEnglish
-
Upload UserAnonymous/Not logged-in
-
File Pages100 Page
-
File Size-