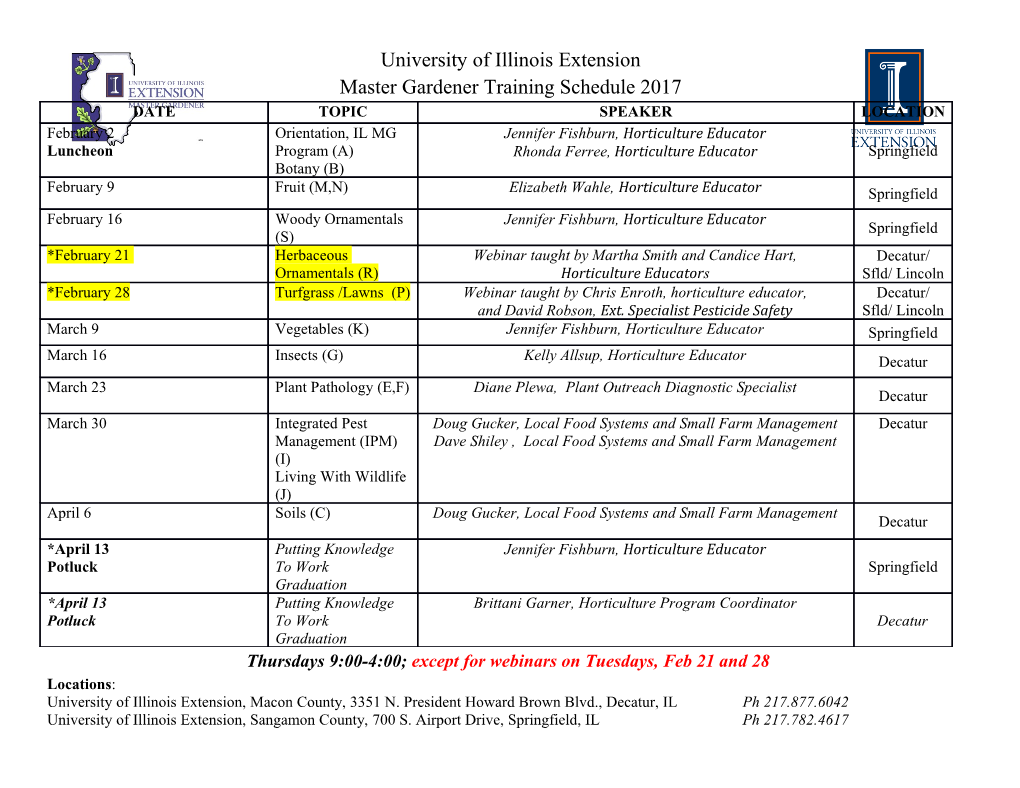
Chapter 4 The Discrete Fourier Transform Recall The Fourier Transform of a function f (t) is 1 2tsi F (t) = f (s) e ds Z1 and Fourier series 1 f (t) ~ (ak cos (2kt) + bk cos (2kt)) k=0 X The Fourier Series transform infinitely discrete signal stream an, bn to a continuous function in so-called the frequency domain. f g Section 4.1 Real-time Processing • — Consider finite data set x = x0, x1, ..., xn 1 f g — In Chap 3, we learned that a causal filter F is basically a con- volution y = h x. In many applications, the original signal x is transmitted through a channel (noise). At the other end a dis- torted signal y is received. The question is how to recover x from y if we have knowledge about the noise. In other words, we want to solve x from equation y = h x with given h. This can be achieved by applying Z transform : Z (y) = Z (h) Z (x) . So 1 y = Z (Z (h) Z (x)) . But this process is very time consuming. It is diffi cult, if not impossible, to process in real time. — Here we introduce another tool called "Discrete Fourier Trans- form" DFT that can be done in real time — DFT of a finite signal stream x is a sequence of the frequency- domain objects 2 i x^ = F (x) = x^0, x^1, ..., x^n 1 , = e n (n-th complex root of 1) f g n 1 n 1 2jk jk i x^k = xj = xje n j=0 j=0 X X n 1 2jk 2jk = x cos i sin j n n j=0 X 1 — Recall that matrix multiplication: for A = (aij)m n ,B = (bij)n p , AB = (cij)m p , where n cij = aikbkj. k=1 X — Using matrix form, let F be the n n symmetric matrix (i 1)(j 1) F = [fij]ij , fij = 1 1 1 1 y^1 x0 1 2 ··· (n 1) 1 y^2 x1 2 2 4 ··· 2(n 1)3 2 3 2 3 1 y^ x F = ··· , y^ = 3 , x = 2 6. .. 7 6 . 7 6 . 7 6. 7 6 . 7 6 . 7 6 (n 1) 2(n 1) (n 1)2 7 6 7 6 7 61 7 6y^n7 6xn 17 6 ··· 7 6 7 6 7 and consider4 the matrix equation 5 4 5 4 5 y^ = F y — One can verify that, for k = 0, 1, ..., n 1, (note that the k-th component of vector x is (x)k = xk 1 n n n 1 k(l 1) l 1=j kj y^k+1 = fk+1,lyl = yl = xj =x ^k l=1 l=1 j=0 X X X — The DFT may be written in matrix form x^ = F x x^0 1 1 1 1 x0 1 2 ··· (n 1) x^1 1 x1 2 3 2 2 4 ··· 2(n 1)3 2 3 x^ 1 x 2 = ··· 2 6 . 7 6. .. 7 6 . 7 6 . 7 6. 7 6 . 