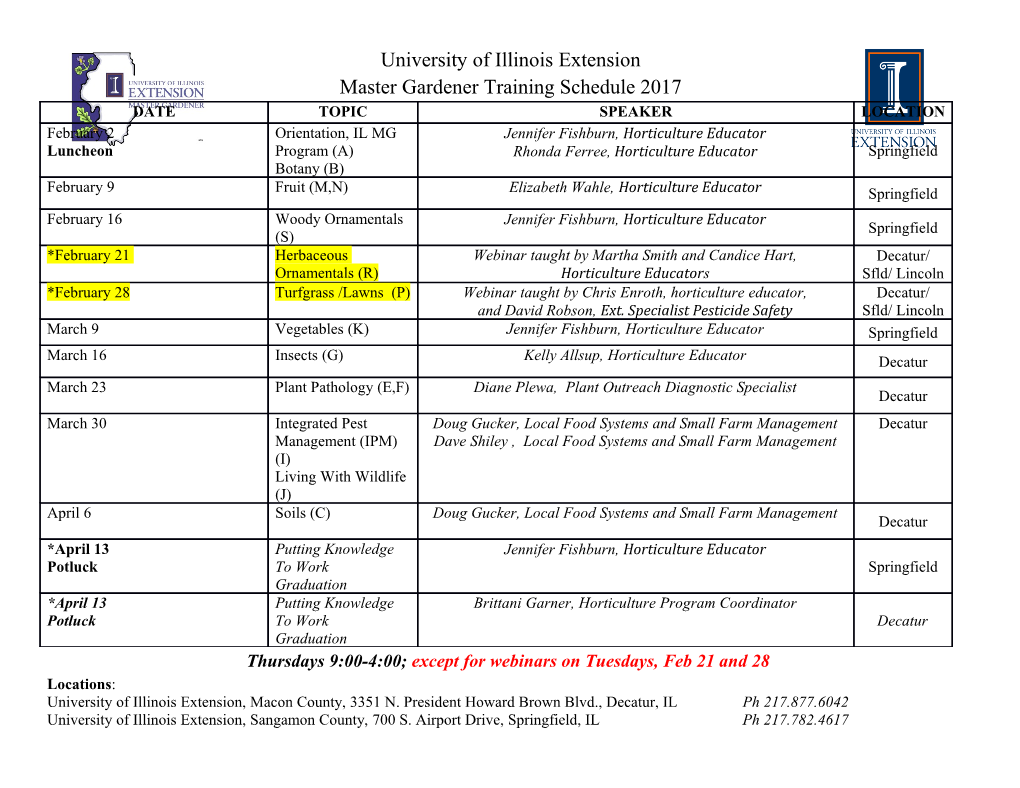
Outline Outline 1 Background and Preliminaries Random Projections for Machine Learning and 2 Johnson-Lindenstrauss Lemma (JLL) and extensions 1 Background and Preliminaries Data Mining: 3 Applications of JLL (1) Approximate Nearest Neighbour Search Theory and Applications 2 RP Perceptron Johnson-Lindenstrauss Lemma (JLL) and extensions Mixtures of Gaussians Random Features 3 Applications of JLL (1) Robert J. Durrant & Ata Kaban´ 4 Compressed Sensing 4 Compressed Sensing University of Birmingham SVM from RP sparse data 5 Applications of JLL (2) r.j.durrant, a.kaban @cs.bham.ac.uk 5 Applications of JLL (2) { } RP LLS Regression www.cs.bham.ac.uk/˜durranrj sites.google.com/site/rpforml Randomized low-rank matrix approximation Randomized approximate SVM solver 6 Beyond JLL and Compressed Sensing ECML-PKDD 2012, Friday 28th September 2012 6 Beyond JLL and Compressed Sensing Compressed FLD Ensembles of RP R.J.Durrant & A.Kaban´ (U.Birmingham) RP for Machine Learning & Data Mining ECML-PKDD 2012 1 / 123 R.J.Durrant & A.Kaban´ (U.Birmingham) RP for Machine Learning & Data Mining ECML-PKDD 2012 2 / 123 R.J.Durrant & A.Kaban´ (U.Birmingham) RP for Machine Learning & Data Mining ECML-PKDD 2012 3 / 123 Motivation - Dimensionality Curse Curse of Dimensionality Mitigating the Curse of Dimensionality The ‘curse of dimensionality’: A collection of pervasive, and often Comment: counterintuitive, issues associated with working with high-dimensional An obvious solution: Dimensionality d is too large, so reduce d to What constitutes high-dimensional depends on the problem setting, k d. data. but data vectors with arity in the thousands very common in practice Two typical problems: (e.g. medical images, gene activation arrays, text, time series, ...). How? Very high dimensional data (arity (1000)) and very many ∈ O Dozens of methods: PCA, Factor Analysis, Projection Pursuit, Random observations (N (1000)): Computational (time and space Issues can start to show up when data arity in the tens! ∈ O Projection ... complexity) issues. Very high dimensional data (arity (1000)) and hardly any We will simply say that the observations, , are d-dimensional and We will be focusing on Random Projection, motivated (at first) by the ∈ O d N T observations (N (10)): Inference a hard problem. Bogus there are N of them: = xi R i=1 and we will assume that, for ∈ O T { ∈ } following important result: interactions between features. whatever reason, d is too large. R.J.Durrant & A.Kaban´ (U.Birmingham) RP for Machine Learning & Data Mining ECML-PKDD 2012 4 / 123 R.J.Durrant & A.Kaban´ (U.Birmingham) RP for Machine Learning & Data Mining ECML-PKDD 2012 5 / 123 R.J.Durrant & A.Kaban´ (U.Birmingham) RP for Machine Learning & Data Mining ECML-PKDD 2012 6 / 123 Outline Johnson-Lindenstrauss Lemma Intuition The JLL is the following rather surprising fact [DG02, Ach03]: Theorem (Johnson and Lindenstrauss, 1984) Geometry of data gets perturbed by random