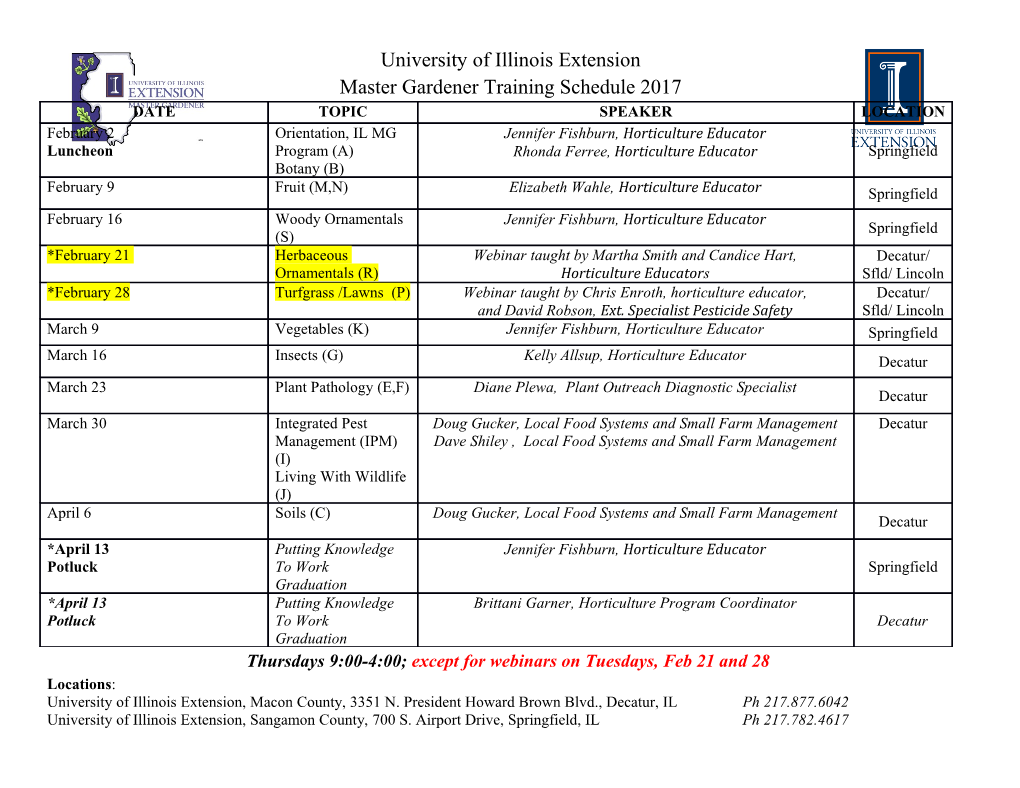
CSE4351/5351 Parallel Processing Instructor: Dr. Song Jiang The CSE Department [email protected] http://ranger.uta.edu/~sjiang/CSE4351-5351-summer/index.htm Lecture: MoTuWeTh 10:30AM - 12:30PM LS 101 Office hours: Monday /2-3pm at ERB 101 1 Outline ▪ Introduction ➢What is parallel computing? ➢Why you should care? ▪ Course administration ➢Course coverage ➢Workload and grading ▪ Inevitability of parallel computing ➢Application demands ➢Technology and architecture trends ➢Economics ▪ Convergence of parallel architecture ➢ Shared address space, message passing, data parallel, data flow ➢ A generic parallel architecture 2 What is Parallel Computer? “A parallel computer is a collection of processing elements that can communicate and cooperate to solve large problems fast” ------ Almasi/Gottlieb ▪ “communicate and cooperate” ➢Node and interconnect architecture ➢Problem partitioning and orchestration ▪ “large problems fast” ➢Programming model ➢Match of model and architecture ▪ Focus of this course ▪ Parallel architecture ▪ Parallel programming models ▪ Interaction between models and architecture 3 What is Parallel Computer? (cont’d) Some broad issues: • Resource Allocation: – how large a collection? – how powerful are the elements? • Data access, Communication and Synchronization – how are data transmitted between processors? – how do the elements cooperate and communicate? – what are the abstractions and primitives for cooperation? • Performance and Scalability – how does it all translate into performance? – how does it scale? 4 Why Study Parallel Computing ▪ Inevitability of parallel computing ➢ Fueled by application demand for performance • Scientific: weather forecasting, pharmaceutical design, and genomics • Commercial: OLTP, search engine, decision support, data mining • Scalable web servers ➢ Enabled by technology and architecture trends • limits to sequential CPU, memory, storage performance o parallelism is an effective way of utilizing growing number of transistors. • low incremental cost of supporting parallelism ▪ Convergence of parallel computer organizations ➢ driven by technology constraints and economies of scale • laptops and supercomputers share the same building block ➢ growing consensus on fundamental principles and design tradeoffs 5 Why Study Parallel Computing (cont’d) • Parallel computing is ubiquitous: ➢ Multithreading ➢ Simultaneous multithreading (SMT) a.k.a. hyper-threading • e.g., Intel® Pentium 4 Xeon ➢Chip Multiprocessor (CMP) a.k.a, multi-core processor • Intel® Core™ Duo, Xbox 360 (triple cores, each with SMTs), AMD Quad-core Opteron. • IBM Cell processor with as many as 9 cores used in Sony PlayStation 3, Toshiba HD sets, and IBM Roadrunner HPC. ➢ Symmetrical Multiprocessor (SMP) a.k.a, shared memory multiprocessor • e.g. Intel® Pentium Pro Quad, motherboard with multiple sockets ➢ Cluster-based supercomputer • IBM Bluegene/L (65,536 modified PowerPC 400, each with two cores) • IBM Roadrunner (6,562 dual-core AMD Opteron® chips and 12,240 Cell chips) 6 Course Coverage • Parallel architectures Q: which are the dominant architectures? A: small-scale shared memory (SMPs), large-scale distributed memory • Programming model Q: how to program these architectures? A: Message passing and shared memory models • Programming for performance Q: how are programming models mapped to the underlying architecture, and how can this mapping be exploited for performance? 