7 6 7 6 (n 1) 2(n 1) (n 1)2 7 6 7 6x^n 17 61 7 6xn 17 6 7 6 ··· 7 6 7 4 5 4 5 4 5 — Note if = 1 6 n 1 n 1 1 na ja = (a)j = = 0 (n = 1) 1 j=0 j=0 X X So n 1 n if a = 1 ja = 0 otherwise j=0 X 2 — Now the (i, j)-entry if F F is n n (i 1)(k 1) (k 1)(j 1) F F ij = fikfkj = k=1 k=1 Xn X n if i = j = (j i)(k 1) = 0 otherwise k=1 X 1 So F F = nI , or F = F /n, n 1 1 1 1 x = Fx^ = Fx^ = x^ jk n n n j j=0 X Section 4.2 Properties of DFT • — DFT is linear bijective maps from Cn to itself — Circular convolution of two n-tuples h and h : z = h x = z0, z1, ..., zn 1 f g n 1 zk = hjxk j = h0xk + h1xk 1 + ... + hkx0 + hk+1xn 1 + ... + hn 1xk+1 j=0 X = (h0, h1, ..., hn 1) (xk, xk 1, xk 2,..., x0, xn 1, xn 2,..., xk+1) where the subscripts are calculated modulo n (i.e., x 1 = xn 1, x 2 = xn 2, ...). — One may extend x periodically to all integer m : xm = xm+kn, and extend hk by 0 for m n. With this extension, circular convolu- tion is the same as the≥ discrete convolution defined in Chapter 3. To see this, we write ~ h = h0, h1, ..., hn 1, 0, 0, ... , f g x~ = x~0, x~1.x~2, ... f g = x0, x1, ..., xn 1, x0, x1, ..., xn 1, x0, x1, ..., xn 1, ... f g x~k = xk jn ~ Let h x~ = u0, u1, u2, ... .Then f g n+k n 1 n 1 un+k = hjx~n+k j = hjx~n+k j = hjxk j = zk j=0 j=0 j=0 X X X 3 So in that sense h x = h~ x~ — Example Given x = 1, 1, 2 , y = ( 1, 3, 4) . then x y = (9, 10, 5) .One can alsof use theg discrete convolution to compute the circular convolution: x~ = 1, 1, 2, 0, 0, 0, ... , y~ = 1, 3, 4, 1, 3, 4, 1, 3, 4, ... f g f g x~ y~ = 1, 2, 5, ( 1 + 4 + 6), (3 1 + 8) , (4 + 3 2) , ... f g = 1, 2, 5, 9, 10, 5, 9, 10, 5, ... f g — Define coordinate-wise product x y = x0y0, x1y1, ..., xn 1yn 1 f g — F (x y) = F (x) F (y) (or x y x^ y^) 7! — F (x y) = F (x) F (y) /n — Example (Using circular convolution to modify xk by its surround- ings) 1. Let h = h0, h1, 0, 0, ..., 0, hn 1 .Then f g n 1 zk = hjxk j = h0xk+h1xk 1+hn 1xk (n 1) = h0xk+h1xk 1+hn 1xk+1 j=0 X Therefore, xk is modified only by itself and its two immediate neighbors, if 1 k n 2,i.e., xk is not an end points. For endpoints ≤ ≤ z0 = h0x0 + h1x 1 + hn 1x (n 1) = h0x0 + h1xn 1 + hn 1x1 zn 1 = h0xn 1 + h1x(n 1) 1 + hn 1x(n 1) (n 1) = h0xn 1 + h1xn 2 + hn 1x0 So x0 is modified by x0, x1,and xn 1 that is at the opposite side. This is called "edge effect" 2. Let h = h0, h1, h2, 0, ..., 0, hn 2, hn 1 .Then zk is modified by itself, twof immediately before and twog immediately after 4 Section 4.4 The Fast Fourier Transform (http://paulbourke.net/miscellaneous/dft/) • — In DFT, each x^k requires n multiplications. So total it requires O (n2) multiplication operations — FFT can reduce the number to O (n ln n) — The Fast Fourier Transform is an algorithm that reduces the com- puter implementation time — data set of even signal x = x0, x1, ..., x2m 1 , n = 2m f g — Let y = x0, x2, x4,..., xn 1 be all signals with even indices, and f g z = x1, x3, ..., xn 1 be odd-index signals. Set f g 2 2 i i i = e n = em , = 2 = e m (m-th complex root of unity) — Then DF T of frame length n = 2m: n 1 n 1 n 1 jk jk jk x^k = xj = xj + xj j=0 j=0,j=even j=0,j=odd X X X m 1 m 1 2lk (2l+1)k = x2l + x2l+1 l=0 l=0 mX1 m X1 lk lk k = yl + zl l=0 l=0 X k X =y ^k +z ^k , k = 0, 1, ...2m 1 — Note that for k = 1, 2, ..., m 1, y^k and z^k are DF T of frame length m, m 2m m i — For d = m+k, k = 0, 1, ..., m 1, since = = 1, = e = 1, we see ld l(m+k) lm lk lk = = = d m k k = = Therefore 5 For k = 0, 1, ..., m 1 k x^k =y ^k +z ^k , (m+k) k x^m+k =y ^k +z ^k =y ^k z^k where y^k and z^k are DF T of frame length m So FFT algorithm reduces a DFT of frame length 2m to the sum of two DFT of frame length m. Total number of multiplications required: 2 (m)2 = n2/2,half as much as DFT. If n = 2a,then apply one step of FFT, the number of multi- plications reduces to 2 n 2a 1 = 2 2 — Recall that DF T has matrix form: x^ = F x — FFT basically is a matrix factorization: Let Fn be the DFT matrix of frame length n.Then Fm 0 Fn = Bn Pn 0 Fm where 2 Im Dm 1 2 (m 1) Bn = ,Dm = diag 1, n , n , ..., n , n = e n Im Dm and Pn is a permutation matrix of 00s and 10s such that y P x = , y even index term, z odd index term n z = [x0, x2, x4, ..., x2m, x1, x3, x5,..., x2m 1] — n = 4, 6 1 0 0 0 0 0 1 0 0 0 0 0 1 0 0 0 0 0 1 0 20 0 0 0 1 03 P4 = 2 3 ,P6 = 0 1 0 0 60 1 0 0 0 07 6 7 60 0 0 17 60 0 0 1 0 07 6 7 6 7 4 5 60 0 0 0 0 17 6 7 4 5 6 — If n = 2m = 4l, then Fm 0 Fn = Bn Pn 0 Fm Fl 0 Bm Pm 0 0 Fl = Bn 2 3 Pn Fl 0 0 Bm Pm 6 0 Fl 7 6 7 4 5 Fl 0 0 0 Bm 0 0 Fl 0 0 Pm 0 = Bn 2 3 Pn 0 Bm 0 0 Fl 0 0 Pm 6 0 0 0 Fl7 6 7 — Note that 4 5 1 1 B = F = 2 2 1 1 — If n = 2a, then we may continue this kind of factorization: F2 0 0 0 B4 0 0 ··· ··· 0 F2 0 0 Bn/2 0 0 B4 0 2 ··· 3 F = B ..
Details
-
File Typepdf
-
Upload Time-
-
Content LanguagesEnglish
-
Upload UserAnonymous/Not logged-in
-
File Pages10 Page
-
File Size-