projection, but not too 1 Background and Preliminaries much: 2 Let (0, 1). Let N, k N such that k C− log N, for a large ∈ ∈ > 2 Johnson-Lindenstrauss Lemma (JLL) and extensions enough absolute constant C. Let V Rd be a set of N points. Then ⊆ there exists a linear mapping R : Rd Rk , such that for all u, v V: → ∈ 3 Applications of JLL (1) (1 ) u v 2 Ru Rv 2 (1 + ) u v 2 − k − k 6 k − k 6 k − k 4 Compressed Sensing Dot products are also approximately preserved by R since if JLL 5 Applications of JLL (2) holds then: uT v (Ru)T Rv uT v + . (Proof: parallelogram − 6 6 law - see appendix). 6 Beyond JLL and Compressed Sensing Scale of k is essentially sharp: N, V s.t. ∀ ∃ k Ω( 2 log N/ log 1) is required [Alo03]. ∈ − − Figure: Original data Figure: RP data (schematic) We shall prove shortly that with high probability random projection implements a suitable linear R. R.J.Durrant & A.Kaban´ (U.Birmingham) RP for Machine Learning & Data Mining ECML-PKDD 2012 7 / 123 R.J.Durrant & A.Kaban´ (U.Birmingham) RP for Machine Learning & Data Mining ECML-PKDD 2012 8 / 123 R.J.Durrant & A.Kaban´ (U.Birmingham) RP for Machine Learning & Data Mining ECML-PKDD 2012 9 / 123 Intuition Applications What is Random Projection? (1) Geometry of data gets perturbed by random projection, but not too much: Random projections have been used for: Classification. e.g. Canonical RP: [BM01, FM03, GBN05, SR09, CJS09, RR08, DK12b] Construct a (wide, flat) matrix R k d by picking the entries ∈ M × Regression. e.g. [MM09, HWB07, BD09] from a univariate Gaussian (0, σ2). N T 1/2 Clustering and Density estimation. e.g. Orthonormalize the rows of R, e.g. set R0 = (RR )− R. [IM98, AC06, FB03, Das99, KMV12, AV09] To project a point v Rd , pre-multiply the vector v with RP matrix ∈ d k Other related applications: structure-adaptive kd-trees [DF08], R0. Then v R0v R0(R ) R is the projection of the 7→ ∈ ≡ low-rank matrix approximation [Rec11, Sar06], sparse signal d-dimensional data into a random k-dimensional projection space. reconstruction (compressed sensing) [Don06, CT06], data stream computations [AMS96]. Figure: Original data Figure: RP data & Original data R.J.Durrant & A.Kaban´ (U.Birmingham) RP for Machine Learning & Data Mining ECML-PKDD 2012 10 / 123 R.J.Durrant & A.Kaban´ (U.Birmingham) RP for Machine Learning & Data Mining ECML-PKDD 2012 11 / 123 R.J.Durrant & A.Kaban´ (U.Birmingham) RP for Machine Learning & Data Mining ECML-PKDD 2012 12 / 123 Comment (1) Concentration in norms of rows of R Near-orthogonality of rows of R If d is very large we can drop the orthonormalization in practice - the rows of R will be nearly orthogonal to each other and all nearly the same length. For example, for Gaussian ( (0, σ2)) R we have [DK12a]: N Pr (1 )dσ2 R 2 (1 + )dσ2 1 δ, (0, 1] − 6 k i k2 6 > − ∀ ∈ n o where Ri denotes the i-th row of R and δ = exp( (√1 + 1)2d/2) + exp( (√1 1)2d/2). − − − − − Similarly [Led01]: Figure: d = 100 norm Figure: d = 500 norm Figure: d = 1000 norm concentration