7 Course Administration • Course prerequisites • Course textbooks • Class attendance • Required work and grading policy • Late policy • Academic honesty (see details on the syllabus) 8 Outline ▪ Introduction ➢Why is parallel computing? ➢Why you should care? ▪ Course administration ➢Course coverage ➢Workload and grading ▪ Inevitability of parallel computing ➢Application demands ➢Technology and architecture trends ➢Economics ▪ Convergence of parallel architecture ➢Shared address space, message passing data parallel, data flow, systolic ➢A generic parallel architecture 9 Inevitability of Parallel Computing • Application demands: ➢ Our insatiable need for computing cycles in challenge applications • Technology Trends ➢Number of transistors on chip growing rapidly ➢Clock rates expected to go up only slowly • Architecture Trends ➢Instruction-level parallelism valuable but limited ➢Coarser-level parallelism, as in MPs, the most viable approach • Economics ➢Low incremental cost of supporting parallelism 10 Application Demands: Scientific Computing • Large parallel machines are a mainstay in many industries ➢Petroleum • Reservoir analysis ➢Automotive • Crash simulation, combustion efficiency ➢Aeronautics • Airflow analysis, structural mechanics, electromegnetism ➢Computer-aided design ➢Pharmaceuticals • Molecular modeling ➢Visualization • Entertainment • Architecture 2,300 CPU years (2.8 GHz ➢Financial modeling Intel Xeon) at a rate of approximately one hour per • Yield and derivative analysis frame. 11 Simulation: The Third Pillar of Science Traditional scientific and engineering paradigm: 1) Do theory or paper design. 2) Perform experiments or build system. Limitations: – Too difficult -- build large wind tunnels. – Too expensive -- build a throw-away passenger jet. – Too slow -- wait for climate or galactic evolution. – Too dangerous -- weapons, drug design, climate experimentation. Computational science paradigm: 3) Use high performance computer systems to simulate the phenomenon – Based on known physical laws and efficient numerical methods. 12 Challenge Computation Examples Science • Global climate modeling • Astrophysical modeling • Biology: genomics; protein folding; drug design • Computational chemistry • Computational material sciences and nanosciences Engineering • Crash simulation • Semiconductor design • Earthquake and structural modeling • Computation fluid dynamics (airplane design) Business • Financial and economic modeling Defense • Nuclear weapons -- test by simulation • Cryptography 13 Units of Measure in HPC High Performance Computing (HPC) units are: • Flop/s: floating point operations • Bytes: size of data Typical sizes are millions, billions, trillions… Mega Mflop/s = 106 flop/sec Mbyte = 106 byte (also 220 = 1048576) Giga Gflop/s = 109 flop/sec Gbyte = 109 byte (also 230 = 1073741824) Tera Tflop/s = 1012 flop/sec Tbyte = 1012 byte (also 240 = 10995211627776) Peta Pflop/s = 1015 flop/sec Pbyte = 1015 byte (also 250 = 1125899906842624) Exa Eflop/s = 1018 flop/sec Exa = 1018 byte 14 Global Climate Modeling Problem Problem is to compute: f(latitude, longitude, elevation, time) → temperature, pressure, humidity, wind velocity Approach: • Discretize the domain, e.g., a measurement point every 1km • Devise an algorithm to predict weather at time t+1 given t Source: http://www.epm.ornl.gov/chammp/chammp.html 15 Example: Numerical Climate Modeling at NASA • Weather forecasting over US landmass: 3000 x 3000 x 11 miles • Assuming 0.1 mile cubic element ---> 1011 cells • Assuming 2 day prediction @ 30 min ---> 100 steps in time scale • Computation: Partial differential equation and finite element approach • Single element computation takes 100 Flops • Total number of flops: 1011 x 100 x 100 = 1015 (i.e., one peta-flops) • Supposed uniprocessor power: 109 flops/sec (Giga-flops) • It takes 106 seconds or 280 hours. (Forecast nine days late!) • 1000 processors at 10% efficiency → around 3 hours • IBM Roadrunner → 1 second ?! • State of the art models require integration of atmosphere, ocean, sea- ice, land models, and more; Models demanding more computation resources will be applied. 16 High Resolution Climate Modeling on NERSC-3 – P. Duffy, et al., LLNL Commercial Computing • Parallelism benefits many applications ➢ Database and Web servers for online transaction processing ➢ Decision support ➢ Data mining and data warehousing ➢ Financial modeling • Scale not necessaily as large, but more widely used • Computational power determines scale of business that can be handled. 18 Outline ▪ Introduction ➢Why is parallel computing? ➢Why you should care? ▪ Course administration ➢Course coverage ➢Workload and grading ▪ Inevitability of parallel computing ➢Application demands ➢Technology and architecture trends ➢Economics ▪ Convergence of parallel architecture ➢Shared address space, message passing data parallel, data flow, systolic ➢A generic parallel architecture 19 Tunnel Vision by Experts “I think there is a world market for maybe five computers.” – Thomas Watson, chairman of IBM, 1943. “There is no reason for any individual to have a computer in their home” – Ken Olson, president and founder of Digital Equipment Corporation, 1977. “640K [of memory] ought to be enough for anybody.” – Bill Gates, chairman of Microsoft,1981. 20 Technology Trends: -processor Capacity The number of transistors on a chip doubles every 18 months (while the costs are halved). 21 Technology Trends:Transistor Count 22 23 Technology Trends 100 Supercomputers 10 Mainframes Microprocessors Minicomputers Performance 1 0.1 1965 1970 1975 1980 1985 1990 1995 • Microprocessor exhibits astonishing progress! • Natural building block for parallel computers are also state-of-art microprocessors. 24 Architecture Trend: Role of Architecture Clock rate increases 30% per year, while the overall CPU performance increases 50% to 100% per year Where is the rest coming from? ➢Parallelism likely to contribute more to performance improvements 25 Architectural Trends Greatest trend in VLSI is an increase in the exploited parallelism • Up to 1985: bit level parallelism: 4-bit -> 8 bit -> 16-bit –
Details
-
File Typepdf
-
Upload Time-
-
Content LanguagesEnglish
-
Upload UserAnonymous/Not logged-in
-
File Pages63 Page
-
File Size-