concentration concentration Pr RT R /dσ2 1 2 exp( 2d/2), i = j. {| i j | 6 } > − − ∀ 6 Figure: Normalized dot product is concentrated about zero, d 100, 200,..., 2500 ∈ { } R.J.Durrant & A.Kaban´ (U.Birmingham) RP for Machine Learning & Data Mining ECML-PKDD 2012 13 / 123 R.J.Durrant & A.Kaban´ (U.Birmingham) RP for Machine Learning & Data Mining ECML-PKDD 2012 14 / 123 R.J.Durrant & A.Kaban´ (U.Birmingham) RP for Machine Learning & Data Mining ECML-PKDD 2012 15 / 123 Why Random Projection? Jargon Proof of JLL (1) We will prove the following randomized version of the JLL, and then show that this implies the original theorem: Linear. ‘With high probability’ (w.h.p) means with a probability as close to 1 as Theorem 2 1 we choose to make it. Let (0, 1). Let k N such that k C− log δ− , for a large enough Cheap. ∈ ∈ > absolute constant C. Then there is a random linear mapping Universal – JLL holds w.h.p for any fixed finite point set. ‘Almost surely’ (a.s.) or ‘with probability 1’ (w.p. 1) means so likely we P : Rd Rk , such that for any unit vector x Rd : → ∈ Oblivious to data distribution. can pretend it always happens. Target dimension doesn’t depend on data dimensionality (for JLL). Pr (1 ) Px 2 (1 + ) 1 δ − 6 k k 6 > − Interpretable - approximates an isometry (when d is large). ‘With probability 0’ (w.p. 0) means so unlikely we can pretend it never n o happens. Tractable to analysis. No loss to take x = 1, since P is linear. k k Note that this mapping is universal and the projected dimension k depends only on and δ. Lower bound [JW11, KMN11] k Ω( 2 log δ 1) ∈ − − R.J.Durrant & A.Kaban´ (U.Birmingham) RP for Machine Learning & Data Mining ECML-PKDD 2012 16 / 123 R.J.Durrant & A.Kaban´ (U.Birmingham) RP for Machine Learning & Data Mining ECML-PKDD 2012 17 / 123 R.J.Durrant & A.Kaban´ (U.Birmingham) RP for Machine Learning & Data Mining ECML-PKDD 2012 18 / 123 Proof of JLL (2) Proof of JLL (3) Proof of JLL (4) Recall that if W (µ , σ2) and the W are independent, then Consider the following simple mapping: i ∼ N i i i W µ , σ2 . Hence, in our setting, we have: i i ∼ N i i i i Pr Z > 1 + = Pr exp(tkZ) > exp(tk(1 + )) , t > 0 1 { } { } ∀ Px := Rx P P P Markov ineq. 6 E [exp(tkZ )] / exp(tk(1 + )), √k d d d d Y = R x E[R x ], Var(R x ) 0, x2 k i ij j ∼ N ij j ij j ≡ N j 2 i.i.d j=1 j=1 j=1 j=1 Yi indep. = E exp(tYi ) / exp(tk(1 + )), where R k d with entries Rij (0, 1). X X X X ∈ M × ∼ N Yi=1 h i Let x Rd be an arbitrary unit vector. 2 d 2 k ∈ and since x = j=1 xj = 1 we therefore have: mgf of χ2 = exp(t)√1 2t − exp( kt), t < 1/2 We are interested in quantifying: k k − − ∀ P h i Yi (0, 1) , i 1, 2,..., k next slide exp kt2/(1 2t) kt , 2 2 k ∼ N ∀ ∈ { } 6 − − 2 1 1 1 2 Px = Rx := (Y , Y ,..., Y ) = Y =: Z 2k/8 k k √ √ 1 2 k k i it follows that each of the Yi are standard normal RVs and therefore 6 e− , taking t = /4 < 1/2.
Details
-
File Typepdf
-
Upload Time-
-
Content LanguagesEnglish
-
Upload UserAnonymous/Not logged-in
-
File Pages21 Page
-
File Size-